Stop-And-Go: Exploring Backdoor Attacks On Deep Reinforcement Learning-Based Traffic Congestion Control Systems
IEEE TRANSACTIONS ON INFORMATION FORENSICS AND SECURITY(2021)
摘要
Recent work has shown that the introduction of autonomous vehicles (AVs) in traffic could help reduce traffic jams. Deep reinforcement learning methods demonstrate good performance in complex control problems, including autonomous vehicle control, and have been used in state-of-the-art AV controllers. However, deep neural networks (DNNs) render automated driving vulnerable to machine learning-based attacks. In this work, we explore the backdooring/trojanning of DRL-based AV controllers. We develop a trigger design methodology that is based on well-established principles of traffic physics. The malicious actions include vehicle deceleration and acceleration to cause stop-and-go traffic waves to emerge (congestion attacks) or AV acceleration resulting in the AV crashing into the vehicle in front (insurance attack). We test our attack on single-lane and two-lane circuits. Our experimental results show that the backdoored model does not compromise normal operation performance, with the maximum decrease in cumulative rewards being 1%. Still, it can be maliciously activated to cause a crash or congestion when the corresponding triggers appear.
更多查看译文
关键词
Games, Training, Deep learning, Computational modeling, Reinforcement learning, Perturbation methods, Integrated circuit modeling, Autonomous vehicles, artificial neural networks, information security
AI 理解论文
溯源树
样例
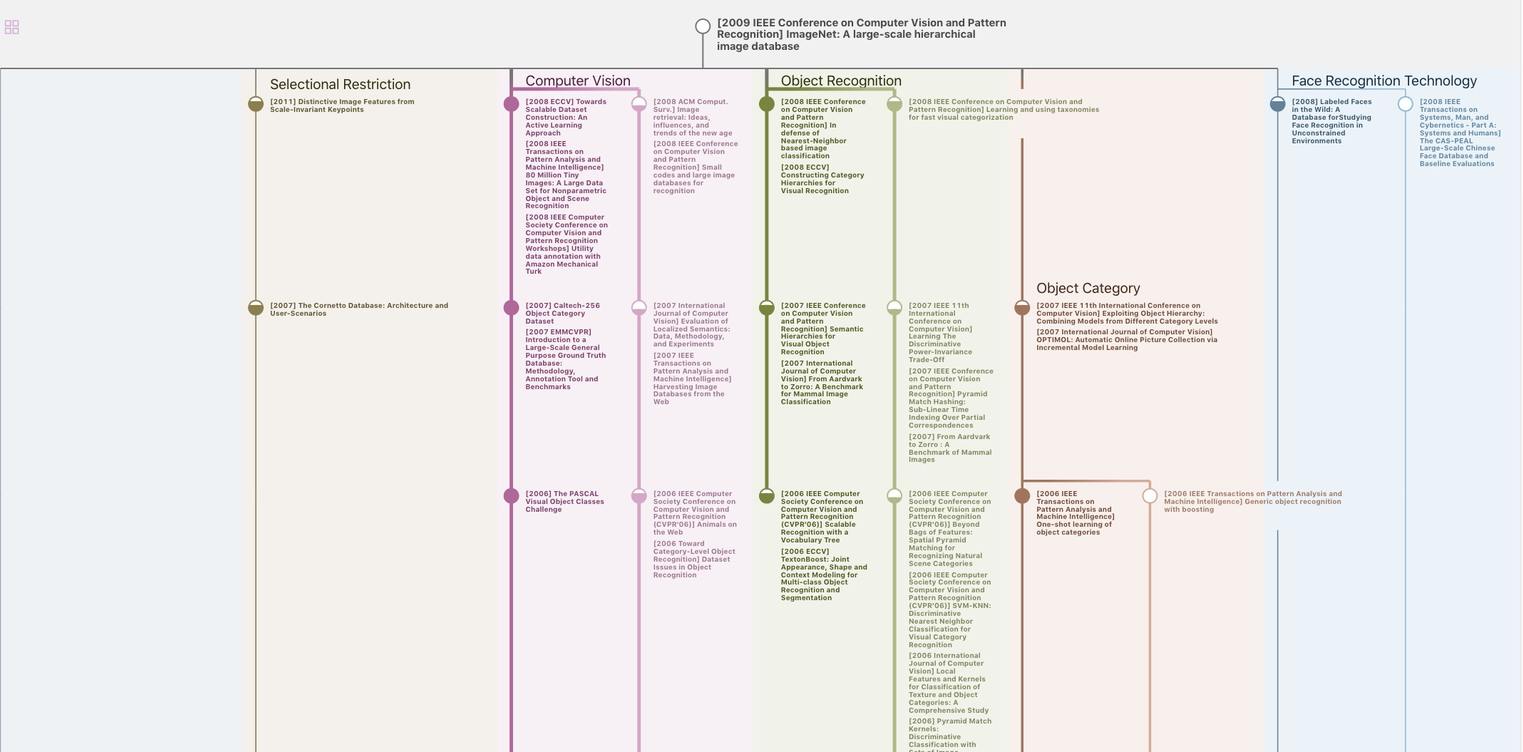
生成溯源树,研究论文发展脉络
Chat Paper
正在生成论文摘要