Distribution-Invariant Deep Belief Network for Intelligent Fault Diagnosis of Machines Under New Working Conditions
IEEE Transactions on Industrial Electronics(2021)
摘要
As a deep learning model, a deep belief network (DBN) consists of multiple restricted Boltzmann machines (RBMs). Based on DBN, many intelligent fault diagnosis methods are proposed. However, these methods seldom considered the appearance of new working conditions during the operation of real machines. Varying working conditions lead to a change of feature distributions and finally result in low diagnosis accuracies. Therefore, we propose a distribution-invariant DBN (DIDBN) to learn distribution-invariant features directly from raw vibration data. DIDBN consists of a locally connected RBM (LCRBM) layer, a fully connected RBM layer, and an RBM layer with a mean discrepancy maximum (MDM-RBM). The LCRBM layer is designed with a locally connected structure. By proposing MDM, the MDM-RBM layer is able to obtain features that have close distributions under varying working conditions. Followed by a softmax classifier, DIDBN is able to recognize faults. The proposed method is applied to two diagnosis cases. Results verify that DIDBN is able to learn distribution-invariant features and achieve higher diagnosis accuracies than recently proposed methods. Moreover, the reason why DIDBN is able to learn distribution-invariant features is explained by visualizing the feature learning process.
更多查看译文
关键词
Deep belief network (DBN),feature visualization,intelligent fault diagnosis,new working condition,transfer learning (TL)
AI 理解论文
溯源树
样例
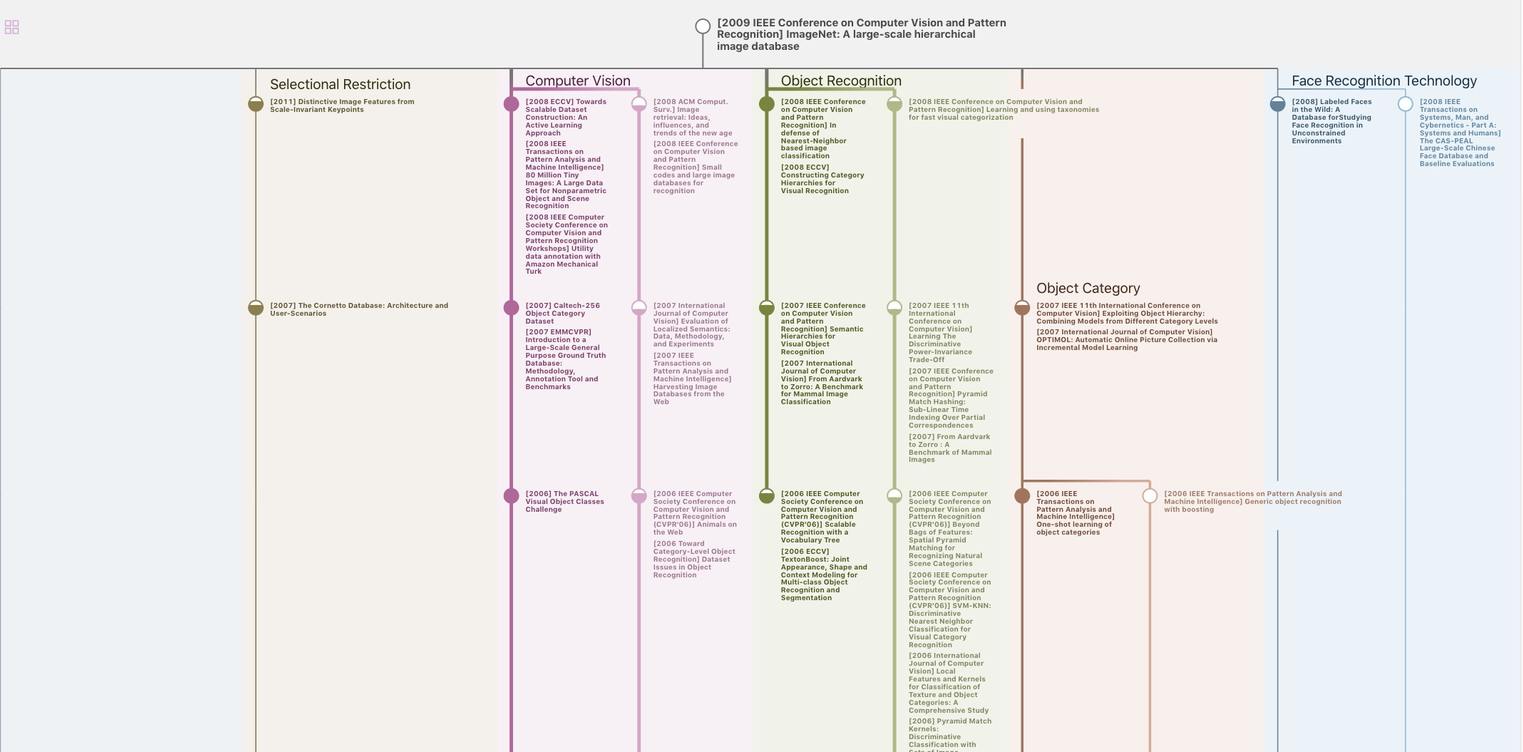
生成溯源树,研究论文发展脉络
Chat Paper
正在生成论文摘要