Artifacts Reduction for Compression Image with Pyramid Residual Convolutional Neural Network
international conference video and image processing(2019)
摘要
A pyramid residual convolutional neural network (PRCNN) is proposed to restore image with compression artifacts in this paper, and the method is enable to process multiple resolutions by fixing patch size extracted from whole image. Convolutional neural network has reached great performance in image processing (e.g. denoise, deblur, super-resolution), however deeper network may cause vanishing or exploding gradient problems, and it is hard to apply in realistic scene for high complexity. Thus, the residual blocks (RB) are proposed to balance between performance and application, besides, this paper exploits pyramid convolutional neural network to learn coarse-fine feature. In order to handle various resolutions, the fixed patch based method is used to adapt realistic scene. The experiment shows that the proposed algorithm can reduce compression artifacts through objective and subjective assessment, and the training/testing data are collected with H.264 coding. The proposed method can improve PSNR and SSIM from 0.54dB to 1.41dB, 0.01 to 0.04 while compression artifacts are reduced in visual quality, respectively.
更多查看译文
关键词
Compression artifacts, fixed patch, pyramid, residual blocks
AI 理解论文
溯源树
样例
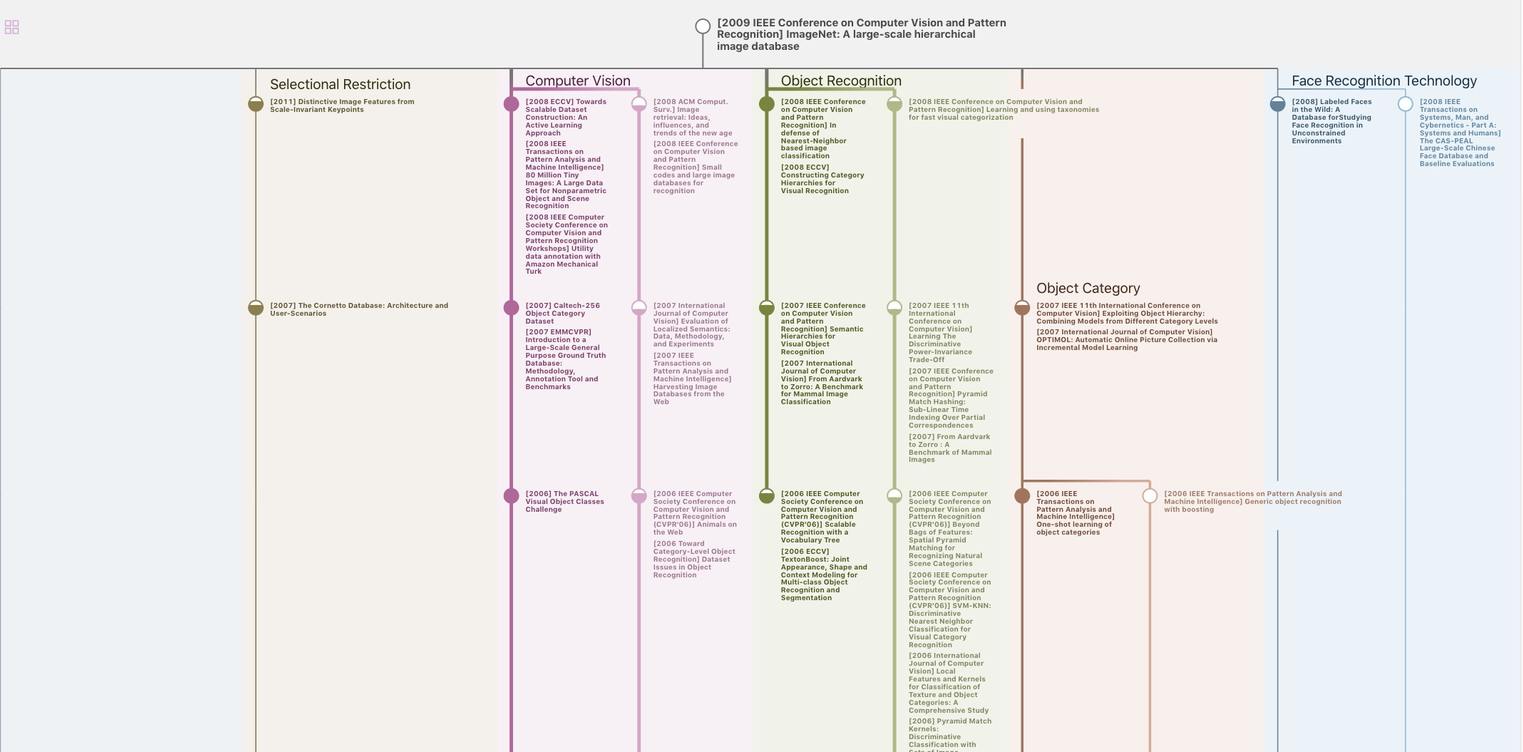
生成溯源树,研究论文发展脉络
Chat Paper
正在生成论文摘要