Abstract P4-10-13: Validation of neural network approach for the prediction of HER2-targeted neoadjuvant chemotherapy response from pretreatment MRI: A multi-site study
CANCER RESEARCH(2020)
摘要
Background: Although the advent of targeted therapy has substantially improved outcomes for HER2+ breast cancer patients, many will still fail to achieve pathological complete response (pCR) following neoadjuvant chemotherapy (NAC). In order to reduce overtreatment among patients resistant to standard HER2-targeted NAC and identify candidates for alternative therapeutic interventions, there is a need for validated markers of anti-HER2 agent benefit. The computational analysis of pretreatment imaging has shown recent promise in identifying responsive breast cancers. However, previous applications have explored response prediction among cohorts of mixed subtype and therapeutic approach, thus limiting its relevance in informing specific therapeutic strategy. Methods: This study comprised retrospective contrast-enhanced MRI data from a total of 159 patients who received anti-HER2 therapy at 5 institutions. A deep learning (DL) model was trained and tuned using 100 HER2+ breast cancer patients who received neoadjuvant taxane (T), carboplatin (C), trastuzumab (H), and pertuzumab (P) at Institution A, of which 49 achieved pCR (ypT0/is). A convolutional neural network was designed to analyze pre- and post-contrast MRI images acquired before NAC and compute a patient9s probability of achieving pCR. Institution A data was split randomly into a 85 patient training cohort, used to directly train the model, and a 15 patient internal validation cohort, used to monitor and improve training progress. Two external, held-out testing datasets were used to evaluate capability to predict response in HER2+: Testing Cohort 1, consisting of 28 patients (16 pCR, 12 non-pCR) treated with TCHP at Institution B, and Testing Cohort 2, consisting of 29 patients (10 pCR, 19 non-pCR) who received TCH at one of 3 other institutions as part of the BrUOG 211B trial. A multivariable clinical model (MCM) incorporating age, ER/PR status, stage, and size was evaluated separately and in combination with the neural network. Performance was assessed by area under the receiver operating characteristic curve (AUC), accuracy, sensitivity, and specificity. Results: The neural network was able to strongly predict response to HER2-targeted NAC in the internal validation (AUC=.93) and testing cohorts (AUC=.84 and AUC=.77). This model offered superior performance compared to a MCM, which performed poorly across institutions. Strikingly, the higher accuracy of DL included correctly identifying responders within the ER+/PR+ subgroup of patients and non-responders within the ER-/PR- subgroup. Combining DL predictions with the clinical model improved performance to AUC of 0.89 in testing cohort 1, but did not improve AUC in cohort 2. Conclusions: DL analysis of breast DCE-MRI could be used to better identify benefit of HER2-targeted therapeutic approaches prior to administration. As the first exploration of automated response prediction from imaging with respect to a targeted NAC approach, this work uniquely has the potential to help guide therapeutic decision-making. Our approach was effective in predicting response to multiple HER2-targeted NAC regimens, with better performance in the cohort who received TCHP (as in the training cohort). The strong performance of this model across 5 institutions is a promising indicator of its robustness and ability to tailor therapy even within clinically-distinct HER2+ patient subpopulations. Citation Format: Nathaniel Braman, Mohammed El Adoui, Manasa Vulchi, Paulette Turk, Maryam Etesami, Pingfu Fu, Stylianos Drisis, Vinay Varadan, Donna Plecha, Mohammed Benjelloun, Jame Abraham, Anant Madabhushi. Validation of neural network approach for the prediction of HER2-targeted neoadjuvant chemotherapy response from pretreatment MRI: A multi-site study [abstract]. In: Proceedings of the 2019 San Antonio Breast Cancer Symposium; 2019 Dec 10-14; San Antonio, TX. Philadelphia (PA): AACR; Cancer Res 2020;80(4 Suppl):Abstract nr P4-10-13.
更多查看译文
AI 理解论文
溯源树
样例
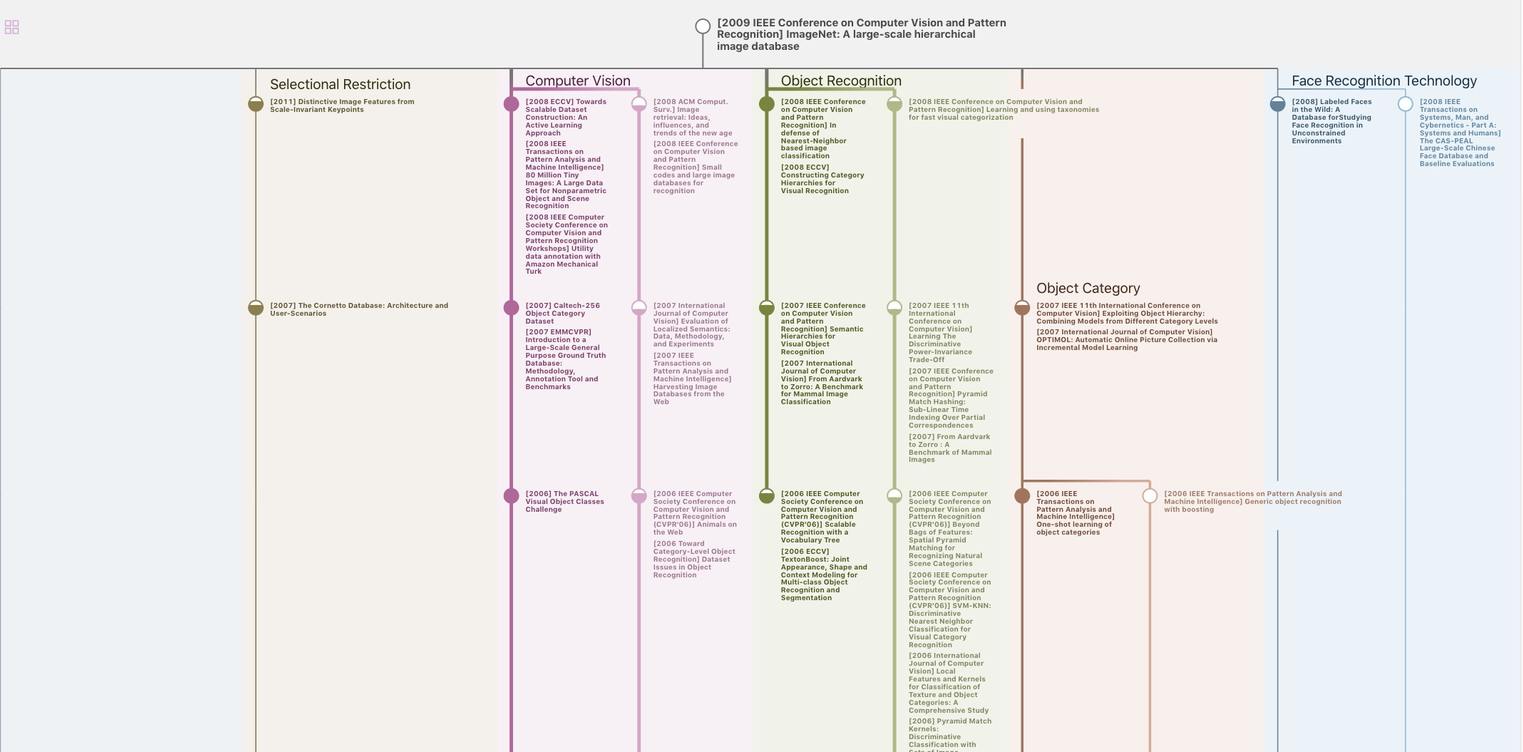
生成溯源树,研究论文发展脉络
Chat Paper
正在生成论文摘要