Risk-Aversion Adjusted Portfolio Optimization With Predictive Modeling
2019 22ND INTERNATIONAL CONFERENCE ON INFORMATION FUSION (FUSION 2019)(2019)
摘要
We propose a multi-period portfolio optimization method rooted on the mean-risk framework, in which the risk aversion coefficient is adjusted in response to the market trend movement predicted by machine learning models. We use the Gini's Mean Difference to characterize the risk of the portfolio, and employ a series of technical indicators as the features to feed the predictive machine learning models. A set of comprehensive computational tests are carried out within a rolling-horizon approach to evaluate the performance of the generated portfolios. The empirical results show that the regularized logistic regression model provides the best prediction of market trend movement, while the proposed dynamic risk-aversion adjusted portfolio rebalancing strategy generates portfolios with higher time-series cumulative returns than a static strategy with fixed risk-aversion coefficient.
更多查看译文
关键词
Portfolio optimization, predictive modeling, technical indicators, logistic LASSO regression, risk-aversion coefficient, information fusion
AI 理解论文
溯源树
样例
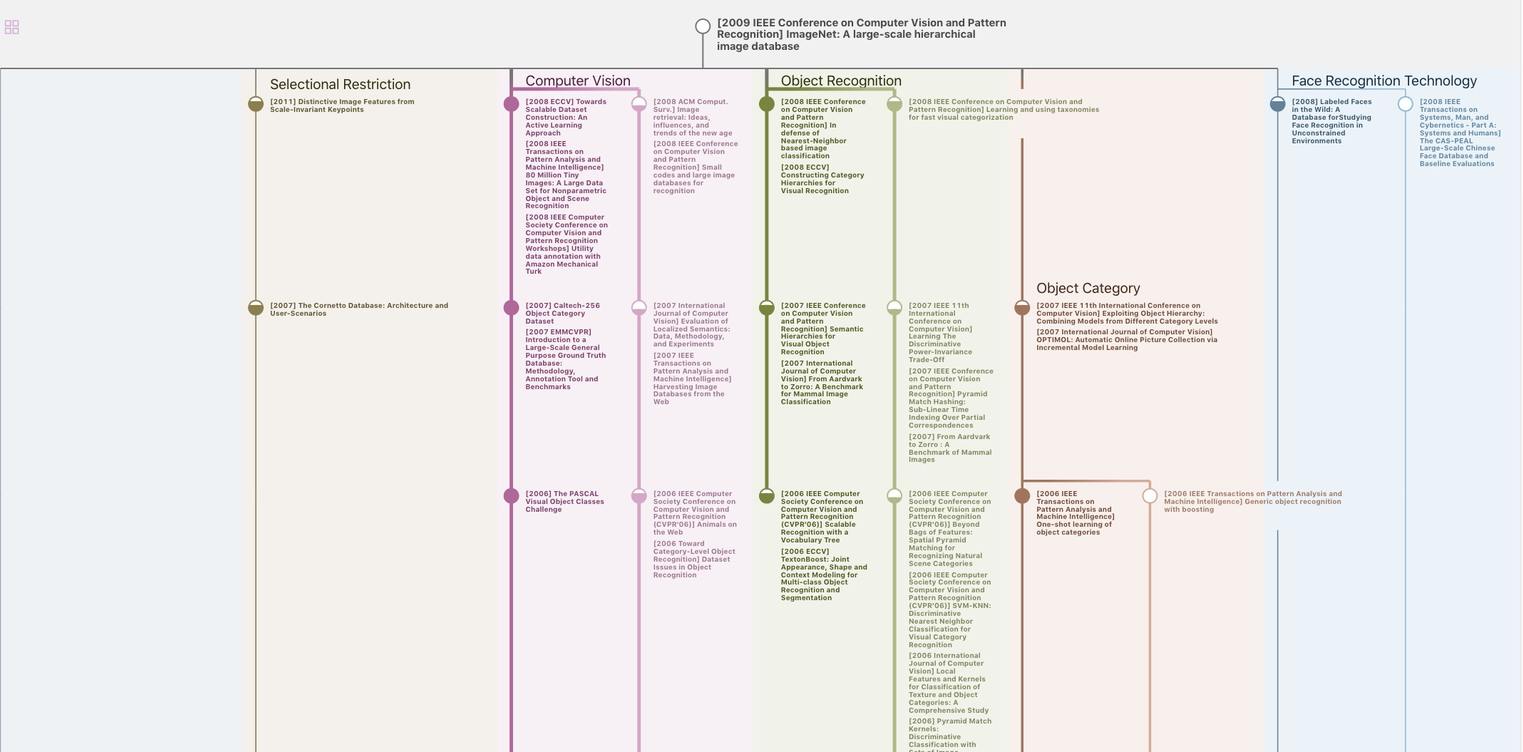
生成溯源树,研究论文发展脉络
Chat Paper
正在生成论文摘要