Unsupervised Learning of Category-Specific Symmetric 3D Keypoints from Point Sets
European Conference on Computer Vision(2020)
摘要
Automatic discovery of category-specific 3D keypoints from a collection of objects of a category is a challenging problem. The difficulty is added when objects are represented by 3D point clouds, with variations in shape and semantic parts and unknown coordinate frames. We define keypoints to be category-specific, if they meaningfully represent objects’ shape and their correspondences can be simply established order-wise across all objects. This paper aims at learning such 3D keypoints, in an unsupervised manner, using a collection of misaligned 3D point clouds of objects from an unknown category. In order to do so, we model shapes defined by the keypoints, within a category, using the symmetric linear basis shapes without assuming the plane of symmetry to be known. The usage of symmetry prior leads us to learn stable keypoints suitable for higher misalignments. To the best of our knowledge, this is the first work on learning such keypoints directly from 3D point clouds for a general category. Using objects from four benchmark datasets, we demonstrate the quality of our learned keypoints by quantitative and qualitative evaluations. Our experiments also show that the keypoints discovered by our method are geometrically and semantically consistent.
更多查看译文
关键词
keypoints,3d,learning,keypoints,category-specific
AI 理解论文
溯源树
样例
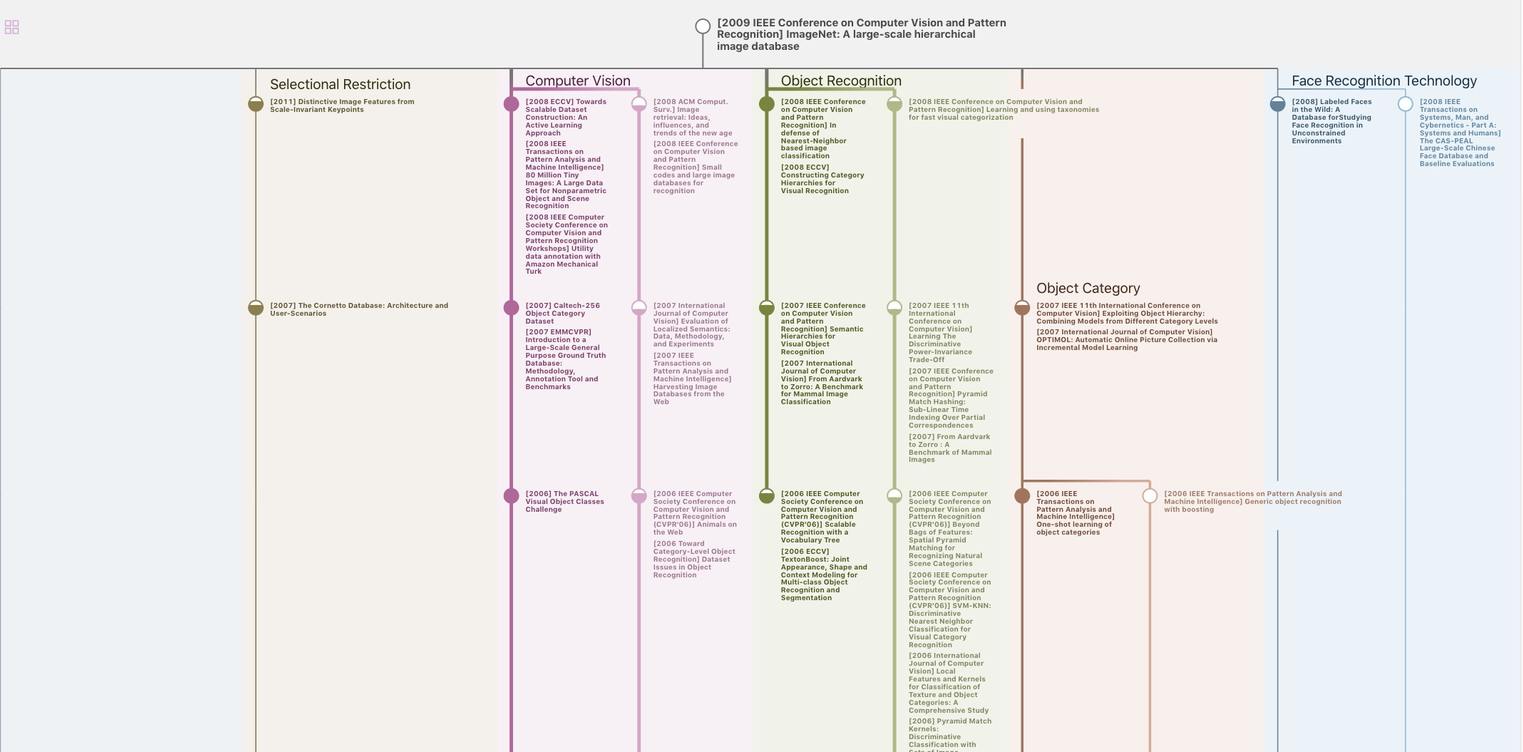
生成溯源树,研究论文发展脉络
Chat Paper
正在生成论文摘要