A Multi-Target Track-Before-Detect Particle Filter Using Superpositional Data In Non-Gaussian Noise
IEEE SIGNAL PROCESSING LETTERS(2020)
摘要
We propose a particle filter (PF) for tracking time-varying states (e.g., position, velocity) of multiple targets jointly from superpositional data, which depend on the sum of all target signals. Many conventional methods perform thresholding for detection prior to tracking, which severely limits tracking performance at a low signal-to-noise ratio. In contrast, the proposed PF can operate directly on unthresholded sensor signals. Though there also exist methods applicable to unthresholded sensor signals called track-before-detect (TBD), the proposed PF has significant advantages over them. First, it is general without any restrictions on the form of a function that maps each target's states to its signal (e.g., disjoint, binary) or on the statistics of observation noise (e.g., Gaussian). Second, it can track an unknown, time-varying number of targets without knowing their initial states owing to Septier et al.'s state modeling with a birth/death process. The proposed PF includes Salmond et al.'s TBD PF for at most one target as a particular instance up to some implementation details. We present a simulation example in the context of radio-frequency tomography, where the proposed PF significantly outperformed Nannuru et al.'s state-of-the-art method based on random finite sets in terms of the optimal subpattern assignment (OSPA) metric.
更多查看译文
关键词
Target tracking, Signal to noise ratio, Particle filters, Radio frequency, Indexes, Signal processing algorithms, Indium tin oxide, Multi-target tracking, track-before-detect, particle filter, superpositional sensor signals
AI 理解论文
溯源树
样例
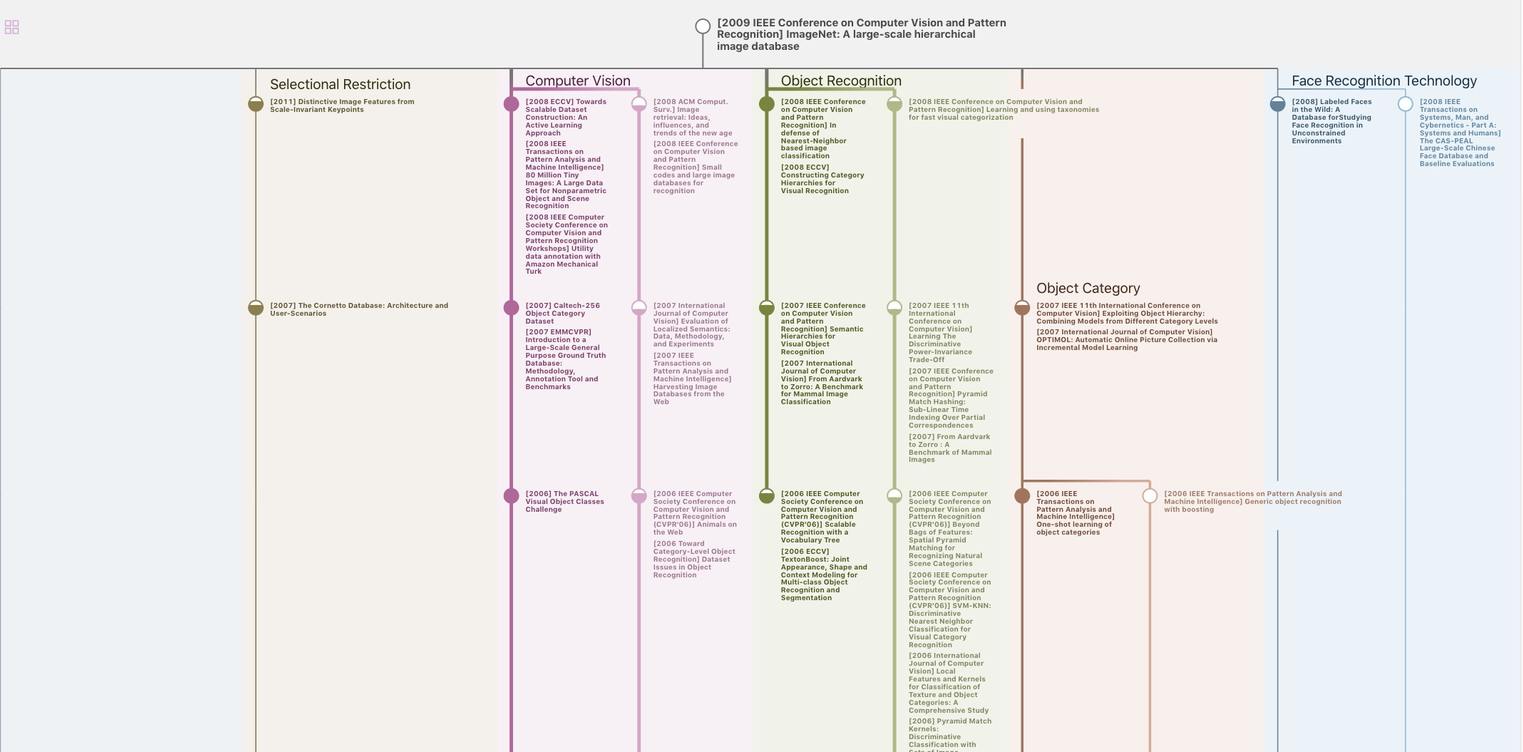
生成溯源树,研究论文发展脉络
Chat Paper
正在生成论文摘要