Is Temporal Difference Learning Optimal? An Instance-Dependent Analysis
SIAM JOURNAL ON MATHEMATICS OF DATA SCIENCE(2021)
摘要
We address the problem of policy evaluation in discounted, tabular Markov decision processes and provide instance-dependent guarantees on the l(infinity)-error under a generative model. We establish both asymptotic and nonasymptotic versions of local minimax lower bounds for policy evaluation, thereby providing an instance-dependent baseline by which to compare algorithms. Theory-inspired simulations show that the widely used temporal difference (TD) algorithm is strictly suboptimal when evaluated in a nonasymptotic setting, even when combined with Polyak-Ruppert iterate averaging. We remedy this issue by introducing and analyzing variance-reduced forms of stochastic approximation, showing that they achieve nonasymptotic, instance-dependent optimality up to logarithmic factors.
更多查看译文
关键词
temporal difference learning, Polyak-Ruppert averaging, variance reduction
AI 理解论文
溯源树
样例
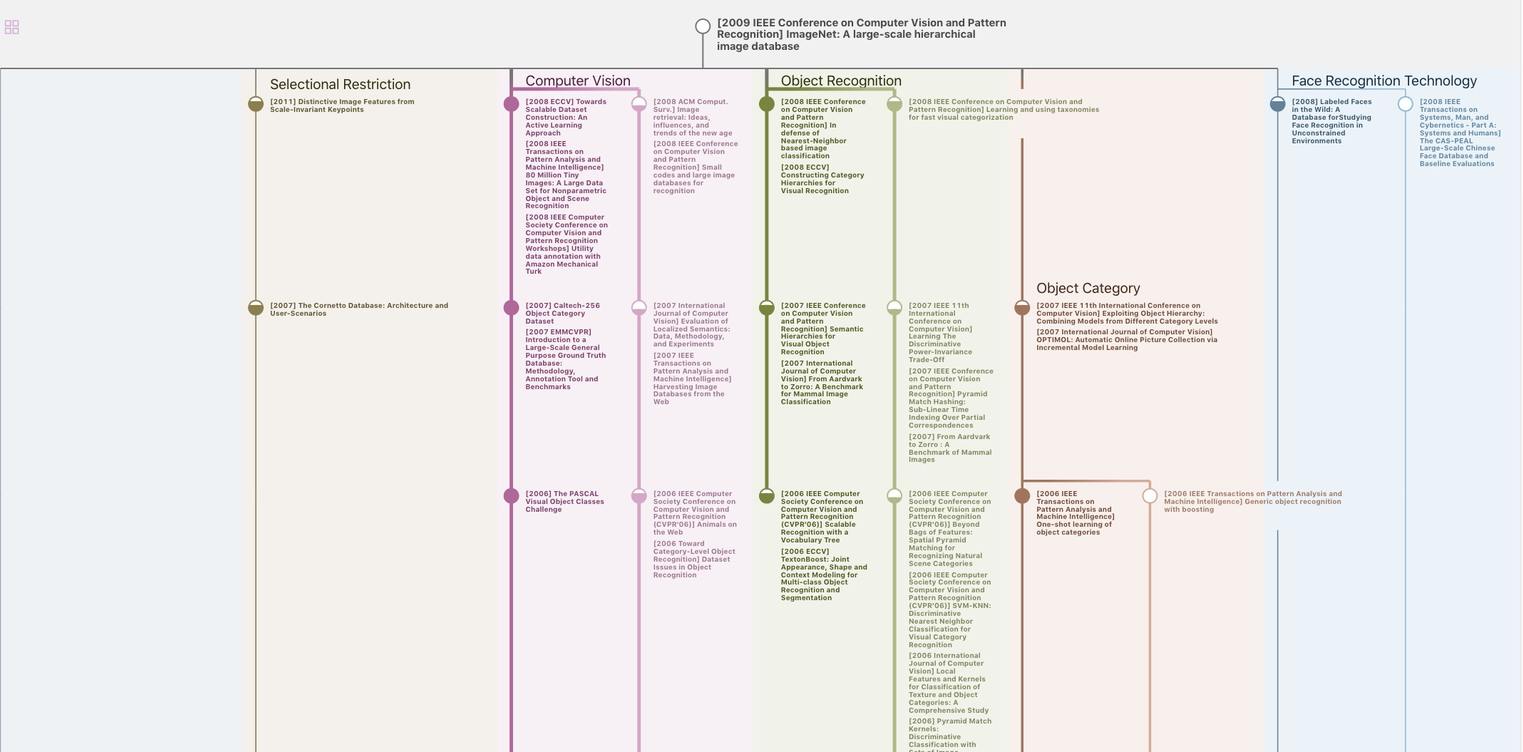
生成溯源树,研究论文发展脉络
Chat Paper
正在生成论文摘要