Using Open Access Data to Model a Technician Routing and Scheduling Problem in a Congested Urban Setting
Procedia Manufacturing(2019)
摘要
Abstract This research aims at studying and improving the attended home services delivery in the city of Bogota (Colombia) by considering varying traffic patterns along the day. The goal is to improve routing decisions by adopting appropriate travel time functions between pairs of locations that are built upon the basis of freely available information gathered from collaborative consumption platforms. In first place, data collected from Uber Movement ® platform is used to identify the 1-hour consolidated traffic patterns based on coordinates and time of the day for all pairs of neighborhoods within the city. However, not all the information is available, as there might exist some journeys scarcely required by Uber users. To overcome this problem, a K-nearest neighbor regression is used to predict missing travel times when required and considering as an input the coordinates and the time of the day. Then, a piecewise linear function representing the travel time between pairs of locations is constructed by assuming that a breakpoint, or change in the travel time behavior, takes place at the middle point of each one-hour time frame. A set of piecewise linear functions is then obtained after solving a system of linear equations. Following, two different approaches are used to generate solutions for a technician routing and scheduling problem with hard time windows. The first consists in an approximation Integer Programming model that represents the problems using an acyclic directed graph. The second consist in a Memetic Algorithm that minimizes the number of vehicles and vehicles field time. Preliminary results show that both approaches present similar results and can be used in practical applications.
更多查看译文
关键词
Last-mile logistics,Time-dependent Technician Routing,Scheduling Problem,Memetic algorithm,Mixed Integer Programming
AI 理解论文
溯源树
样例
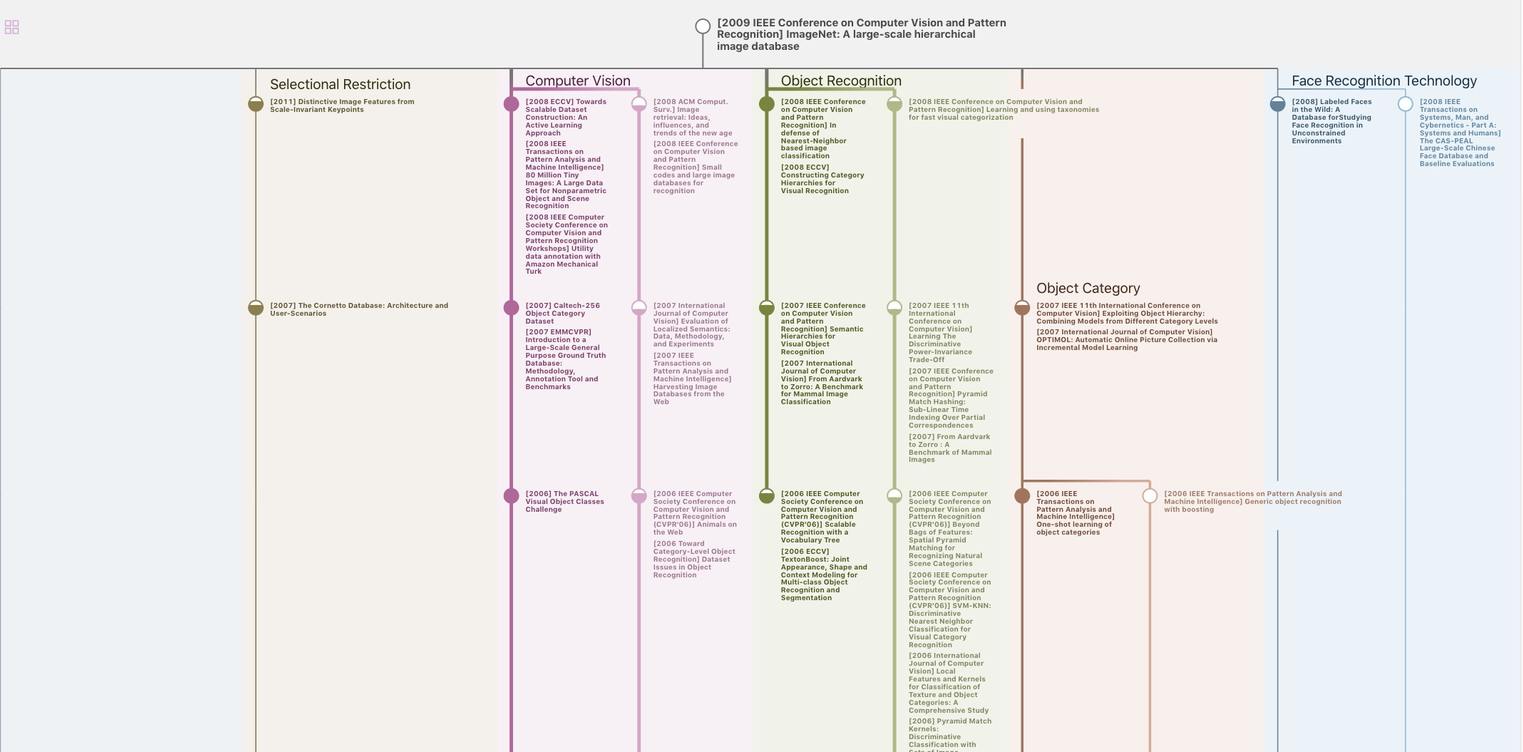
生成溯源树,研究论文发展脉络
Chat Paper
正在生成论文摘要