A Multiviewpoint Outdoor Dataset for Human Action Recognition
IEEE Transactions on Human-Machine Systems(2020)
摘要
Advancements in deep neural networks have contributed to near-perfect results for many computer vision problems, such as object recognition, face recognition, and pose estimation. However, human action recognition is still far from human-level performance. Owing to the articulated nature of the human body, it is challenging to detect an action from multiple viewpoints, particularly from an aerial viewpoint. This is further compounded by a scarcity of datasets that cover multiple viewpoints of actions. To fill this gap and enable research in wider application areas, in this article we present a multiviewpoint outdoor action recognition dataset collected from YouTube and our own drone. The dataset consists of 20 dynamic human action classes, 2324 video clips, and 503 086 frames. All videos are cropped and resized to 720 × 720 without distorting the original aspect ratio of the human subjects in videos. This dataset should be useful to many research areas, including action recognition, surveillance, and situational awareness. We evaluate the dataset with a two-stream convolutional neural network architecture coupled with a recently proposed temporal pooling scheme called kernelized rank pooling that produces nonlinear feature subspace representations. The overall baseline action recognition accuracy is 74.0%.
更多查看译文
关键词
Human action recognition,kernelized rank pooling,multiviewpoint,video dataset
AI 理解论文
溯源树
样例
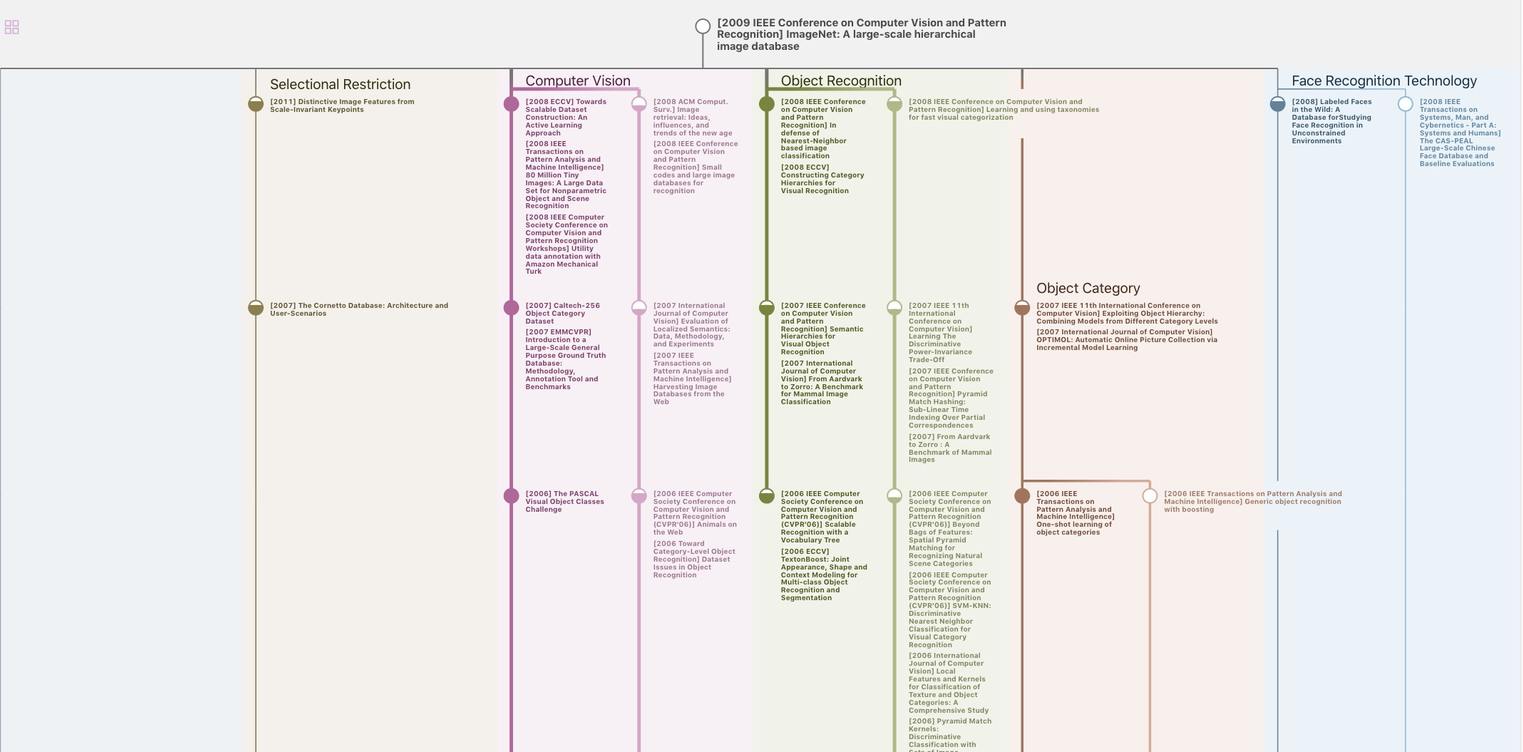
生成溯源树,研究论文发展脉络
Chat Paper
正在生成论文摘要