Deep quantification down-plain-upsampling residual learning for single image super-resolution
INTERNATIONAL JOURNAL OF MACHINE LEARNING AND CYBERNETICS(2020)
摘要
Deep convolutional neural networks have been widely used in single image super-resolution (SISR) with great success. However, the performance and efficiency of such models need to be improved for practical applications. In this paper, a novel deep quantification down-plain-upsampling (QDPU) network for SISR is proposed. In the framework, a down-plain-upsampling (DPU) residual block based on U-Net is firstly designed to reduce the computational cost by transforming the spatial scale of feature maps without sacrificing the reconstruction performance. Then, to better transmit low-level features to the reconstruction layer, we construct quantification skip-connection modules to integrate the outputs of the DPU residual blocks. Finally, QDPU is developed by stacking the DPU residual blocks with multiple skip-connections to reconstruct high-resolution images and reduce the computational burden. Quantitative and qualitative evaluations of the reconstruction results on four benchmark datasets show that the proposed method can achieve better performance compared with several state-of-the-art SISR methods.
更多查看译文
关键词
Super-resolution, Deep convolutional neural networks, U-Net, Residual learning
AI 理解论文
溯源树
样例
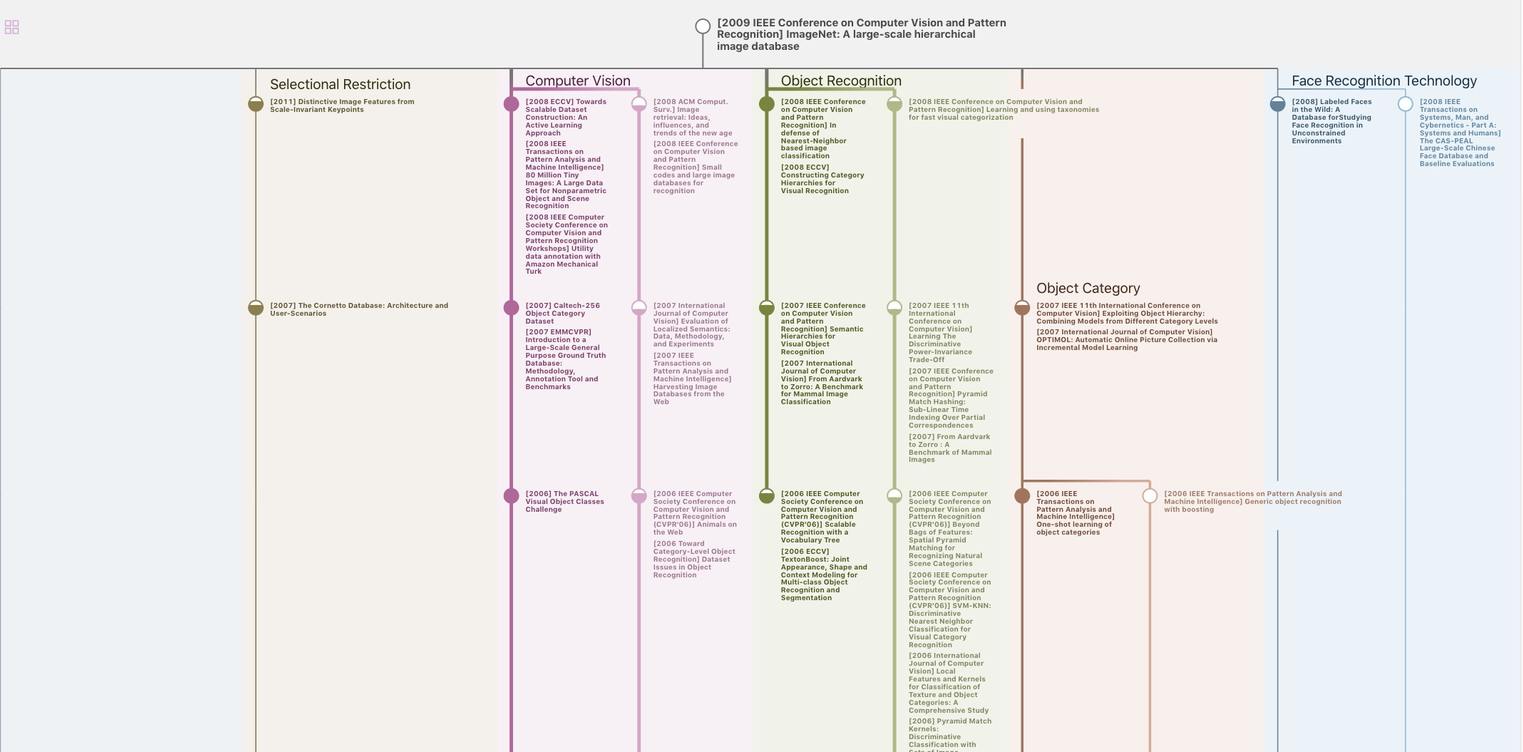
生成溯源树,研究论文发展脉络
Chat Paper
正在生成论文摘要