Learning to Fly via Deep Model-Based Reinforcement Learning
arxiv(2020)
摘要
Learning to control robots without requiring models has been a long-term goal, promising diverse and novel applications. Yet, reinforcement learning has only achieved limited impact on real-time robot control due to its high demand of real-world interactions. In this work, by leveraging a learnt probabilistic model of drone dynamics, we achieve human-like quadrotor control through model-based reinforcement learning. No prior knowledge of the flight dynamics is assumed; instead, a sequential latent variable model, used generatively and as an online filter, is learnt from raw sensory input. The controller and value function are optimised entirely by propagating stochastic analytic gradients through generated latent trajectories. We show that "learning to fly" can be achieved with less than 30 minutes of experience with a single drone, and can be deployed solely using onboard computational resources and sensors, on a self-built drone.
更多查看译文
关键词
learning,deep,model-based
AI 理解论文
溯源树
样例
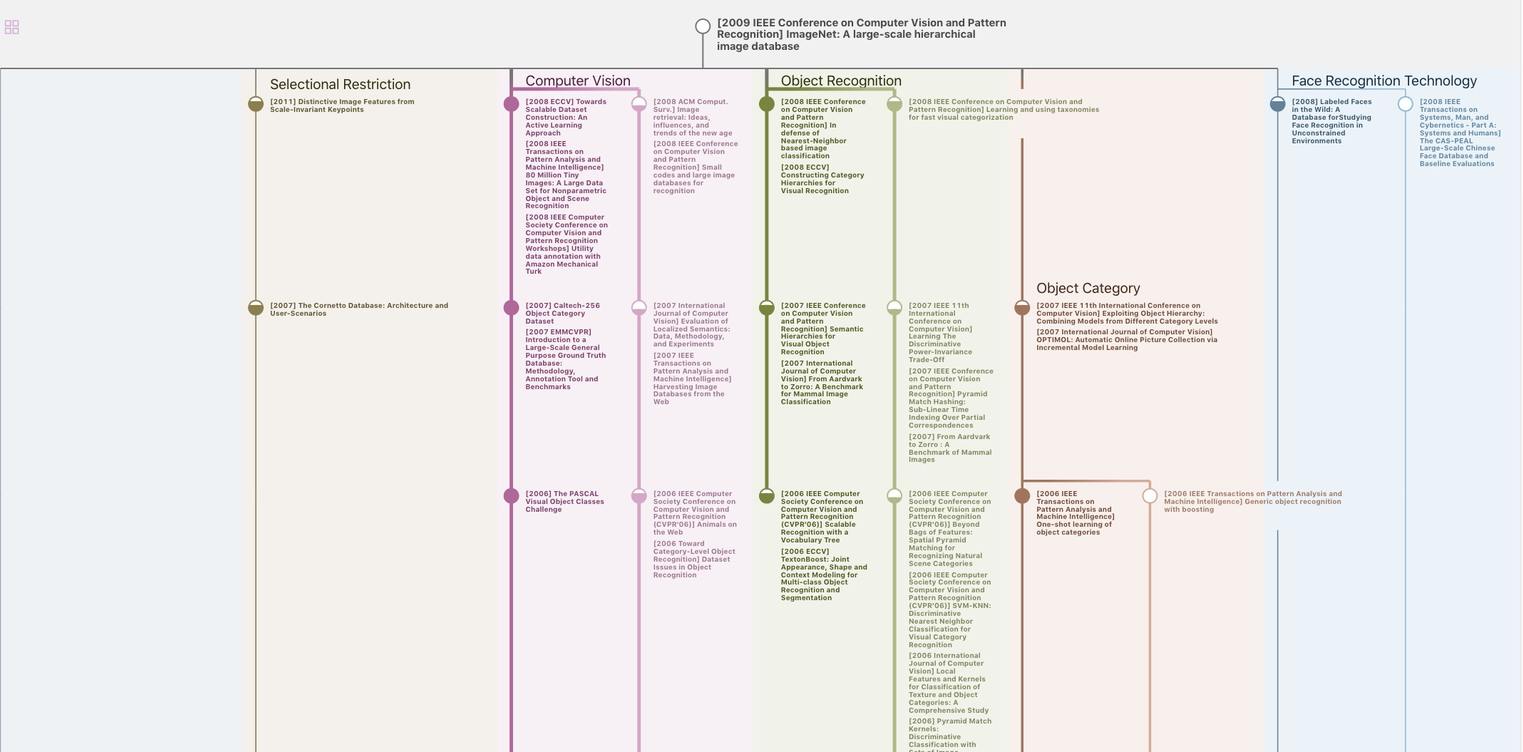
生成溯源树,研究论文发展脉络
Chat Paper
正在生成论文摘要