Improving Accuracy of Key Information Acquisition for Social Media Text Summarization
2019 IEEE International Conferences on Ubiquitous Computing & Communications (IUCC) and Data Science and Computational Intelligence (DSCI) and Smart Computing, Networking and Services (SmartCNS)(2019)
摘要
The attention-based sequence-to-sequence (Seq2Seq) model for abstractive summarization has achieved good performance, but it still suffers from generating inaccurate information and repetitive content when applied to noisy social media text. To address these issues, we propose a novel model that includes a feature extractor and a semantic enhancer. In our model, the feature extractor produces feature vectors that contain key information, filtering out noise in the source text. The semantic enhancer takes the concatenation of the feature vectors and the encoder hidden states as input to learn long-range dependencies, further improving semantic relevance and reducing repetition. Experimental results on a Chinese social media dataset show that our model can capture the key information from noisy text and achieve improvement as compared to state-of-the-art baseline models.
更多查看译文
关键词
feature extractor, semantic enhancer, text summarization, Seq2Seq
AI 理解论文
溯源树
样例
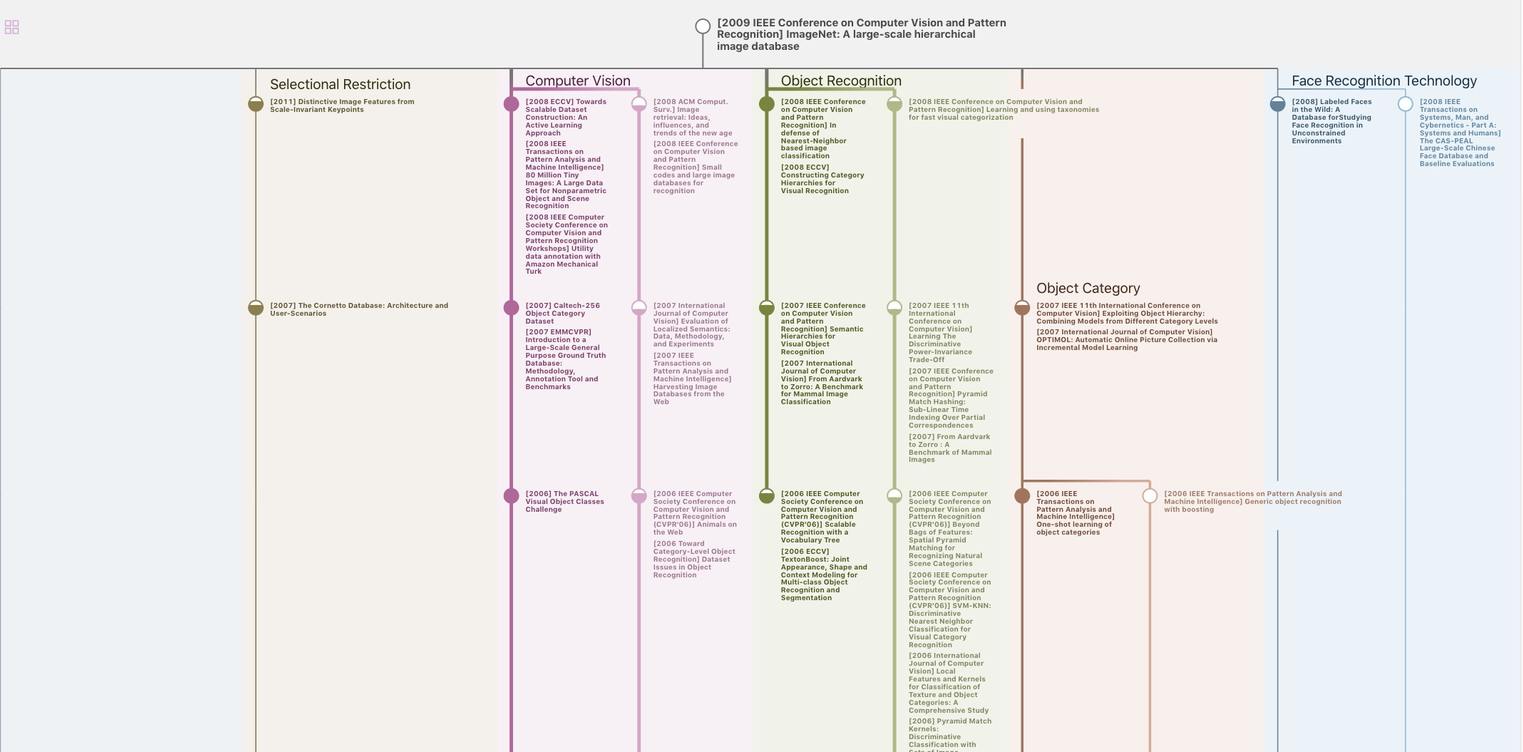
生成溯源树,研究论文发展脉络
Chat Paper
正在生成论文摘要