Linear Mixed Models Minimise False Positive Rate And Enhance Precision Of Mass Univariate Vertex-Wise Analyses Of Grey-Matter
2020 IEEE 17TH INTERNATIONAL SYMPOSIUM ON BIOMEDICAL IMAGING (ISBI 2020)(2020)
摘要
We evaluated the statistical power, family wise error rate (FWER) and precision of several competing methods that perform mass-univariate vertex-wise analyses of grey-matter (thickness and surface area). In particular, we compared several generalised linear models (GLMs, current state of the art) to linear mixed models (LMMs) that have proven superior in genomics. We used phenotypes simulated from real vertex-wise data and a large sample size (N=8,662) which may soon become the norm in neuroimaging.No method ensured a FWER<5% (at a vertex or cluster level) after applying Bonferroni correction for multiple testing. LMMs should be preferred to GLMs as they minimise the false positive rate and yield smaller clusters of associations. Associations on real phenotypes must be interpreted with caution, and replication may be warranted to conclude about an association.
更多查看译文
关键词
Grey-matter, mass univariate vertex-wise analyses, simulations, false positive, precision
AI 理解论文
溯源树
样例
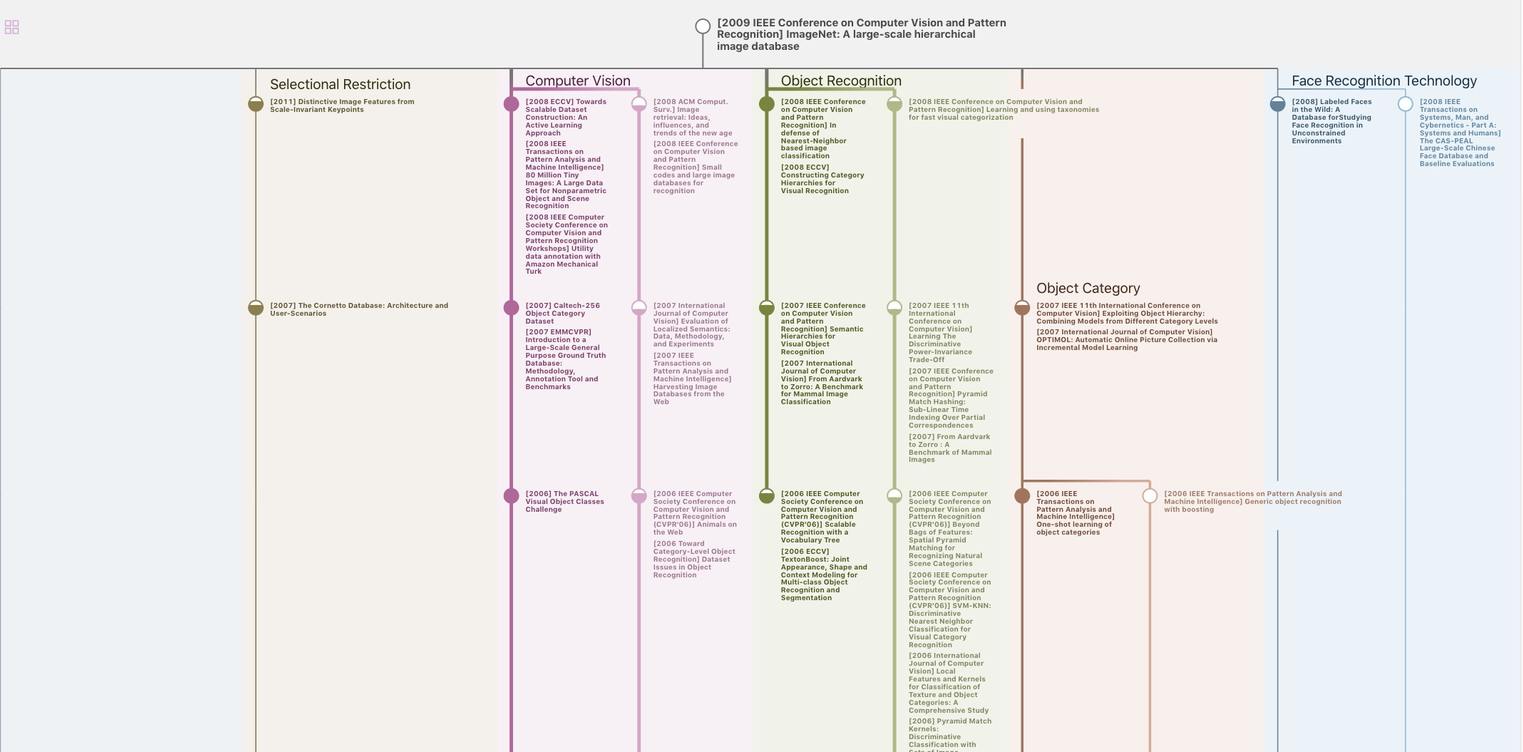
生成溯源树,研究论文发展脉络
Chat Paper
正在生成论文摘要