DRST: Deep Residual Shearlet Transform for Densely Sampled Light Field Reconstruction
arxiv(2020)
摘要
The Image-Based Rendering (IBR) approach using Shearlet Transform (ST) is one of the most effective methods for Densely-Sampled Light Field (DSLF) reconstruction. The ST-based DSLF reconstruction typically relies on an iterative thresholding algorithm for Epipolar-Plane Image (EPI) sparse regularization in shearlet domain, involving dozens of transformations between image domain and shearlet domain, which are in general time-consuming. To overcome this limitation, a novel learning-based ST approach, referred to as Deep Residual Shearlet Transform (DRST), is proposed in this paper. Specifically, for an input sparsely-sampled EPI, DRST employs a deep fully Convolutional Neural Network (CNN) to predict the residuals of the shearlet coefficients in shearlet domain in order to reconstruct a densely-sampled EPI in image domain. The DRST network is trained on synthetic Sparsely-Sampled Light Field (SSLF) data only by leveraging elaborately-designed masks. Experimental results on three challenging real-world light field evaluation datasets with varying moderate disparity ranges (8 - 16 pixels) demonstrate the superiority of the proposed learning-based DRST approach over the non-learning-based ST method for DSLF reconstruction. Moreover, DRST provides a 2.4x speedup over ST, at least.
更多查看译文
关键词
deep residual shearlet transform
AI 理解论文
溯源树
样例
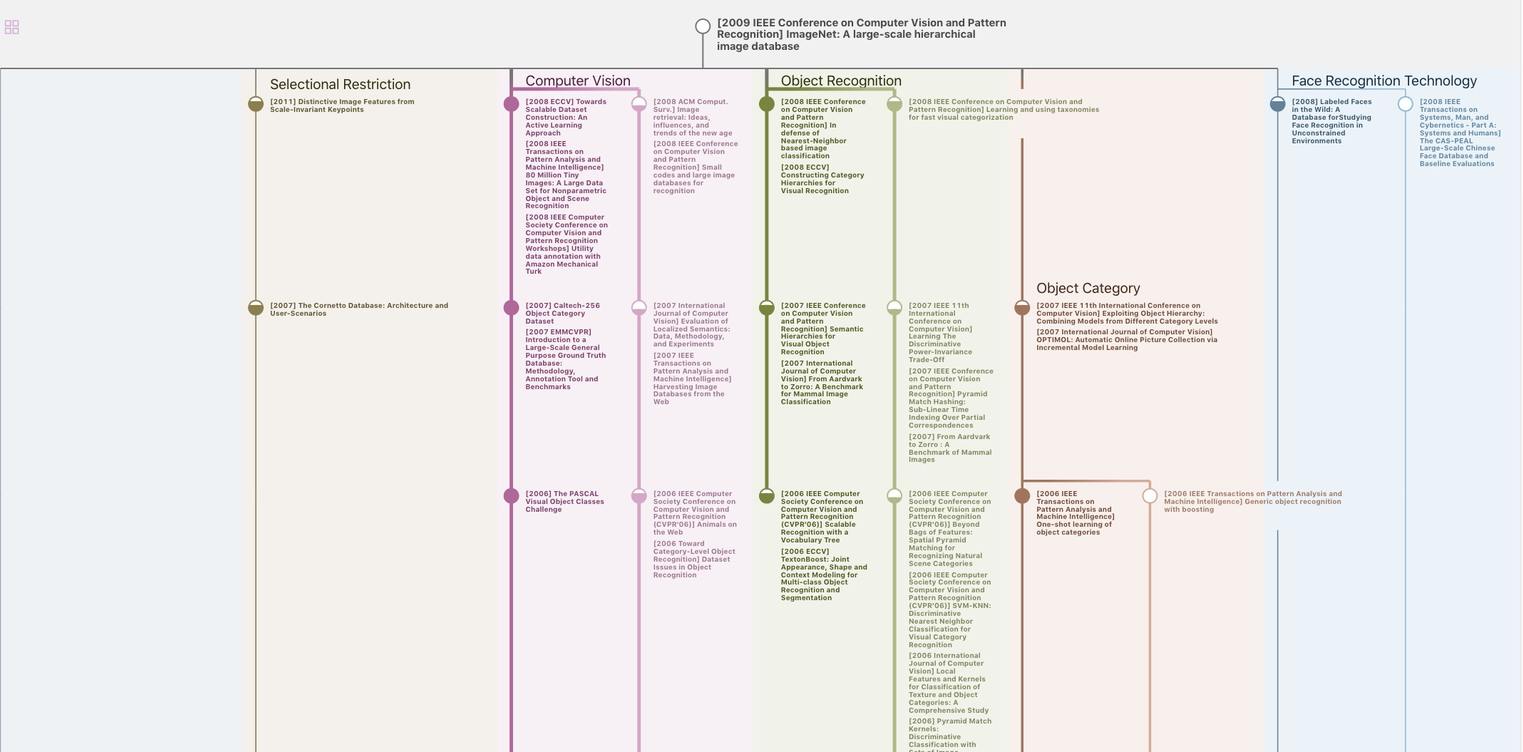
生成溯源树,研究论文发展脉络
Chat Paper
正在生成论文摘要