Night-Time Scene Parsing With a Large Real Dataset
IEEE TRANSACTIONS ON IMAGE PROCESSING(2021)
摘要
Although huge progress has been made on scene analysis in recent years, most existing works assume the input images to be in day-time with good lighting conditions. In this work, we aim to address the night-time scene parsing (NTSP) problem, which has two main challenges: 1) labeled night-time data are scarce, and 2) over- and under-exposures may co-occur in the input night-time images and are not explicitly modeled in existing pipelines. To tackle the scarcity of night-time data, we collect a novel labeled dataset, named NightCity, of 4,297 real night-time images with ground truth pixel-level semantic annotations. To our knowledge, NightCity is the largest dataset for NTSP. In addition, we also propose an exposure-aware framework to address the NTSP problem through augmenting the segmentation process with explicitly learned exposure features. Extensive experiments show that training on NightCity can significantly improve NTSP performances and that our exposure-aware model outperforms the state-of-the-art methods, yielding top performances on our dataset as well as existing datasets.
更多查看译文
关键词
Streaming media, Urban areas, Image segmentation, Annotations, Semantics, Computer science, Automobiles, Autonomous driving, night-time vision, scene analysis, adverse conditions
AI 理解论文
溯源树
样例
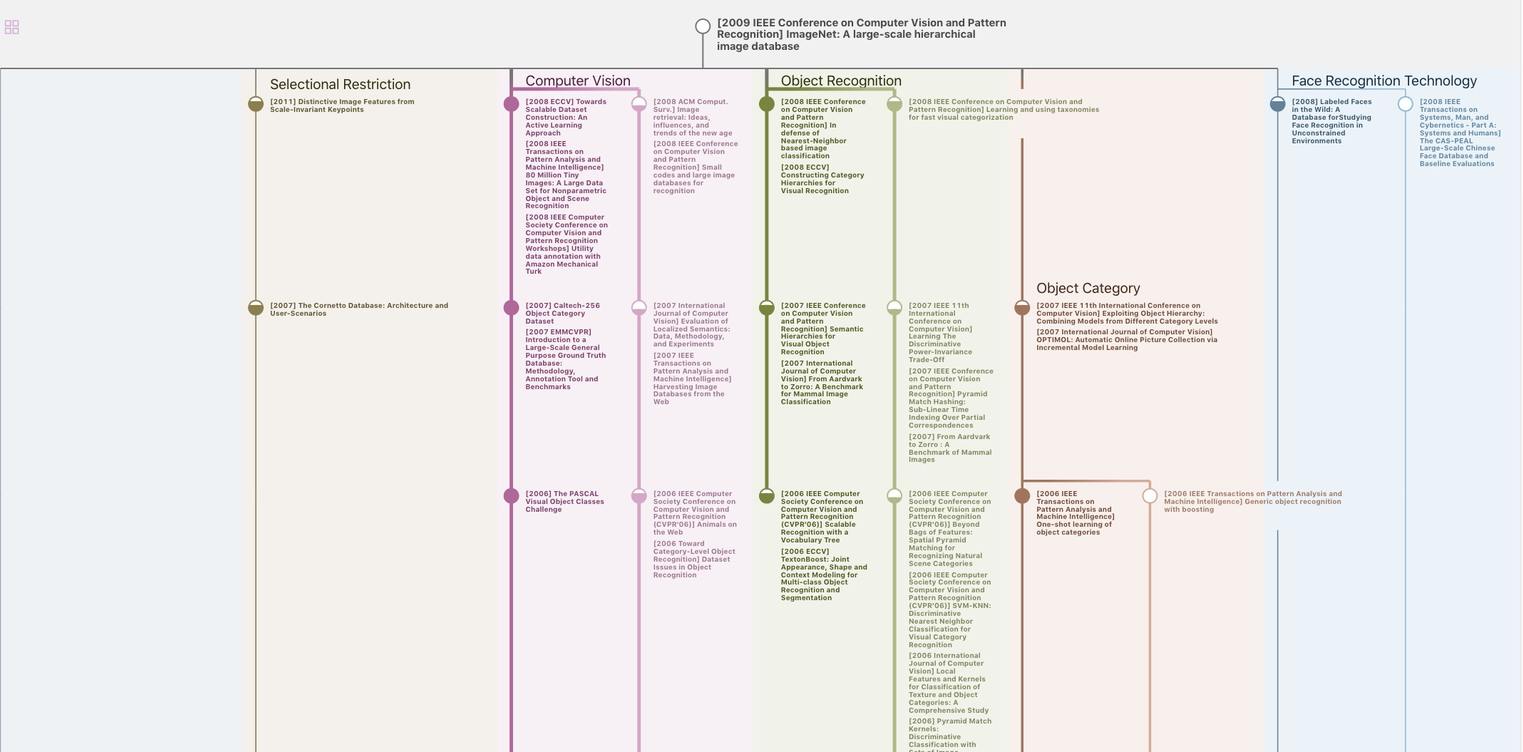
生成溯源树,研究论文发展脉络
Chat Paper
正在生成论文摘要