Graph augmented triplet architecture for fine-grained patient similarity
WORLD WIDE WEB-INTERNET AND WEB INFORMATION SYSTEMS(2020)
摘要
Electronic Health Records (EHRs) provide rich information for the research of multiple healthcare applications that improve chance of survival in Intensive Care Units (ICU), especially the case-based decision support system, which helps physicians make effective clinical decisions in the rapidly changing environment of ICUs according to similar historical patient records. Thus, an efficient approach being able to measure clinically similarities among patients is a fundamental and critical module for the decision support system. In this paper, we propose a novel framework that derives informative EHR graphs from patient records to augment information transmission in Recurrent Neural Networks (RNNs) for fine-grained patient similarity learning, named Graph Augmented Triplet Architecture (GATA). Specifically, GATA firstly derives Dynamic Bayesian Networks (DBNs) from EHRs to reveal correlations among medical variables, then it constructs graph augmented RNNs where each unit aggregate information from variables that it conditionally dependent in DBNs. After that, the specially designed RNNs will act as the fundamental components of the Triplet architecture to measure similarities among patients. GATA has been compared to different baselines based on a real-world ICU dataset MIMIC III, and the experimental results illustrate the effectiveness of GATA in fine-grained patient similarity learning, providing a promising direction for the research on clinical decision support.
更多查看译文
关键词
Patient similarity,EHRs,Graph augmentation,Triplet architecture
AI 理解论文
溯源树
样例
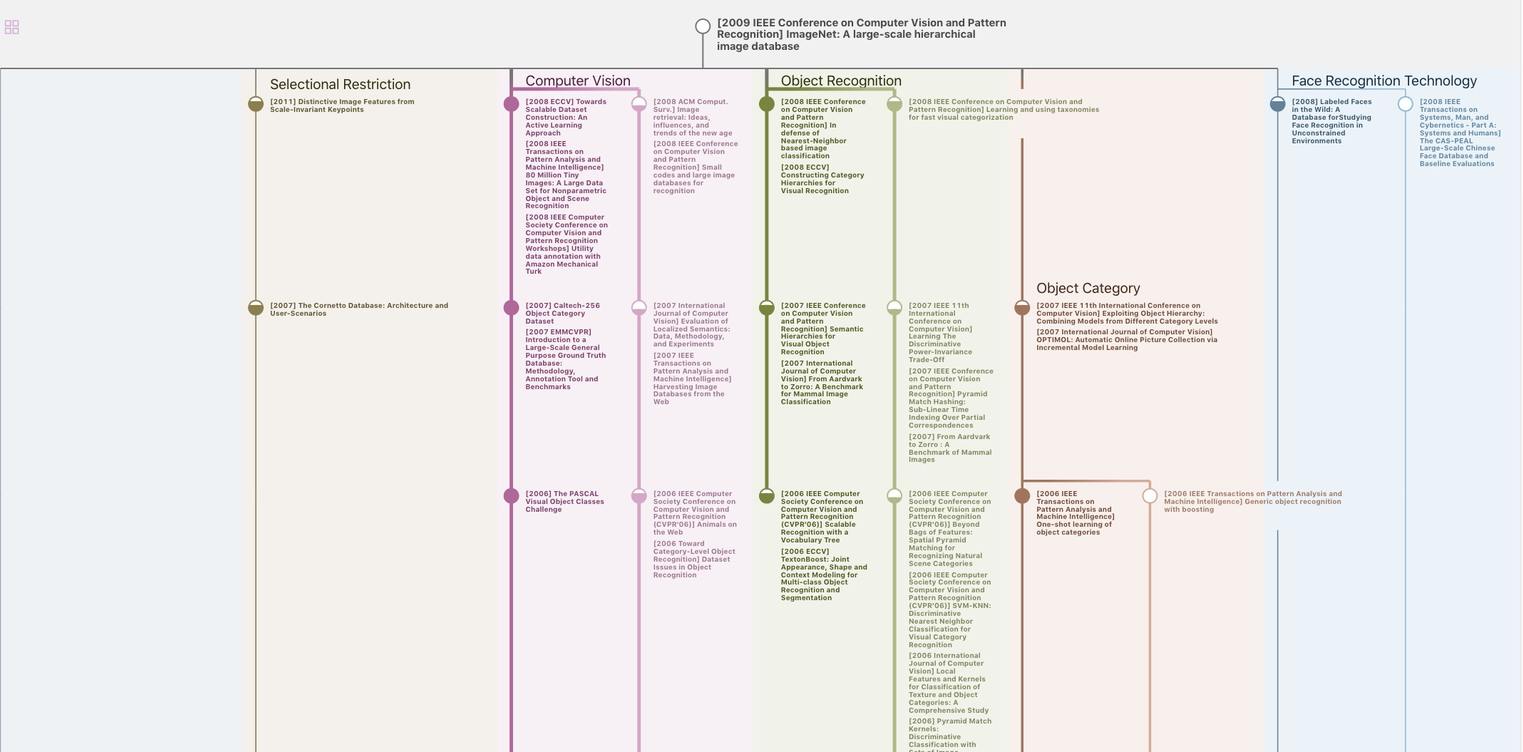
生成溯源树,研究论文发展脉络
Chat Paper
正在生成论文摘要