Bootstrapping promotes the RSFC-behavior associations: An application of individual cognitive traits prediction.
HUMAN BRAIN MAPPING(2020)
摘要
Resting-state functional connectivity (RSFC) records enormous functional interaction information between any pair of brain nodes, which enriches the individual-phenotypic prediction. To reduce high-dimensional features, correlation analysis is a common way for feature selection. However, resting state fMRI signal exhibits typically low signal-to-noise ratio and the correlation analysis is sensitive to outliers and data distribution, which may bring unstable features to prediction. To alleviate this problem, a bootstrapping-based feature selection framework was proposed and applied to connectome-based predictive modeling, support vector regression, least absolute shrinkage and selection operator, and Ridge regression to predict a series of cognitive traits based on Human Connectome Project data. To systematically investigate the influences of different parameter settings on the bootstrapping-based framework, 216 parameter combinations were evaluated and the best performance among them was identified as the final prediction result for each cognitive trait. By using the bootstrapping methods, the best prediction performances outperformed the baseline method in all four prediction models. Furthermore, the proposed framework could effectively reduce the feature dimension by retaining the more stable features. The results demonstrate that the proposed framework is an easy-to-use and effective method to improve RSFC prediction of cognitive traits and is highly recommended in future RSFC-prediction studies.
更多查看译文
关键词
bootstrapping,cognitive trait,feature selection,functional connectivity,individualized prediction
AI 理解论文
溯源树
样例
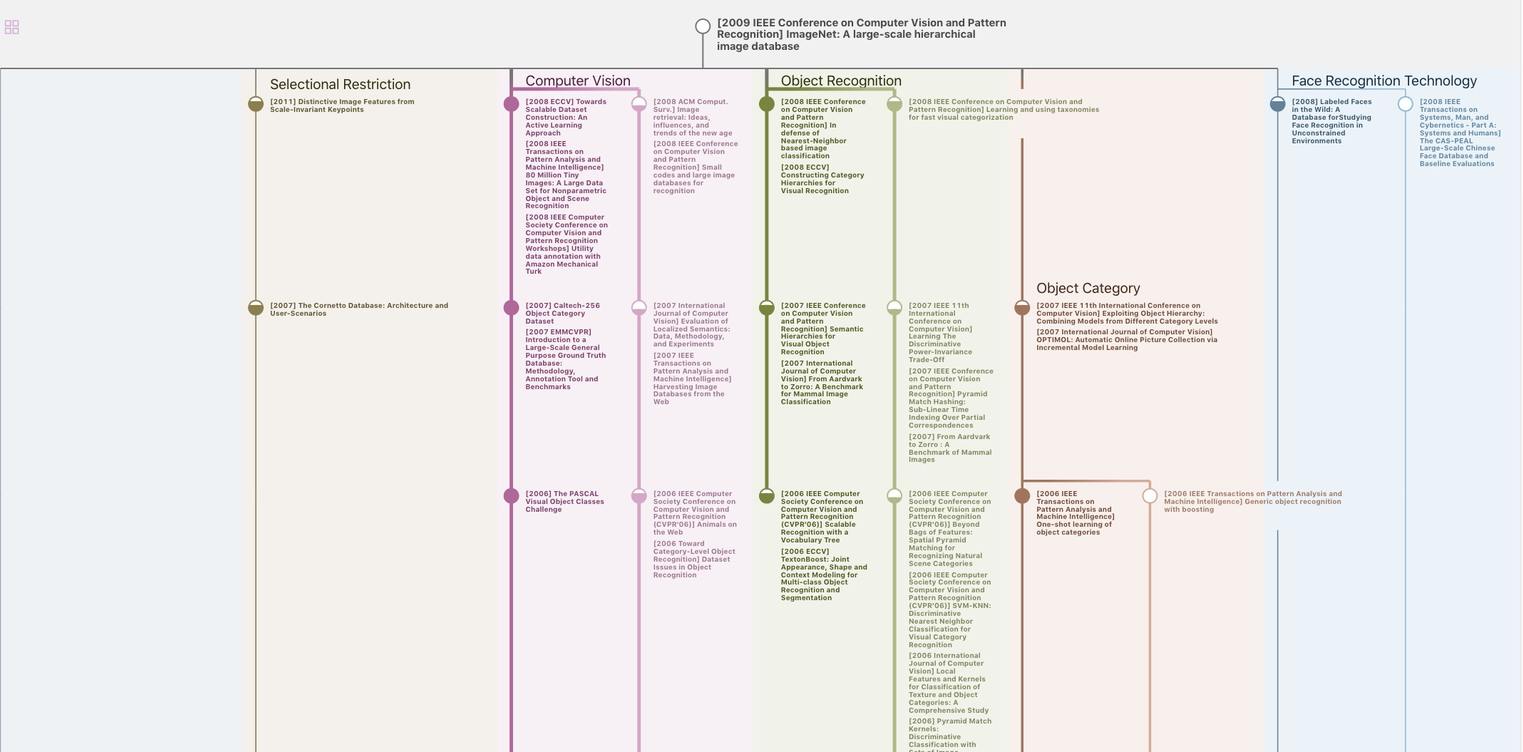
生成溯源树,研究论文发展脉络
Chat Paper
正在生成论文摘要