A data-driven approach for predicting printability in metal additive manufacturing processes
JOURNAL OF INTELLIGENT MANUFACTURING(2020)
摘要
Metal powder-bed fusion additive manufacturing technologies offer numerous benefits to the manufacturing industry. However, the current approach to printability analysis, determining which components are likely to build unsuccessfully, prior to manufacture, is based on ad-hoc rules and engineering experience. Consequently, to allow full exploitation of the benefits of additive manufacturing, there is a demand for a fully systematic approach to the problem. In this paper we focus on the impact of geometry in printability analysis. For the first time, we detail a machine learning framework for determining the geometric limits of printability in additive manufacturing processes. This framework consists of three main components. First, we detail how to construct strenuous test artefacts capable of pushing an additive manufacturing process to its limits. Secondly, we explain how to measure the printability of an additively manufactured test artefact. Finally, we construct a predictive model capable of estimating the printability of a given artefact before it is additively manufactured. We test all steps of our framework, and show that our predictive model approaches an estimate of the maximum performance obtainable due to inherent stochasticity in the underlying additive manufacturing process.
更多查看译文
关键词
Additive manufacturing,Machine learning,Powder bed fusion,Electron beam melting,Printability analysis
AI 理解论文
溯源树
样例
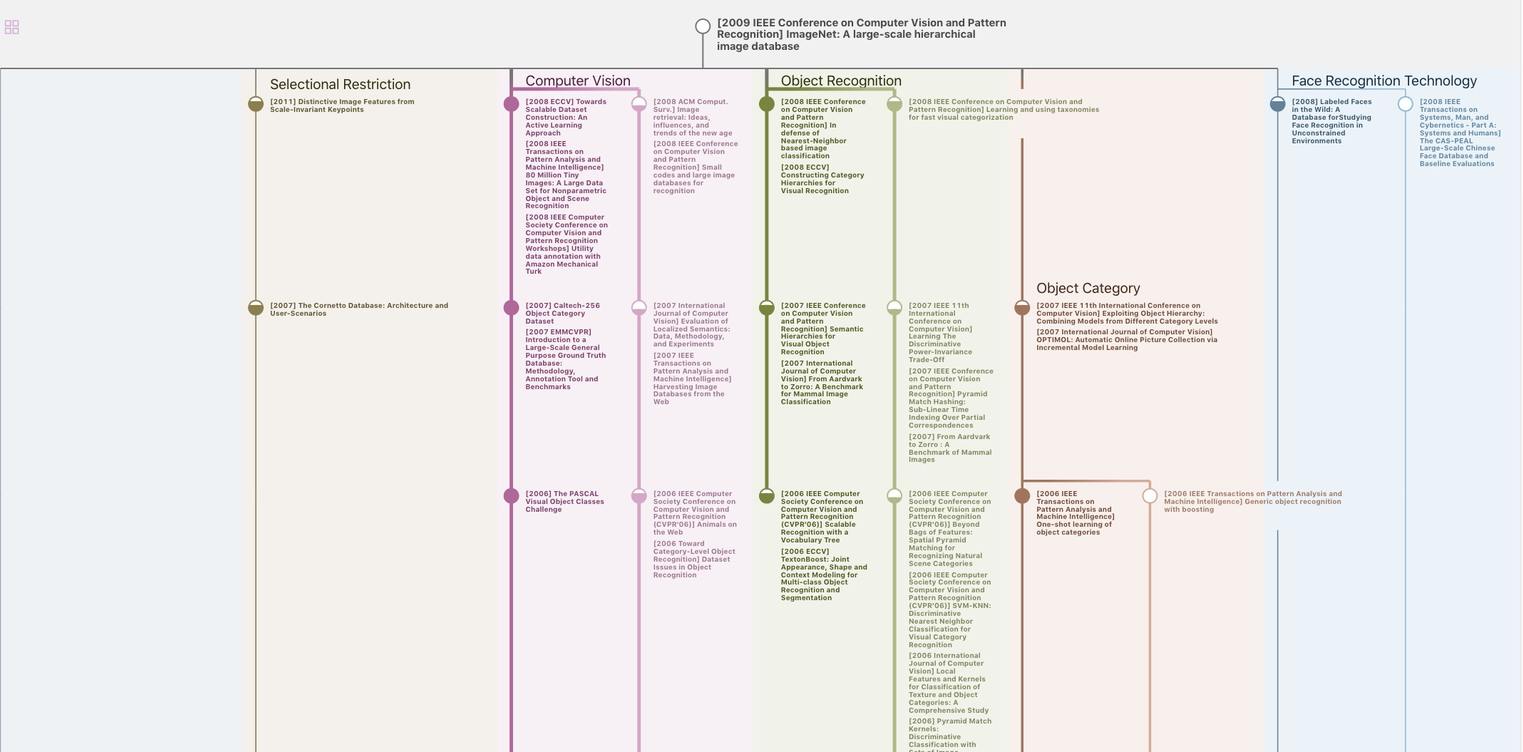
生成溯源树,研究论文发展脉络
Chat Paper
正在生成论文摘要