A Learning-Based Bandwidth Resource Allocation Method in Sliced 5G C-RAN
2019 IEEE Globecom Workshops (GC Wkshps)(2019)
摘要
In the fifth generation (5G) network, the Cloud Radio Access Network (C-RAN) architecture is considered as a promising technology. In the typical C-RAN network, the base station is decoupled into Baseband Unit (BBU) and Remote Radio Head (RRH). In order to achieve higher flexibility and better resource utilization ratio, the BBU is further split into Centralized Unit (CU) and Distributed Unit (DU) in the 5G C-RAN architecture. Moreover, as the 5G network needs to support various type of services, network slicing is employed to meet the multiple Quality of Service (QoS) requirements of different services. Due to the limited bandwidth of the link between the CU and DU, which is also known as fronthaul-II, it is still a challenge to satisfy the needs of different services simultaneously in sliced 5G C-RAN. We propose a learning based resource allocation scheme to manage the limited fronthaul-II bandwidth and optimize the QoS for multiple services with the same physical network infrastructure. The simulation results indicate that the learning based scheme can achieve higher QoS, lower packet loss rate and also better bandwidth utilization ratio comparing with some typical policy-based allocation methods.
更多查看译文
关键词
Cloud Radio Access Network architecture,typical C-RAN network,base station,Baseband Unit,BBU,Remote Radio Head,resource utilization ratio,Centralized Unit,Distributed Unit,DU,network slicing,quality of service requirements,learning based resource allocation scheme,fronthaul-II bandwidth,physical network infrastructure,learning based scheme,bandwidth utilization ratio,learning-based bandwidth resource allocation method,fifth generation network,5G,policy-based allocation methods,sliced 5G C-RAN
AI 理解论文
溯源树
样例
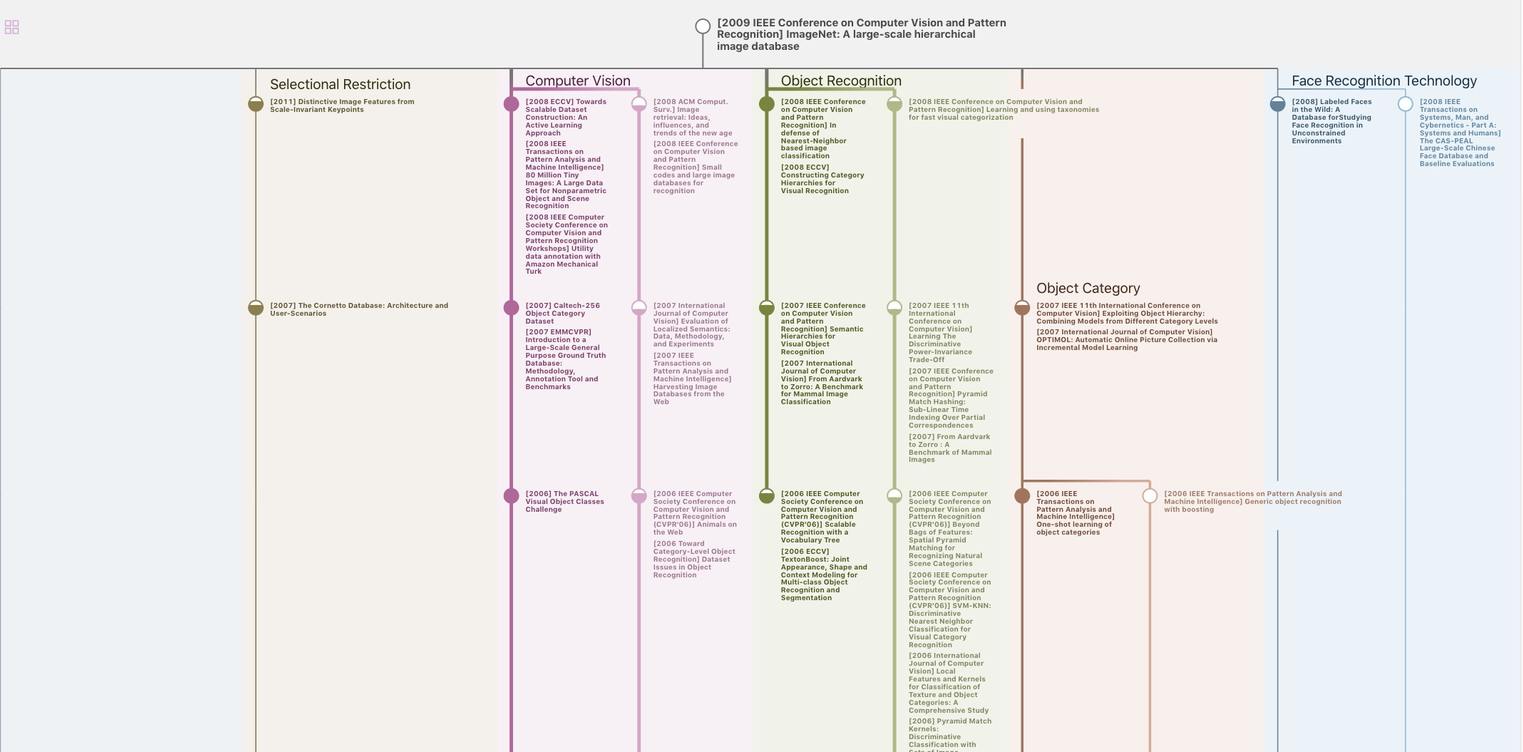
生成溯源树,研究论文发展脉络
Chat Paper
正在生成论文摘要