HUSBoost: A Hubness-Aware Boosting for High-Dimensional Imbalanced Data Classification
2019 International Conference on Machine Learning and Data Engineering (iCMLDE)(2019)
摘要
Learning from high-dimensional imbalanced data is a challenging research problem in machine learning, due to the curse of dimensionality caused by high dimension and the learning bias resulted from class imbalance. The existing works generally apply dimension reduction methods to reduce the dimensionality of features first, and then deal with the class imbalance problem by traditional imbalanced learning technologies. However, dimensionality reduction may cause the loss of useful information and cannot effectively address the problem of hubness which is an important aspect of the curse of dimensionality. In this paper, we present a hubness-aware cluster-based ensemble algorithm, HUSBoost, for learning high- dimensional imbalanced data. For hubs induced by high dimensionality, HUSBoost introduces discount factors to slow down the excessive growth of their weights, so as to alleviate the negative impacts of "bad" hubs on the classification decisions of component classifiers. To address the class imbalance problem, HUSBoost utilizes a cluster-based majority undersampling method to correct imbalanced class distribution. Specifically, k- hubs clustering technology is used to divide the majority samples into multiple clusters, and then the representative majority samples are selected from each cluster so as to form the balanced class distribution. Experimental results based on sixteen high-dimensional imbalanced data sets show the effectiveness of HUSBoost.
更多查看译文
关键词
Hubness,class imbalance,high dimension,undersampling,AdaBoost
AI 理解论文
溯源树
样例
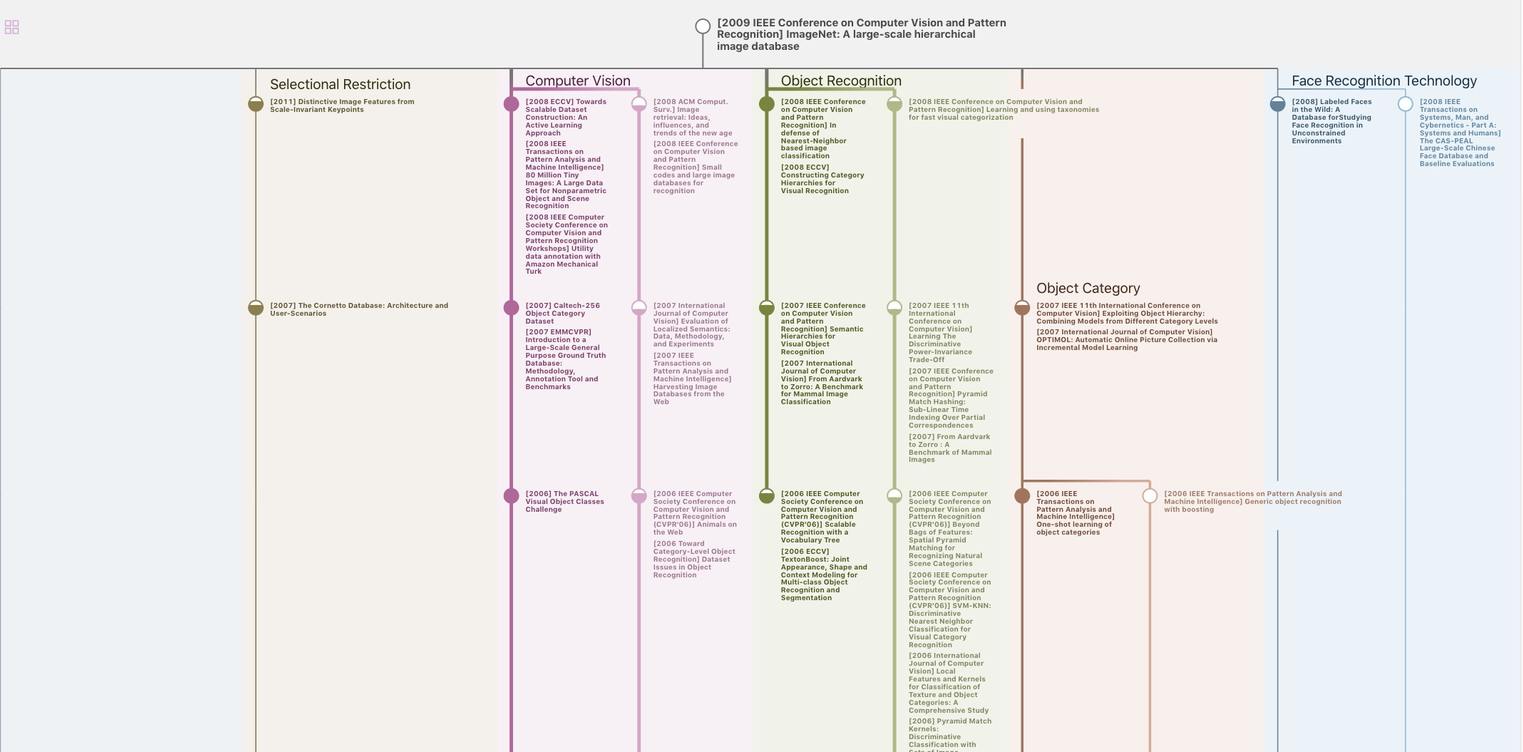
生成溯源树,研究论文发展脉络
Chat Paper
正在生成论文摘要