An Enhanced Design of Sparse Autoencoder for Latent Features Extraction Based on Trigonometric Simplexes for Network Intrusion Detection Systems
ELECTRONICS(2020)
摘要
Despite the successful contributions in the field of network intrusion detection using machine learning algorithms and deep networks to learn the boundaries between normal traffic and network attacks, it is still challenging to detect various attacks with high performance. In this paper, we propose a novel mathematical model for further development of robust, reliable, and efficient software for practical intrusion detection applications. In this present work, we are concerned with optimal hyperparameters tuned for high performance sparse autoencoders for optimizing features and classifying normal and abnormal traffic patterns. The proposed framework allows the parameters of the back-propagation learning algorithm to be tuned with respect to the performance and architecture of the sparse autoencoder through a sequence of trigonometric simplex designs. These hyperparameters include the number of nodes in the hidden layer, learning rate of the hidden layer, and learning rate of the output layer. It is expected to achieve better results in extracting features and adapting to various levels of learning hierarchy as different layers of the autoencoder are characterized by different learning rates in the proposed framework. The idea is viewed such that every learning rate of a hidden layer is a dimension in a multidimensional space. Hence, a vector of the adaptive learning rates is implemented for the multiple layers of the network to accelerate the processing time that is required for the network to learn the mapping towards a combination of enhanced features and the optimal synaptic weights in the multiple layers for a given problem. The suggested framework is tested on CICIDS2017, a reliable intrusion detection dataset that covers all the common, updated intrusions and cyber-attacks. Experimental results demonstrate that the proposed architecture for intrusion detection yields superior performance compared to recently published algorithms in terms of classification accuracy and F-measure results.
更多查看译文
关键词
intrusion detection system,tuning hyperparameters,Hassan-Nelder-Mead,sparse autoencoder,adaptive learning rates,extracting features,over-fitting problem
AI 理解论文
溯源树
样例
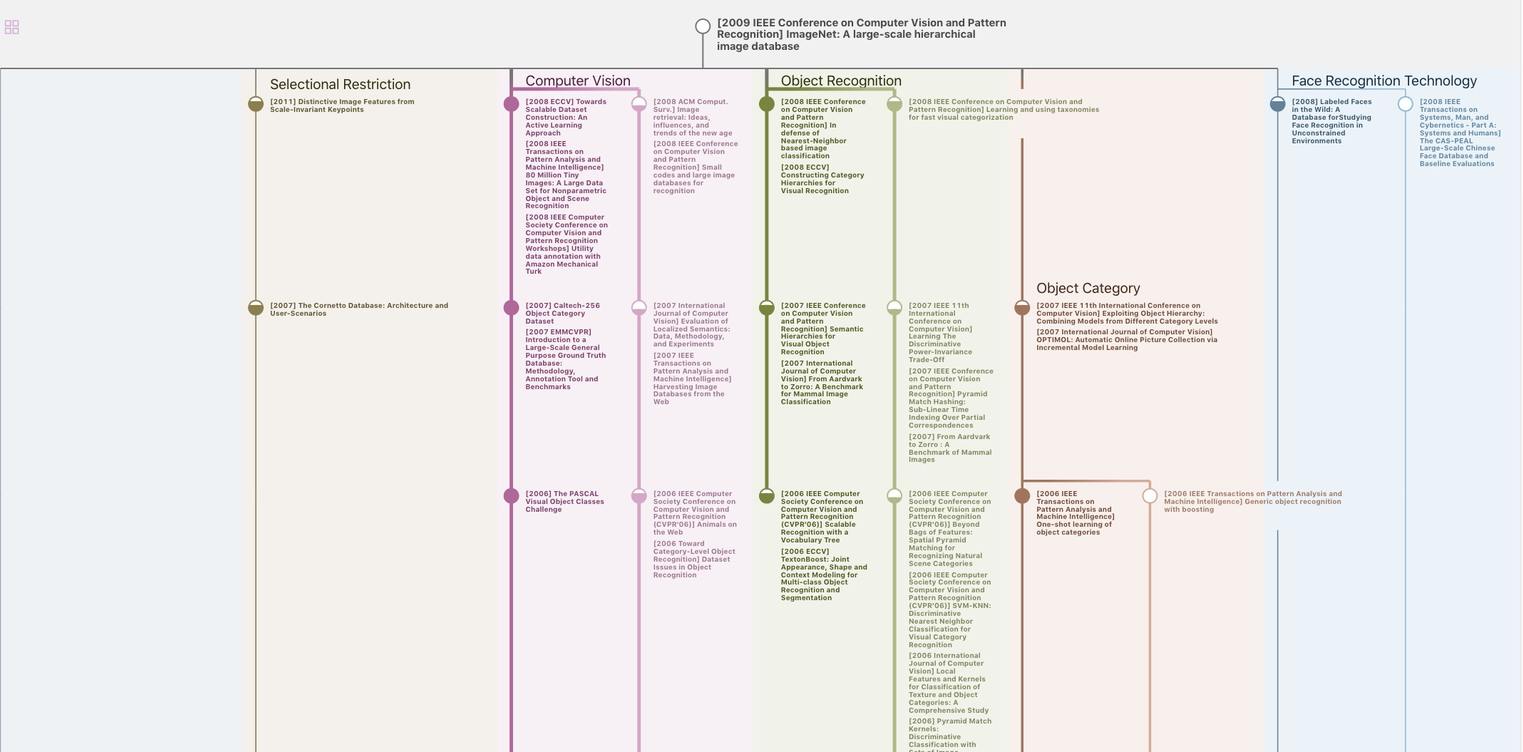
生成溯源树,研究论文发展脉络
Chat Paper
正在生成论文摘要