Online Learning Enabled Task Offloading for Vehicular Edge Computing
IEEE Wireless Communications Letters(2020)
摘要
Vehicular edge computing pushes the cloud computing capability to the distributed network edge nodes, enabling computation-intensive and latency-sensitive computing services for smart vehicles through task offloading. However, the inherent mobility introduces fast variation of network structure, which are usually unknown
a priori
. In this letter, we formulate the vehicular task offloading as a mortal multi-armed bandit problem, and develop a new online algorithm to enable distributed decision making on the node selection. The key is to exploit the contextual information of edge nodes and transform the infinite exploration space to a finite one. Theoretically, we prove that the proposed algorithm has a sublinear learning regret. Simulation results verify its effectiveness.
更多查看译文
关键词
Task analysis,Space exploration,Energy consumption,Benchmark testing,Edge computing,Central Processing Unit,Delays
AI 理解论文
溯源树
样例
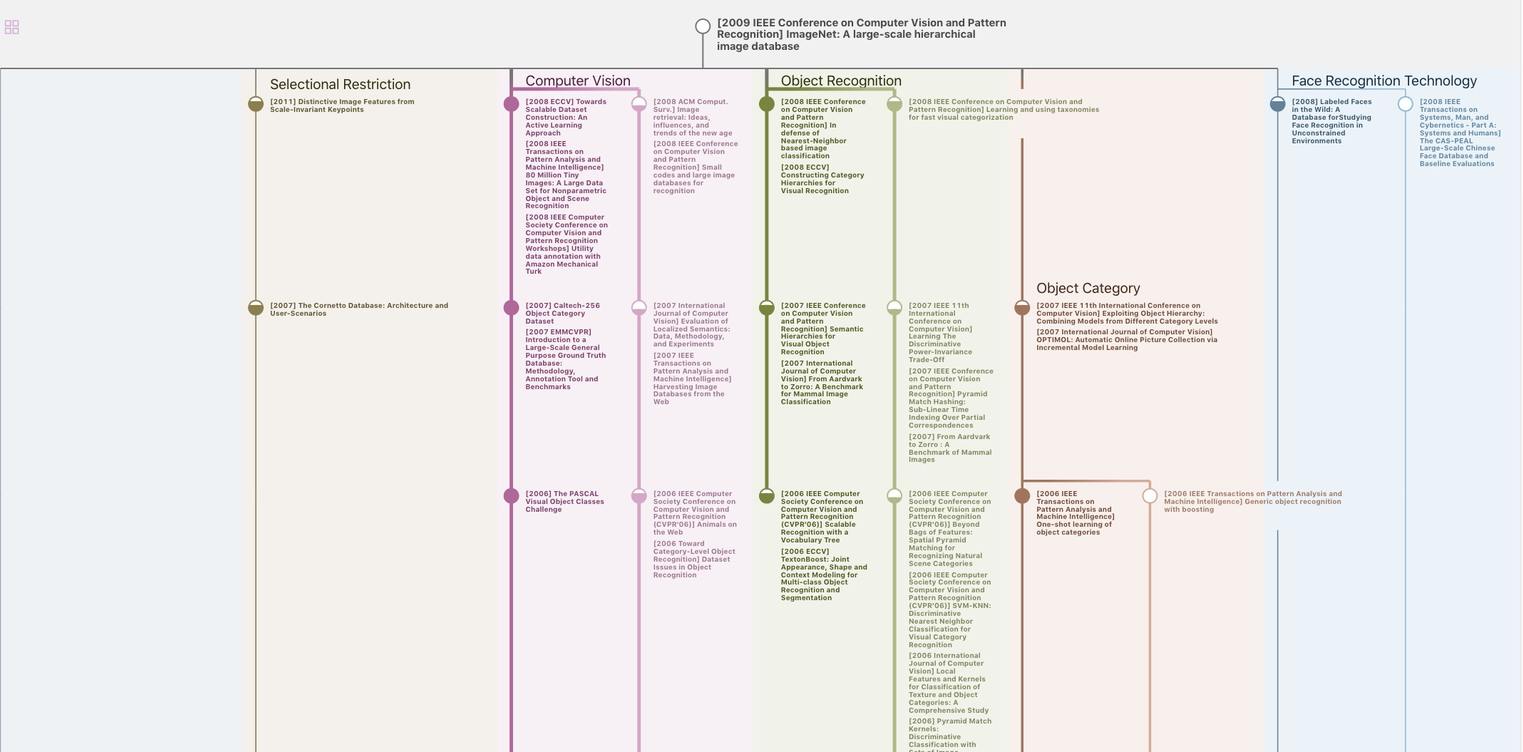
生成溯源树,研究论文发展脉络
Chat Paper
正在生成论文摘要