Characterizing and Mitigating Sensor Generated Spatial Correlations in Airborne Hyperspectral Imaging Data
REMOTE SENSING(2020)
摘要
In hyperspectral imaging (HSI), the spatial contribution to each pixel is non-uniform and extends past the traditionally square spatial boundaries designated by the pixel resolution, resulting in sensor-generated blurring effects. The spatial contribution to each pixel can be characterized by the net point spread function, which is overlooked in many airborne HSI applications. The objective of this study was to characterize and mitigate sensor blurring effects in airborne HSI data with simple tools, emphasizing the importance of point spread functions. Two algorithms were developed to (1) quantify spatial correlations and (2) use a theoretically derived point spread function to perform deconvolution. Both algorithms were used to characterize and mitigate sensor blurring effects on a simulated scene with known spectral and spatial variability. The first algorithm showed that sensor blurring modified the spatial correlation structure in the simulated scene, removing 54.0%-75.4% of the known spatial variability. Sensor blurring effects were also shown to remove 31.1%-38.9% of the known spectral variability. The second algorithm mitigated sensor-generated spatial correlations. After deconvolution, the spatial variability of the image was within 23.3% of the known value. Similarly, the deconvolved image was within 6.8% of the known spectral variability. When tested on real-world HSI data, the algorithms sharpened the imagery while characterizing the spatial correlation structure of the dataset, showing the implications of sensor blurring. This study substantiates the importance of point spread functions in the assessment and application of airborne HSI data, providing simple tools that are approachable for all end-users.
更多查看译文
关键词
hyperspectral imaging,point spread function,spatial correlations,image deconvolution
AI 理解论文
溯源树
样例
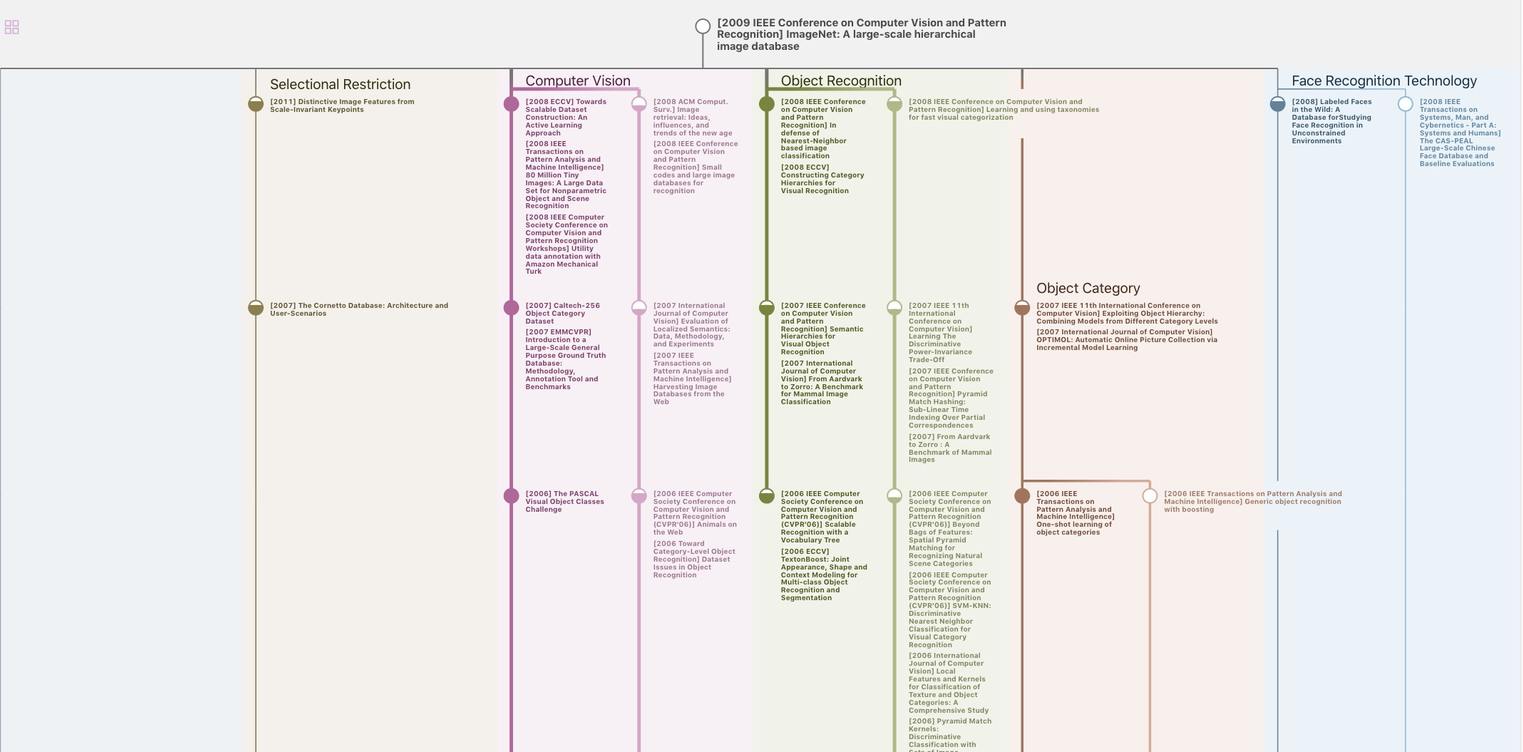
生成溯源树,研究论文发展脉络
Chat Paper
正在生成论文摘要