On the Use of Tiny Convolutional Neural Networks for Human-Expert-Level Classification Performance in Sonar Imagery
IEEE Journal of Oceanic Engineering(2021)
摘要
Efficient convolutional neural networks (CNNs) are designed and trained for an underwater target classification task with synthetic aperture sonar (SAS) imagery collected at sea. The main contribution is demonstrating that classification performance that matches, and even surpasses, the level achievable by a human domain expert obtained from tiny CNNs with three to six orders of magnitude fewer parameters than have traditionally been used in the literature. In doing so, this work represents the first large-scale classification study in the sonar domain to establish a favorable comparison between automated algorithm performance and human ability. Extensive experimental results on challenging real-world SAS image data sets collected in diverse environments and conditions demonstrate that the CNNs possess strong generalization ability. These findings should significantly impact the manner in which CNNs are utilized in the underwater remote-sensing community. To wit, the tiny CNNs proposed here provide a blueprint for achieving excellent classification performance even with limited computing power or limited data.
更多查看译文
关键词
Machine learning,neural networks,pattern recognition,synthetic aperture sonar
AI 理解论文
溯源树
样例
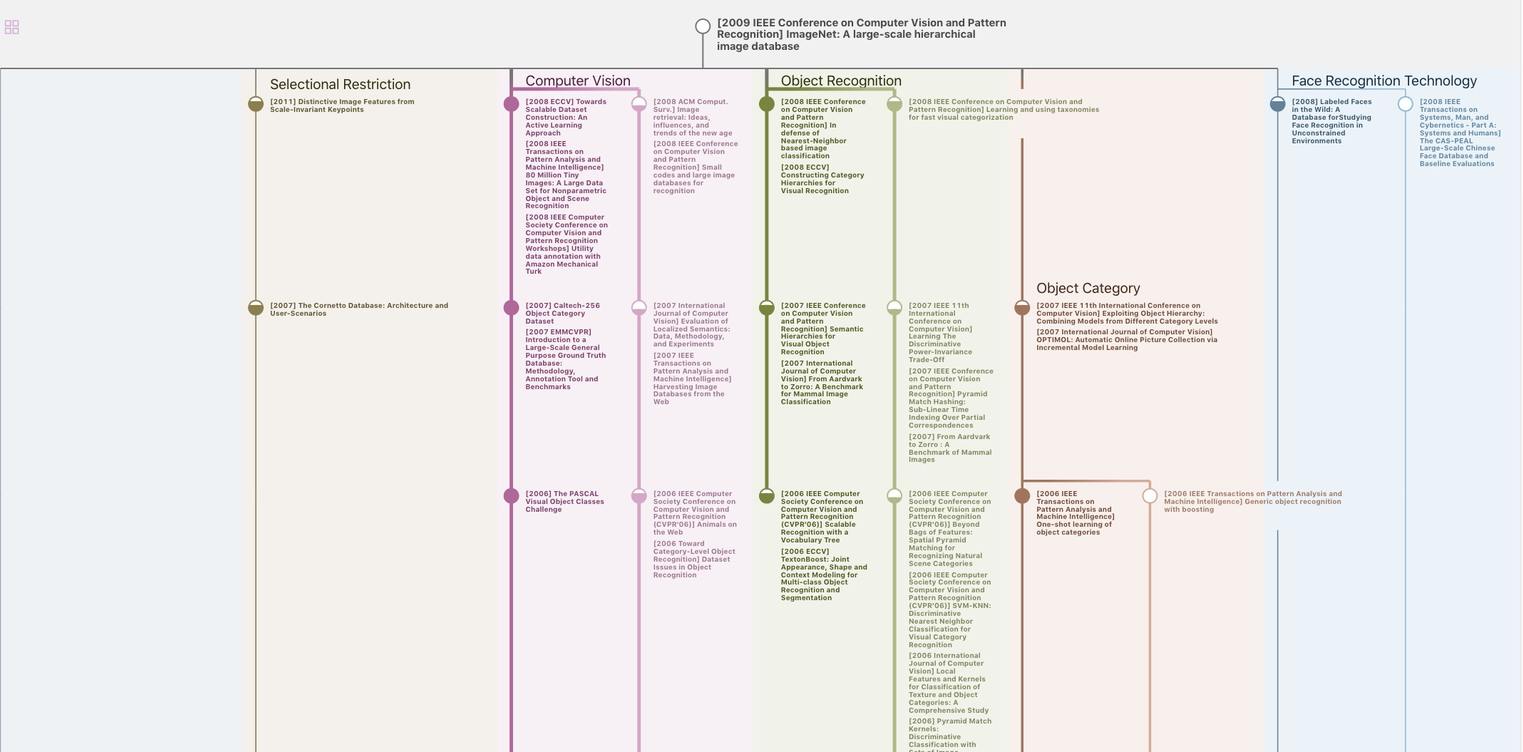
生成溯源树,研究论文发展脉络
Chat Paper
正在生成论文摘要