Generalized nuclear norm and Laplacian scale mixture based low-rank and sparse decomposition for video foreground-background separation
Signal Processing(2020)
摘要
•We extend the generalized nuclear norm (GNN) and the Laplacian scale mixture (LSM) to approximate the low-rank and sparse matrices respectively, and propose a generalized formulation which called GNNLSM for the nonconvex low-rank and sparse decomposition (LRSD) problem.•We solve the proposed GNNLSM problem via the alternating direction method of multipliers.•We give numerical results on synthetic data to validate the superior performance of the proposed method. We also use our proposed method to video foreground-background separation, and the experimental results show that the proposed method is more effective than other LRSD based video foreground-background separation methods.
更多查看译文
关键词
Low-rank and sparse decomposition,Generalized nuclear norm,Laplacian scale mixture,Alternating direction method of multipliers,Video foreground-background separation
AI 理解论文
溯源树
样例
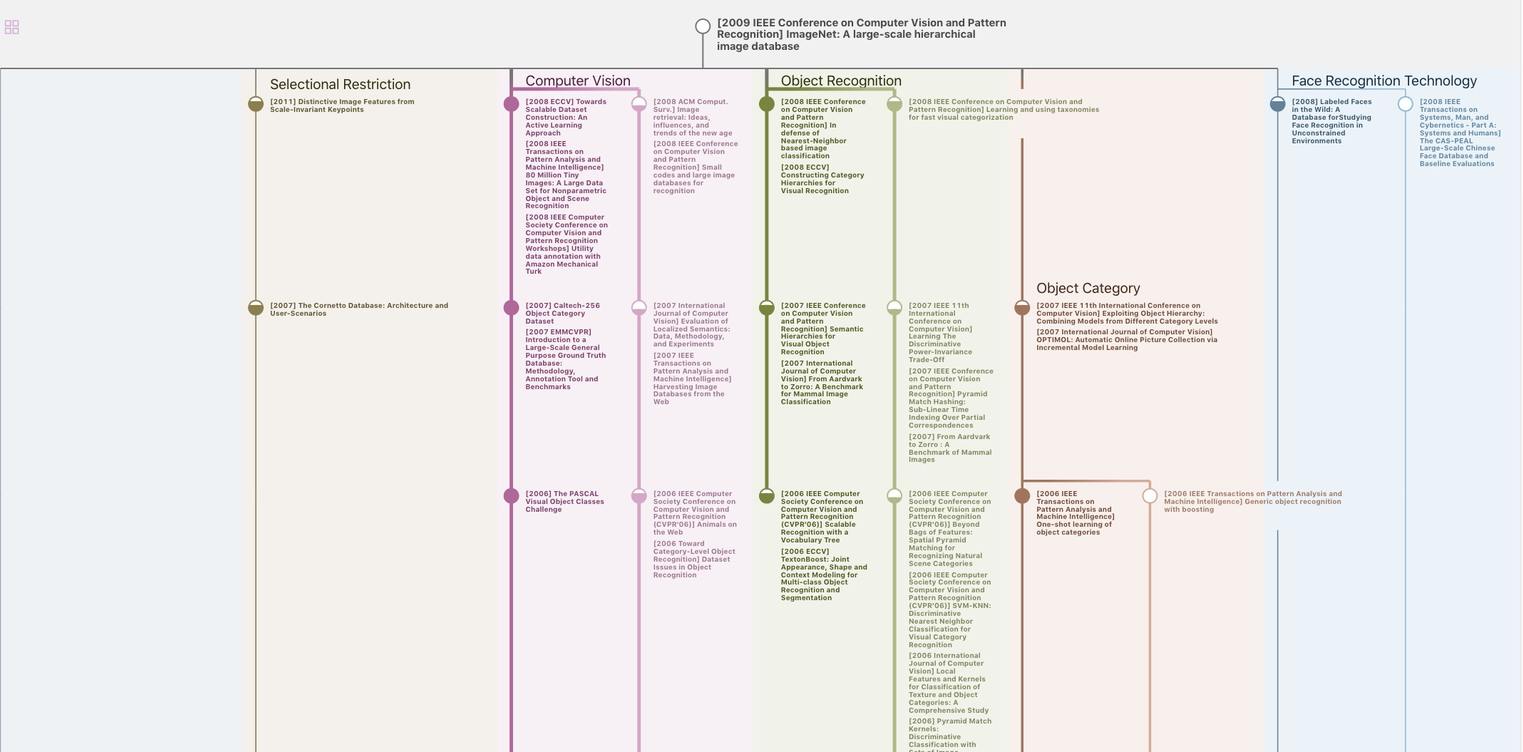
生成溯源树,研究论文发展脉络
Chat Paper
正在生成论文摘要