Inspection on Ball Bearing Malfunction by Chen-Lee Chaos System
IEEE ACCESS(2020)
摘要
With the trend of Industry 4.0, the global machine tool industry is developing towards smart manufacturing. The ball bearing is a key component of the rotary axis of machine tool, and its functionality is to bear the external load on the axis as well as maintain the center position of the axis. A damaged bearing will result in abnormal vibration and noise, and thus will lead to the damage of the machine and produced workpieces. Therefore, inspection and identification of ball bearing failures is particularly important. This paper discusses the fault signals of ball bearings published by the Society for Machinery Failure Prevention Technology (MFPT) and creates a recognition model for the ball bearing state based on different fault states, and then we adopt two different approaches for feature extraction. The first approach implements Finite Impulse Response Filter (FIR) and Approximate Entropy (ApEn) to extract the signal features. The second approach utilizes the Chen-Lee chaotic system for analysis and takes its chaotic attractor as the feature of the state recognition. The comparison of model recognition accuracy for Back Propagation Neural Network (BPNN), Support Vector Machine (SVM), and K Nearest Neighbor (KNN) was conducted after acquiring the features through the two approaches in this paper. The results of the experiments in this paper show that both of the feature extraction approaches enable the state to be recognized easily. The Chen-Lee chaotic system with BPNN not only reaches 100% identification rate and it has the highest overall efficiency; it takes only 0.054 second to complete the feature extraction for 63 sets of data; this study is able to provide timely and precise solution for the failure of key mechanical components.
更多查看译文
关键词
Ball bearing,back propagation neural network,support vector machine,fault detection,approximate entropy,Chen-Lee chaotic system
AI 理解论文
溯源树
样例
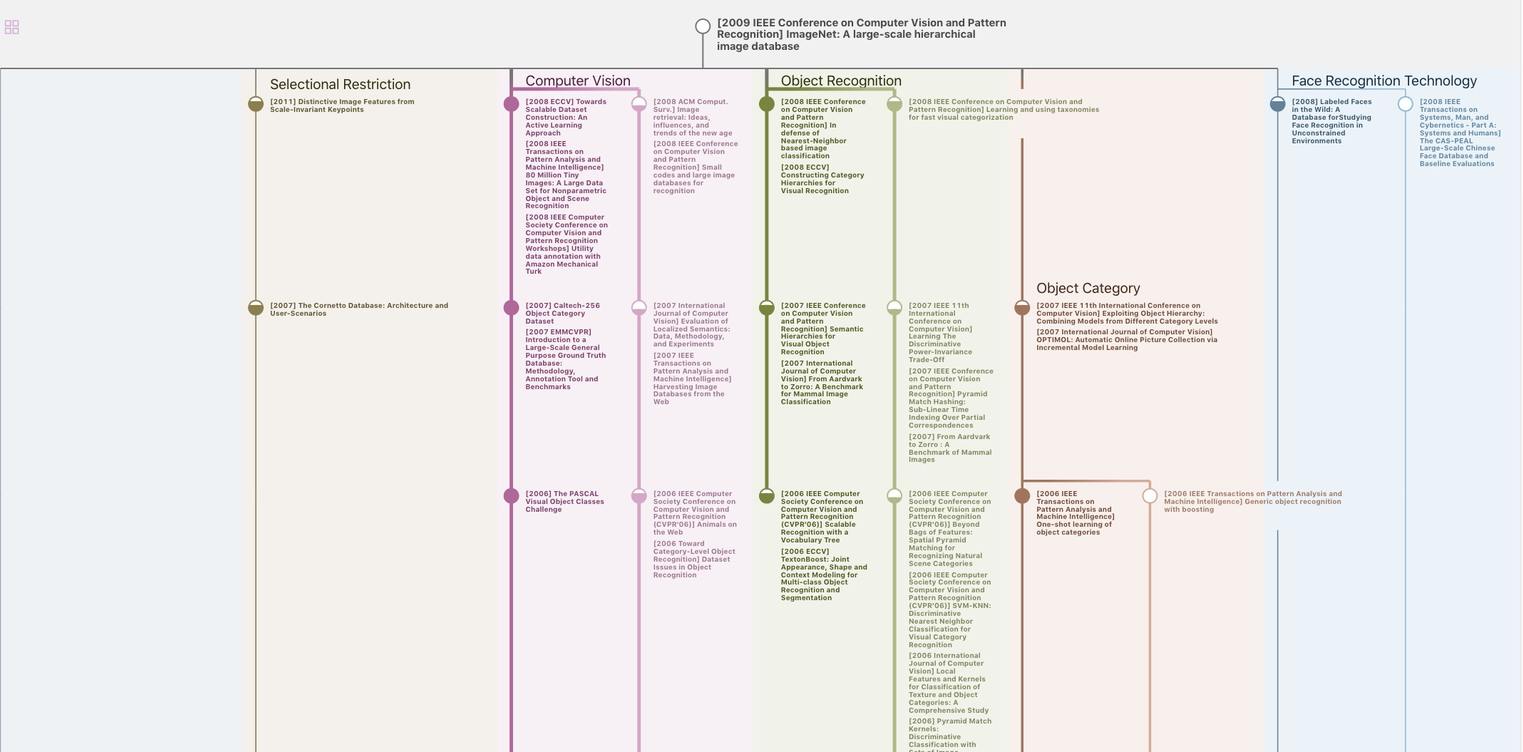
生成溯源树,研究论文发展脉络
Chat Paper
正在生成论文摘要