Dnn Plus Neurosim: An End-To-End Benchmarking Framework For Compute-In-Memory Accelerators With Versatile Device Technologies
2019 IEEE INTERNATIONAL ELECTRON DEVICES MEETING (IEDM)(2019)
摘要
DNN+NeuroSim is an integrated framework to benchmark compute-in-memory (CIM) accelerators for deep neural networks, with hierarchical design options from device-level, to circuit-level and up to algorithm-level. A python wrapper is developed to interface NeuroSim with popular machine learning platforms such as Pytorch and Tensorflow. The framework supports automatic algorithm to hardware mapping, and evaluates both chip-level performance and inference accuracy with hardware constraints. In this work, we analyze the impact of reliability in "analog" synaptic devices, and analog-to-digital converter (ADC) quantization effects on the inference accuracy. Then we benchmark CIM accelerators based on SRAM and versatile emerging devices including RRAM, PCM, FeFET and ECRAM, from VGG to ResNet, and from CIFAR to ImageNet dataset, revealing the benefits of high on-state resistance, e.g. by using three-terminal synapses. The open-source code of DNN+NeuroSim is available at haps://github.com/neurosim/DNN_NeuroSim_V1.0.
更多查看译文
AI 理解论文
溯源树
样例
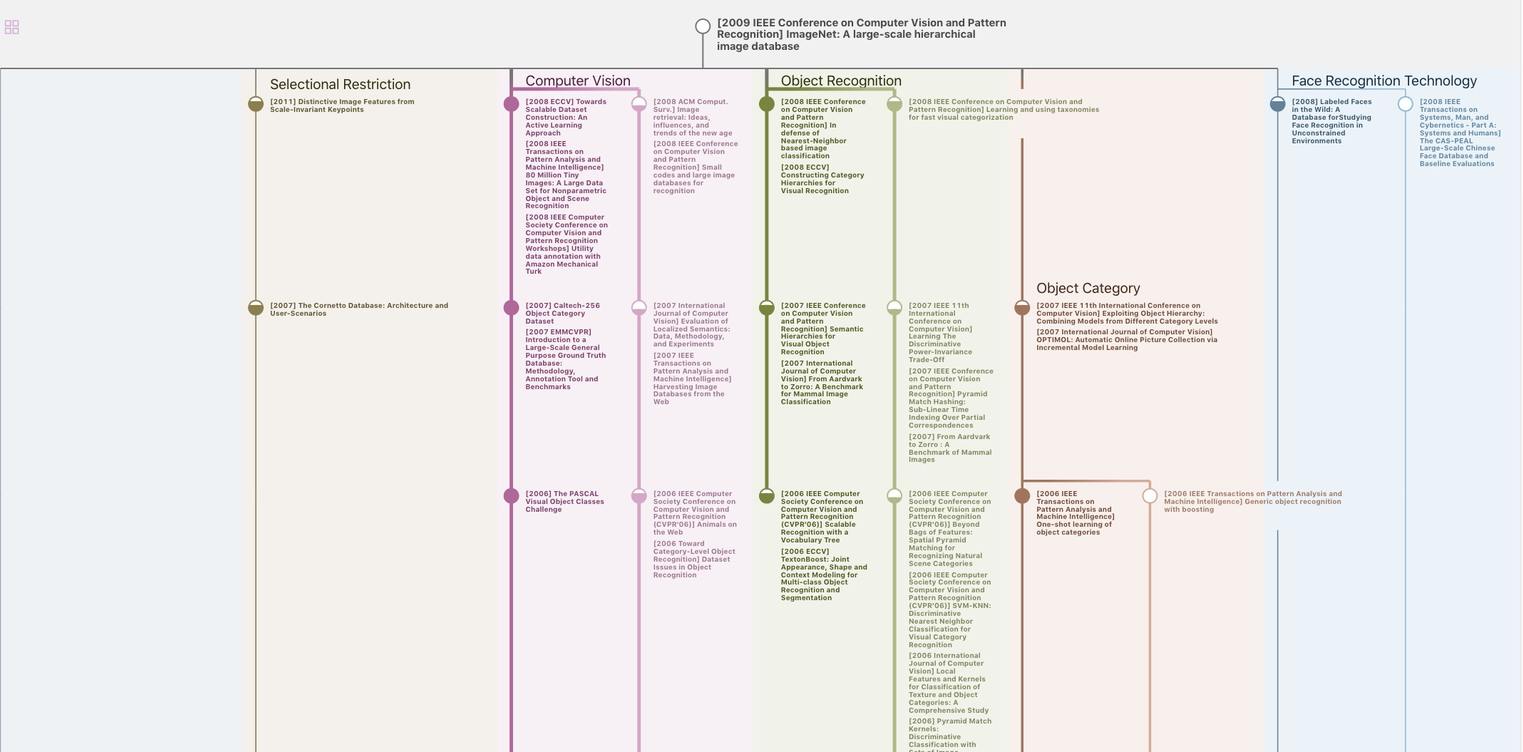
生成溯源树,研究论文发展脉络
Chat Paper
正在生成论文摘要