Enabling automated herbarium sheet image post-processing using neural network models for color reference chart detection.
APPLICATIONS IN PLANT SCIENCES(2020)
摘要
Premise Large-scale efforts to digitize herbaria have resulted in more than 18 million publicly available Plantae images on sites such as iDigBio. The automation of image post-processing will lead to time savings in the digitization of biological specimens, as well as improvements in data quality. Here, new and modified neural network methodologies were developed to automatically detect color reference charts (CRC), enabling the future automation of various post-processing tasks. Methods and Results We used 1000 herbarium specimen images from 52 herbaria to test our novel neural network model, ColorNet, which was developed to identify CRCs smaller than 4 cm(2), resulting in a 30% increase in accuracy over the performance of other state-of-the-art models such as Faster R-CNN. For larger CRCs, we propose modifications to Faster R-CNN to increase inference speed. Conclusions Our proposed neural networks detect a range of CRCs, which may enable the automation of post-processing tasks found in herbarium digitization workflows, such as image orientation or white balance correction.
更多查看译文
关键词
automation,digitization,herbarium,machine learning,natural history collections,specimen images
AI 理解论文
溯源树
样例
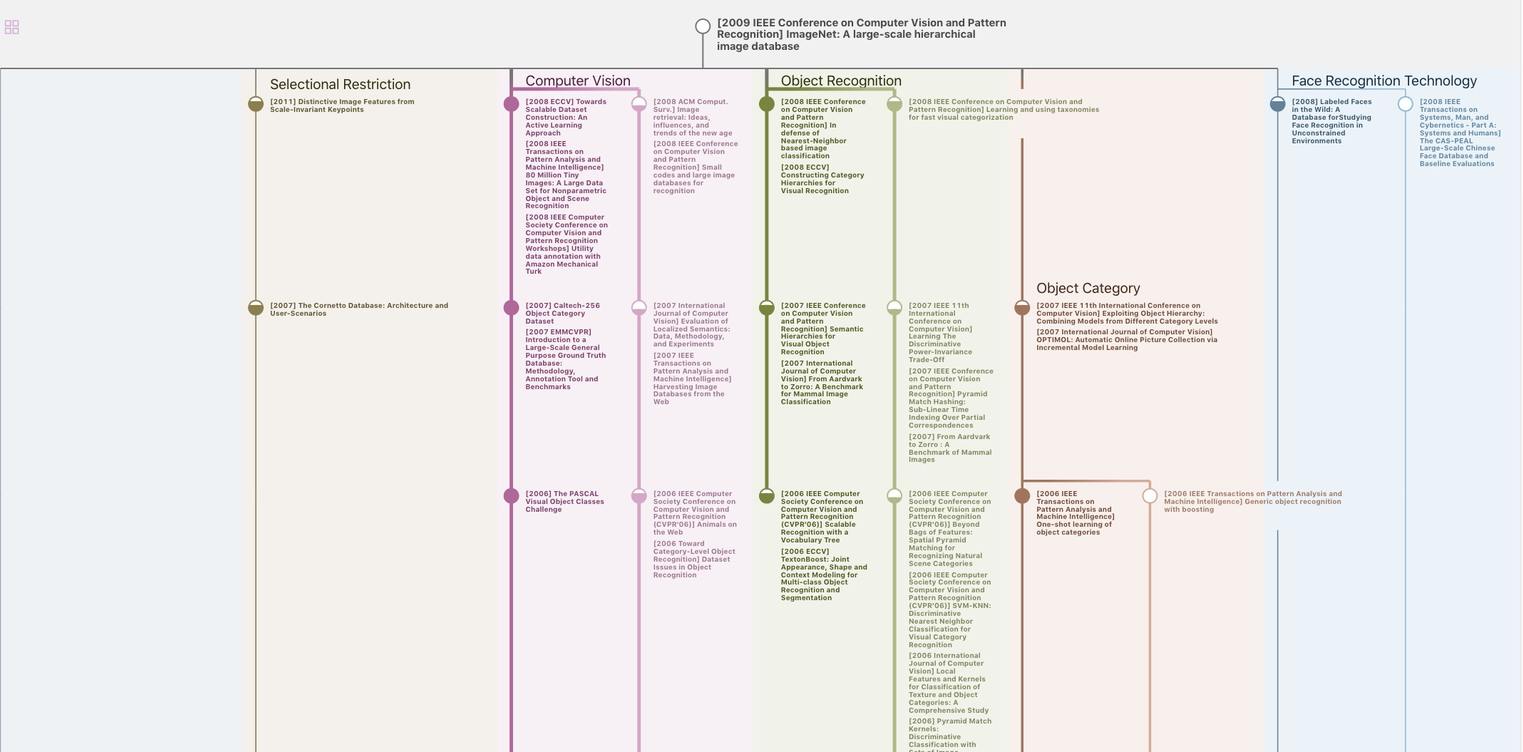
生成溯源树,研究论文发展脉络
Chat Paper
正在生成论文摘要