Second-order Monte Carlo sensitivities in linear or constant time
JOURNAL OF COMPUTATIONAL FINANCE(2020)
摘要
We consider the problem of efficiently computing the full matrix of second-order sensitivities of a Monte Carlo price when the number of inputs is large. Specifically, we analyze and compare methods with run times of at most O(NT), where N is the dimension of the input and T is the time required to compute the price. Since none of the alternatives from previous literature appears to be satisfactory in all settings, we propose two original methods: the first method is based on differentiation in a distributional sense, while the second method leverages a functional relation between first- and second-order derivatives. The former shows excellent generality and computational times to achieve a given target accuracy. The latter is by far the most effective in at least one relevant example and has theoretical interest in that it is the first practical estimator of the full Hessian whose complexity, as a multiple of that of the only-price implementation, does not grow with the dimension of the problem.
更多查看译文
关键词
Greeks,Gamma,algorithmic differentiation,derivatives pricing,distributional derivative,functional derivative
AI 理解论文
溯源树
样例
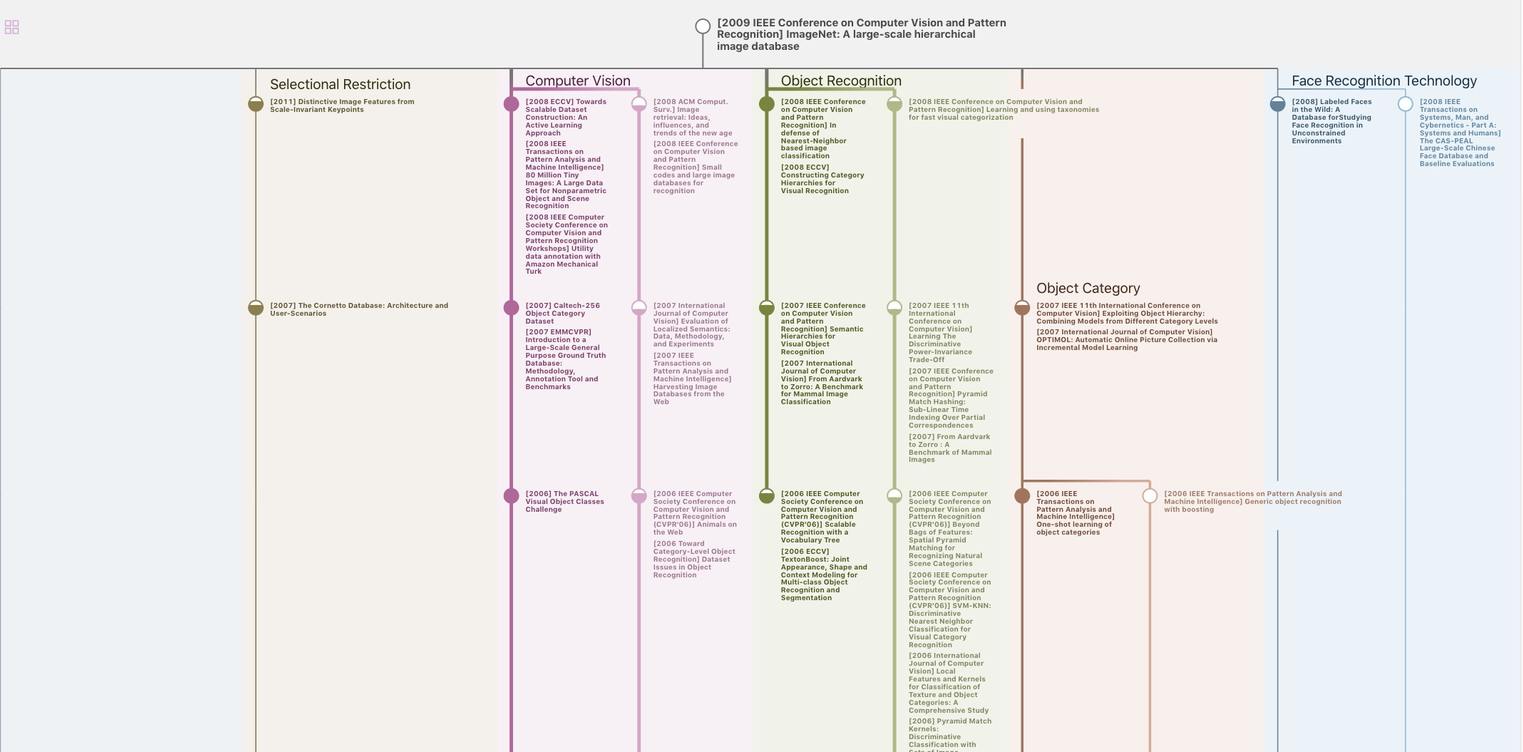
生成溯源树,研究论文发展脉络
Chat Paper
正在生成论文摘要