Improving The Performance Of Transient Stability Prediction Using Resampling Methods
2019 11TH INTERNATIONAL CONFERENCE ON ELECTRICAL AND ELECTRONICS ENGINEERING (ELECO 2019)(2019)
摘要
Detection of evolving transient instabilities in power systems is of high importance in order to maintain the system's security and integrity. With the recent developments on wide area monitoring systems, employing machine learning models for transient stability assessment has drawn a great attention. Nevertheless, as the power systems are being designed and operated in a secure and robust manner, the ratio of the contingencies that lead to transient instability to the ones that do not is relatively low. This makes the learning problem harder for such systems as the training data would be inherently imbalanced. In this work, we exploit the resampling techniques to tackle the imbalanced learning problem by utilizing three different over-sampling methods: random over-sampling, SMOTE and ADASYN. The XGBoost classifier model is adopted within the proposed framework to compare the performance improvements through each over-sampling method. The results obtained in the Nordic power system show notable improvements, especially when unequal misclassification costs are considered.
更多查看译文
关键词
transient stability prediction,resampling methods,transient instability,wide area monitoring systems,machine learning models,transient stability assessment,imbalanced learning problem,XGBoost classifier model,Nordic power system
AI 理解论文
溯源树
样例
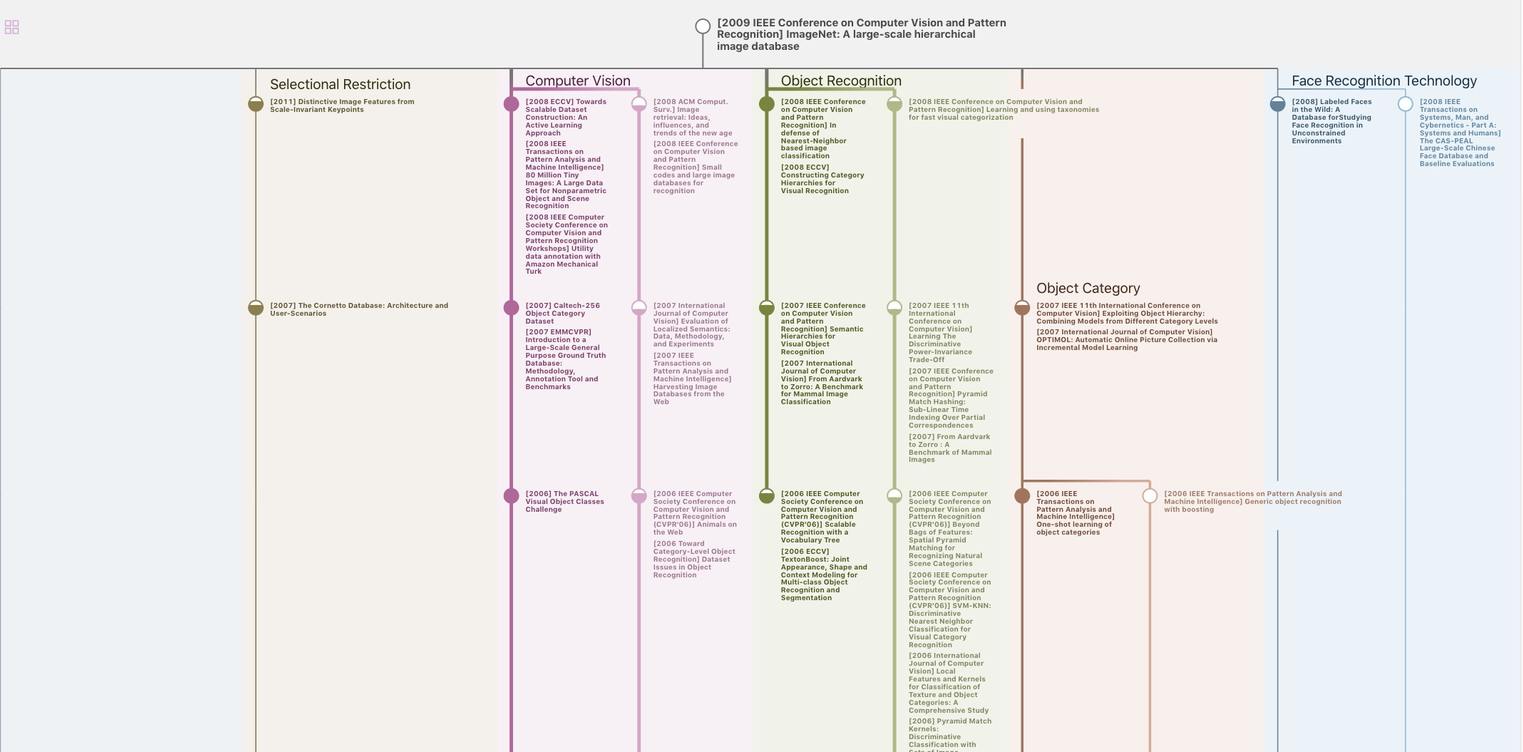
生成溯源树,研究论文发展脉络
Chat Paper
正在生成论文摘要