Subspace-based multi-view fusion for instance-level image retrieval
The Visual Computer(2020)
摘要
In this paper, we address the problem of multiple features fusion in instance-level image retrieval. Achieving tremendous success in recent retrieval task, convolutional neural network (CNN) features are capable of encoding high-level image contents and demonstrate unrivaled superiority to the hand-crafted shallow image signatures. However, the shallow features still play a beneficial role in visual matching particularly when dramatic variances in viewpoint and scale are present, since they inherit certain invariance from the local robust descriptor, e.g., scale-invariant feature transform (SIFT). Thus, it is important to leverage the mutual correlation between these two heterogeneous signatures for effective visual representation. Since it is still an open problem, in this paper, we propose a subspace-based multi-view fusion strategy where a shared subspace is uncovered from the original high-dimensional features yielding a compact latent representation. Experiments on six public benchmark datasets reveal the proposed method works better than other classical fusion approaches and achieve the state-of-the-art performance.
更多查看译文
关键词
Instance retrieval, Deep neural network, Shallow features, Multi-view fusion, Latent representations
AI 理解论文
溯源树
样例
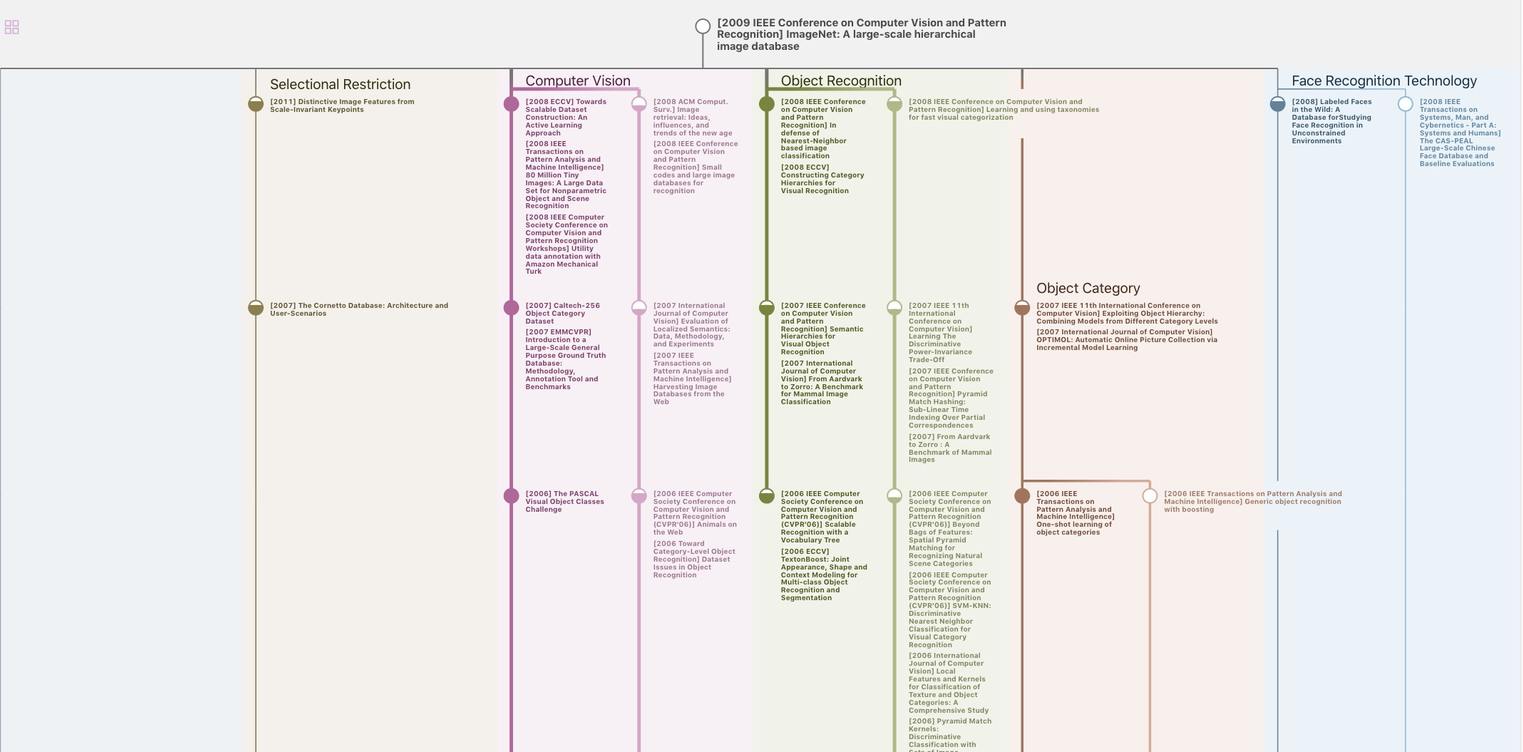
生成溯源树,研究论文发展脉络
Chat Paper
正在生成论文摘要