Supervised learning as an inverse problem based on non-smooth loss function
Knowledge and Information Systems(2020)
摘要
This paper is concerned by solving supervised machine learning problem as an inverse problem. Recently, many works have focused on defining a relationship between supervised learning and the well-known inverse problems. However, this connection between the learning problem and the inverse one has been done in the particular case where the inverse problem is reformulated as a minimization problem with a quadratic cost functional ( L^2 cost functional). Although, it is well known that the cost functional can be L^1 , L^2 or any positive function that measures the gap between the predicted data and the observed one. Indeed, the use of L^1 loss function for supervised learning problem gives more consistent results (see Rosasco et al. in Neural Comput 16:1063–1076, 2004). This strengthens the idea of reformulating the inverse problem, associated to machine learning problem, into a minimization problem using L^1 functional. However, the L^1 loss function is non-differentiable, which precludes the use of standard optimization tools. To overcome this difficulty, we propose in this paper a new technique of approximation based on the reformulation of the associated inverse problem into a minimizing one of a slanting cost functional Chen et al. (MIS Q Manag Inf Syst 36:1165–1188, 2012), which is solved using Tikhonov regularization and Newton’s method. This approach leads to an efficient numerical algorithm allowing us to solve supervised learning problem in the most general framework. To confirm this, we present some numerical results showing the efficiency of the proposed approach. Furthermore, the numerical experiment validation is made through academic and real-life data. Thus, the comparison with existing methods and numerical stability of the algorithm is presented in order to show that our approach is better in terms of convergence speed and quality of predicted models.
更多查看译文
关键词
Inverse problem, Supervised learning, Non-smooth loss function, Optimization, Slanting function, Airfoil self-noise, ECG signals
AI 理解论文
溯源树
样例
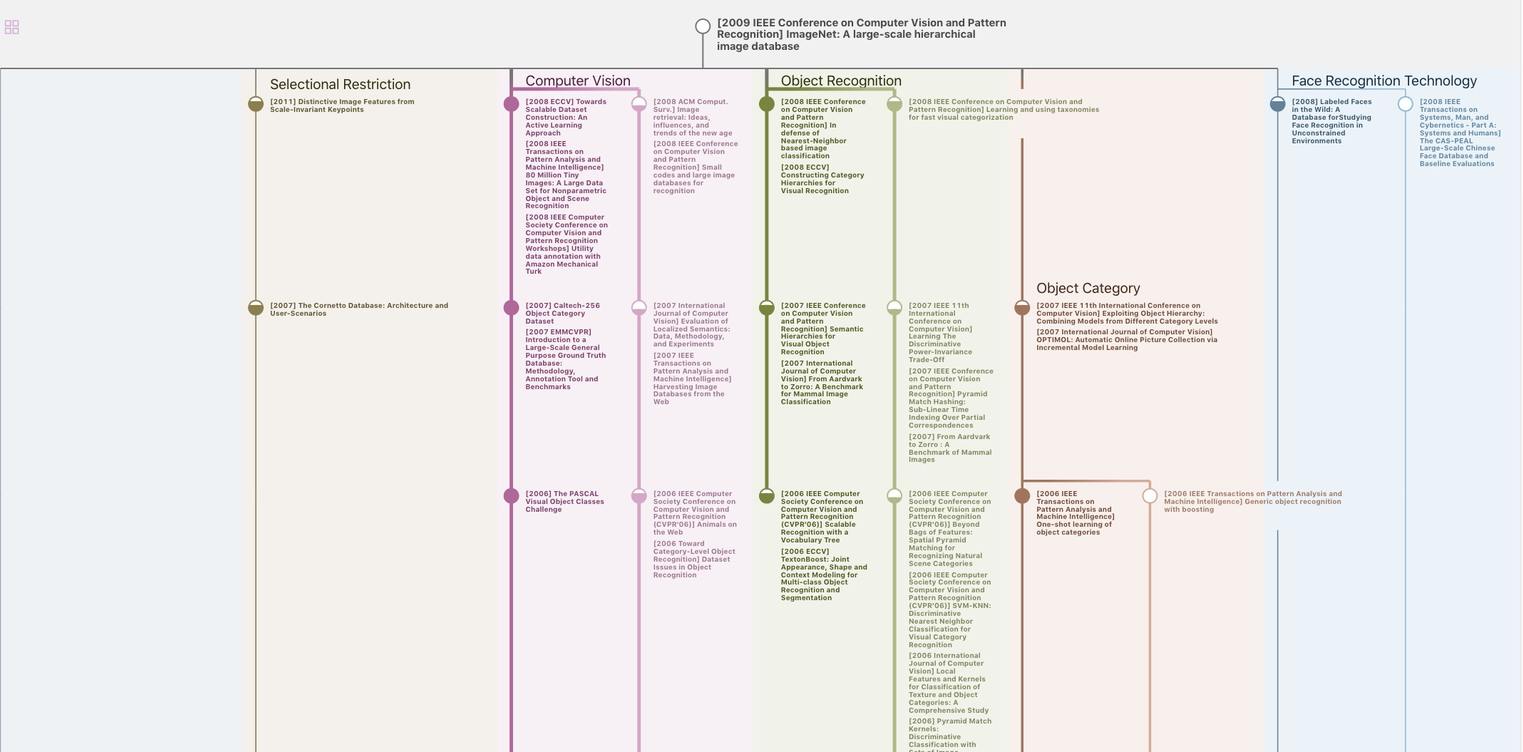
生成溯源树,研究论文发展脉络
Chat Paper
正在生成论文摘要