Detection and Localization of Short Duration Variations Using Sliding Window SVD and Sparse Signal Decomposition
IEEE Transactions on Instrumentation and Measurement(2020)
摘要
This article proposes a new approach for the detection and localization of short-duration variations using sliding window singular value decomposition (SVD) and sparse signal decomposition (SSD) on the overcomplete dictionary. In order to solve the problem of high computational complexity in SSD, the estimated frequency is obtained first by using the fast Fourier transform (FFT) to search the frequency component with the maximum energy. Next, the SVD is applied to decompose the Hankel matrices constructed by sliding window to achieve short-duration variations localization preliminarily. After that, using the estimation of each parameter as the center, a small-scale dictionary is obtained by the rediscretized parameters of the atoms in overcomplete dictionary. The method finally decomposes the signal using the proposed SSD technique. Thus, the short-duration variation events can be extracted from the signal, and the output results of SSD can provide their power quality (PQ) features to identify the event type. The proposed method is tested using both simulation and realistic signals. The experimental results show that the application of proposed sliding window SVD algorithm can greatly reduce computational complexity by decreasing the number of atoms in the overcomplete dictionary and demonstrate the accuracy and robustness of the proposed SSD technique in detection of short-duration variations under noiseless and noisy conditions.
更多查看译文
关键词
Overcomplete dictionary,short-duration variation,singular value decomposition (SVD),sliding window,sparse signal decomposition (SSD)
AI 理解论文
溯源树
样例
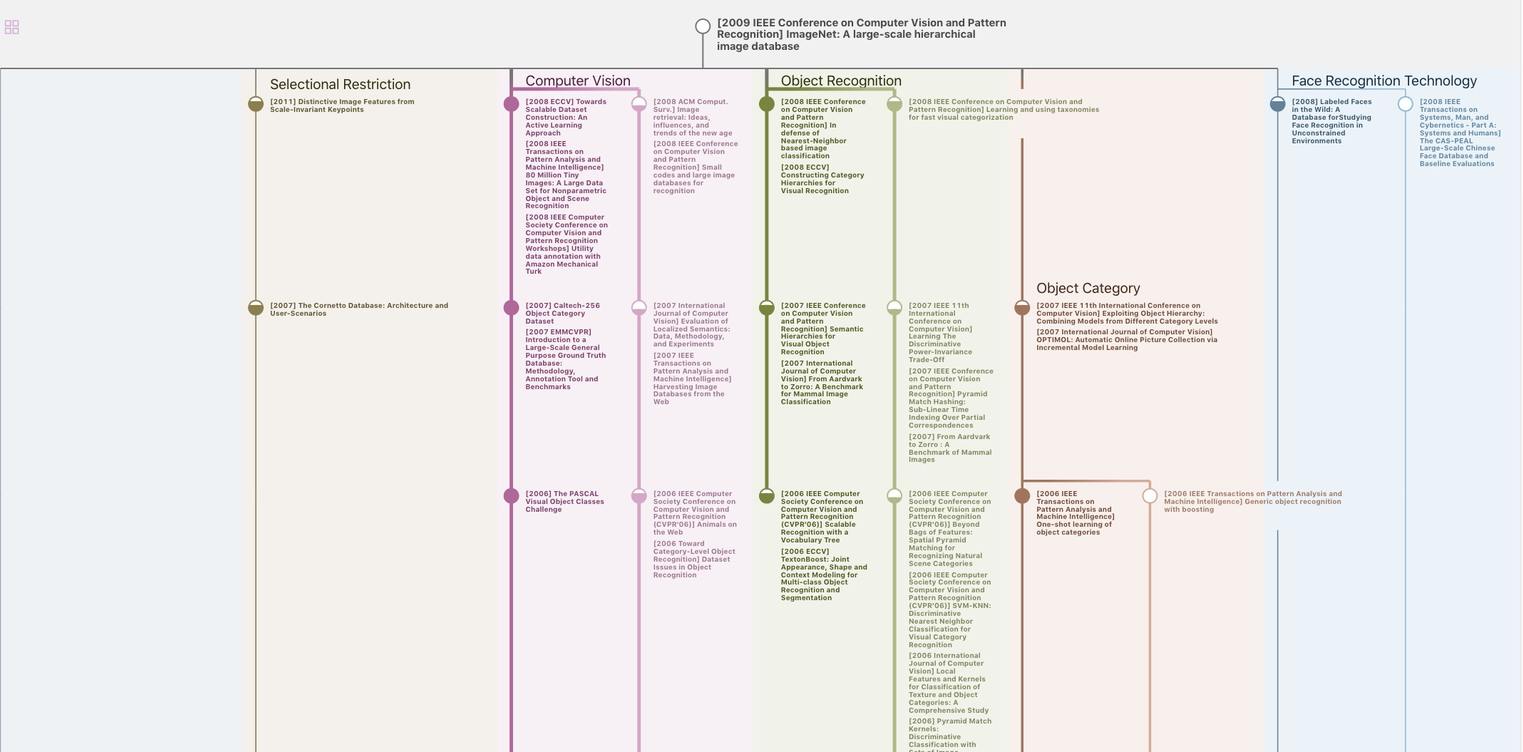
生成溯源树,研究论文发展脉络
Chat Paper
正在生成论文摘要