Efficient Selection of Hyperparameters in Large Bayesian VARs Using Automatic Differentiation
JOURNAL OF FORECASTING(2020)
摘要
Large Bayesian vector autoregressions with the natural conjugate prior are now routinely used for forecasting and structural analysis. It has been shown that selecting the prior hyperparameters in a data-driven manner can often substantially improve forecast performance. We propose a computationally efficient method to obtain the optimal hyperparameters based on automatic differentiation, which is an efficient way to compute derivatives. Using a large US data set, we show that using the optimal hyperparameter values leads to substantially better forecast performance. Moreover, the proposed method is much faster than the conventional grid-search approach, and is applicable in high-dimensional optimization problems. The new method thus provides a practical and systematic way to develop better shrinkage priors for forecasting in a data-rich environment.
更多查看译文
关键词
automatic differentiation,forecasts,marginal likelihood,optimal hyperparameters,vector autoregression
AI 理解论文
溯源树
样例
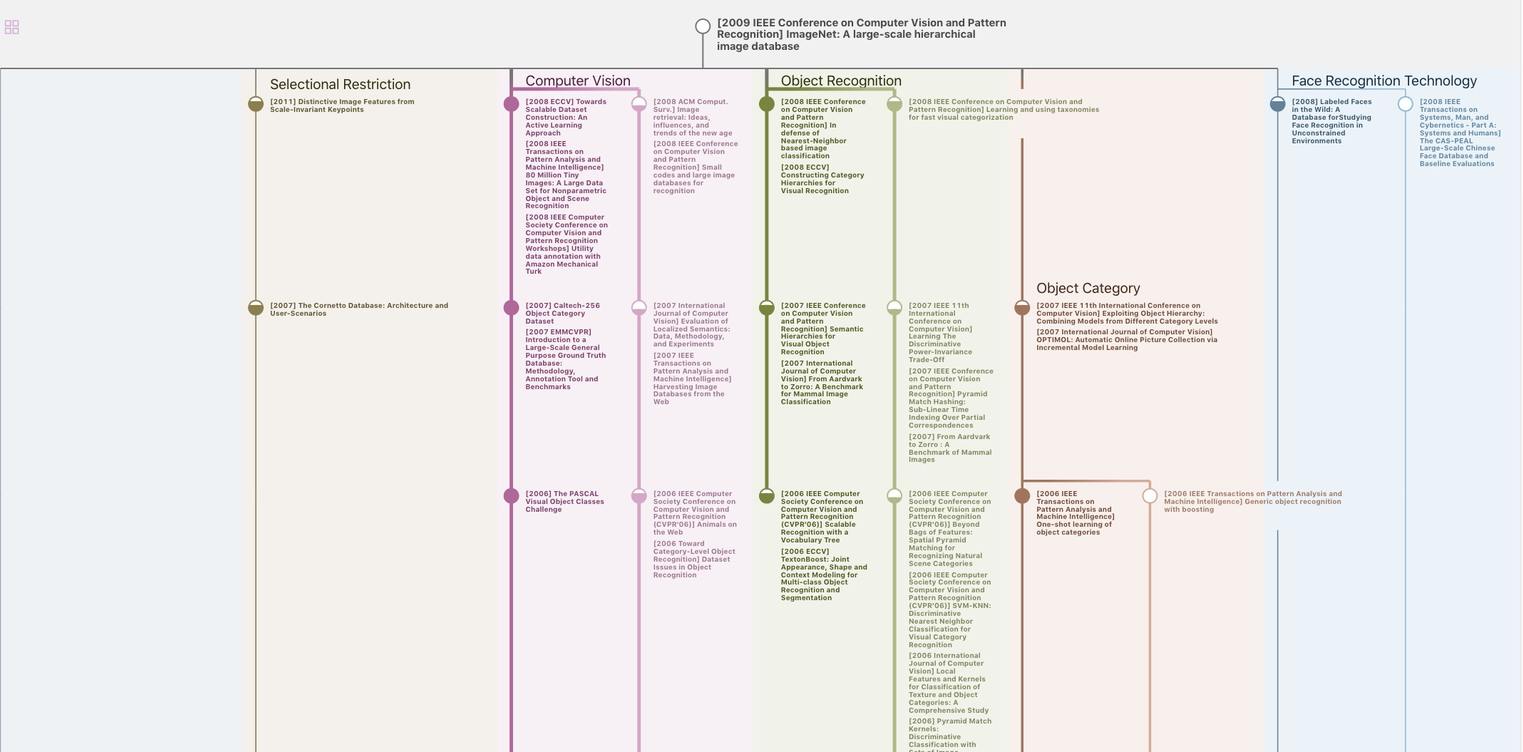
生成溯源树,研究论文发展脉络
Chat Paper
正在生成论文摘要