Research on Resolution Limit of Community Detection in Location-Based Social Networks
2019 International Conference on Networking and Network Applications (NaNA)(2019)
摘要
Location-based social network (LBSN) is a highly used social network at present. Detecting the community structures in LBSN plays a significant role in analyzing the network comprehensively. Modularity optimization is a traditional social network community detection method which has excellent performance. Whereas, resolution limit is an inherent problem of it. Some existing community detection methods for LBSN fail to take account of the full network information. To solve above problems, a network weighting scheme is proposed in this paper. Firstly, we combine a network embedding method that named walk2friends with this paper. In view of the shortcoming of walk2friends, we proposes a collaborative filtering method based on social relationship to improve it. Then, the learned similarity is obtained by improved walk2friends and the structural similarity is obtained by a random walk method. These two similarities are fused and then transformed into edge weight. Finally, the undirected unweighted graph is preprocessed as an undirected weighted graph. Experimental results on real networks show that when using modularity optimization method for optimization optimization the weighting scheme not only can avoid the resolution limit but also applicable to LBSN . The mined community is more cohesive than the existing work on LBSN.
更多查看译文
关键词
LBSN,Community Detection,Resolution Limit,Modularity Optimization
AI 理解论文
溯源树
样例
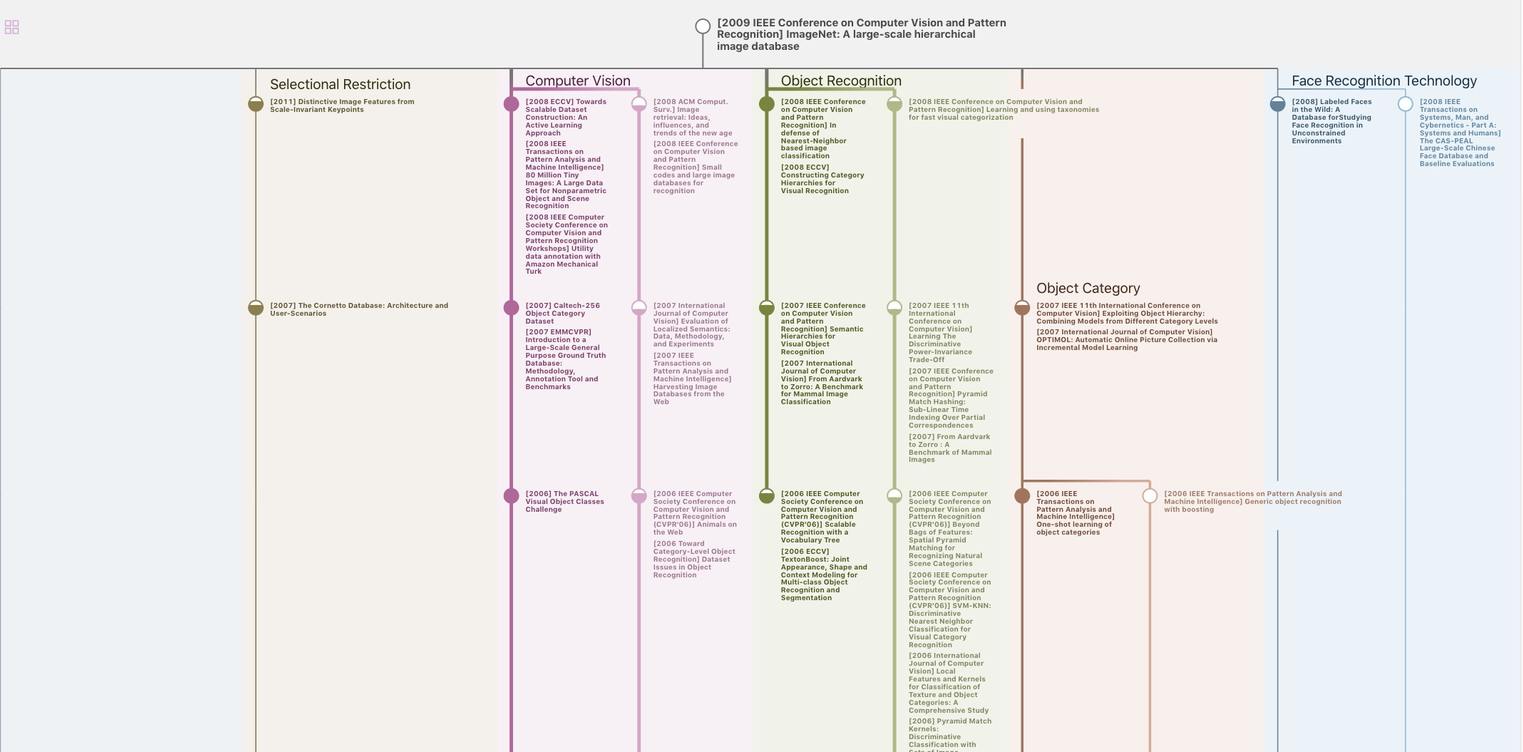
生成溯源树,研究论文发展脉络
Chat Paper
正在生成论文摘要