Data analytics for the sustainable use of resources in hospitals: Predicting the length of stay for patients with chronic diseases
Information & Management(2022)
摘要
Various factors are behind the forces that drive hospitals toward more sustainable operations. Hospitals contracting with Medicare, for instance, are reimbursed for the procedures performed, regardless of the number of days that patients stay in the hospital. This reimbursement structure has incentivized hospitals to use their resources (such as their beds) more efficiently to maximize revenues. One way hospitals can improve bed utilization is by predicting patients’ length of stay (LOS) at the time of admission, the benefits of which extend to employees, communities, and the patients themselves. In this paper, we employ a data analytics approach to develop and test a deep learning neural network to predict LOS for patients with chronic obstructive pulmonary disease (COPD) and pneumonia. The theoretical contribution of our effort is that it identifies variables related to patients’ prior admissions as important factors in the prediction of LOS in hospitals, thereby revising the current paradigm in which patients’ medical histories are rarely considered for the prediction of LOS. The methodological contributions of our work include the development of a data engineering methodology to augment the data sets, prediction of LOS as a numerical (rather than a binary) variable, temporal evaluation of the training and validation data sets, and a significant improvement in the accuracy of predicting LOS for COPD and pneumonia inpatients. Our evaluations show that variables related to patients’ previous admissions are the main driver of the deep network’s superior performance in predicting the LOS as a numerical variable. Using the assessment criteria introduced in prior studies (i.e., ±2 days and ±3 days tolerance), our models are able to predict the length of hospital stay with 86 % and 91 % accuracy for the COPD data set, and with 74 % and 85 % accuracy for the pneumonia data set. Hence, our effort could help hospitals serve a larger number of patients with a fixed amount of resources, thereby reducing their environmental footprint while increasing their revenue, as well as their patients’ satisfaction.
更多查看译文
关键词
Data analytics,Length of hospital stay,Deep learning,Temporal evaluation,Sustainability
AI 理解论文
溯源树
样例
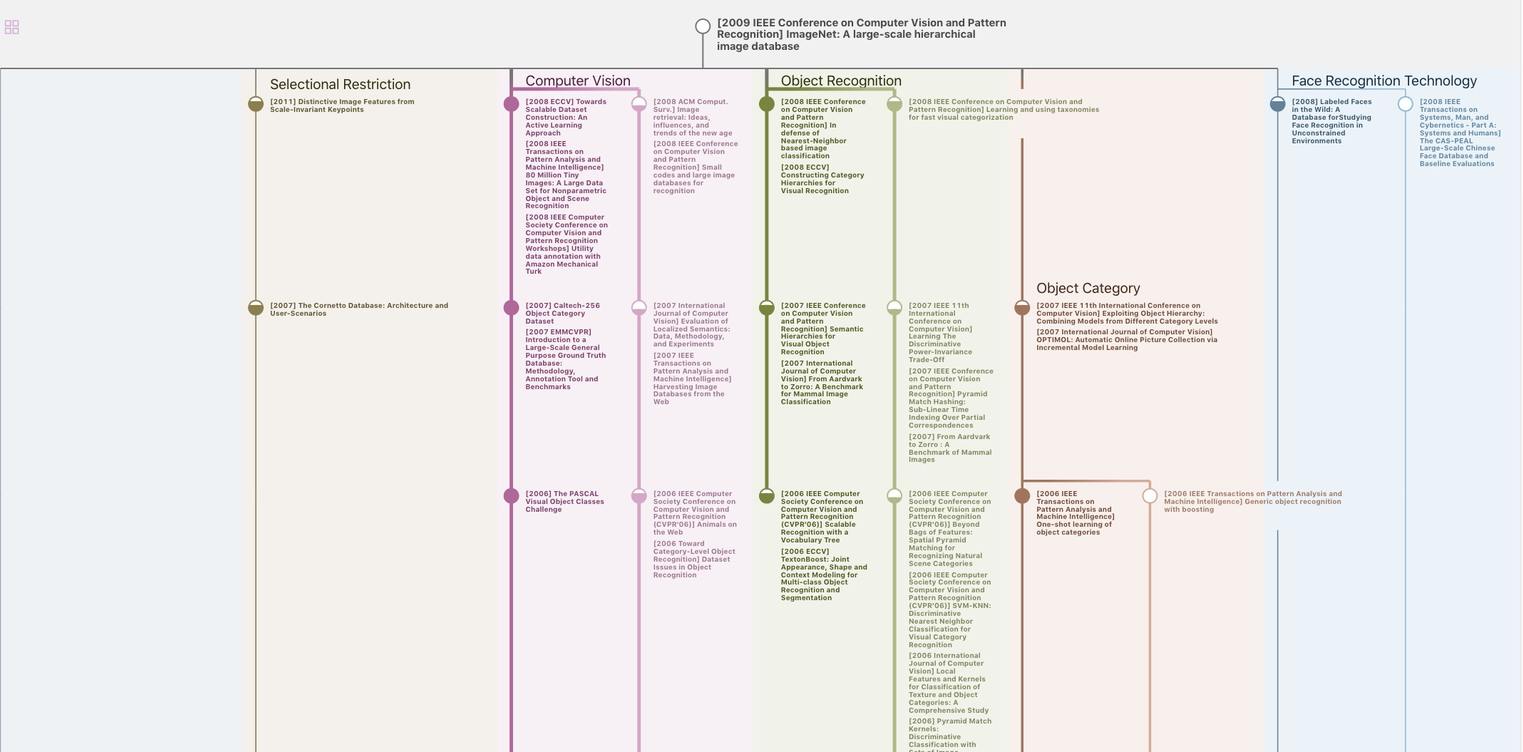
生成溯源树,研究论文发展脉络
Chat Paper
正在生成论文摘要