Probabilistic PCA for Heteroscedastic Data
2019 IEEE 8th International Workshop on Computational Advances in Multi-Sensor Adaptive Processing (CAMSAP)(2019)
摘要
Principal Component Analysis (PCA) is a standard dimensionality reduction technique, but it treats all samples uniformly' making it suboptimal for heterogeneous data that are increasingly common in modern settings. This paper proposes a PCA variant for samples with heterogeneous noise levels, i.e., heteroscedastic noise, that naturally arise when some of the data come from higher quality sources than others. The technique handles heteroscedasticity by incorporating it in the statistical model of a probabilistic PCA. The resulting optimization problem is an interesting nonconvex problem related to but not seemingly solved by singular value decomposition, and this paper derives an expectation maximization (EM) algorithm. Numerical experiments illustrate the benefits of using the proposed method to combine samples with heteroscedastic noise in a single analysis, as well as benefits of careful initialization for the EM algorithm.
更多查看译文
关键词
Principal component analysis,heterogeneous data,maximum likelihood estimation,latent factors
AI 理解论文
溯源树
样例
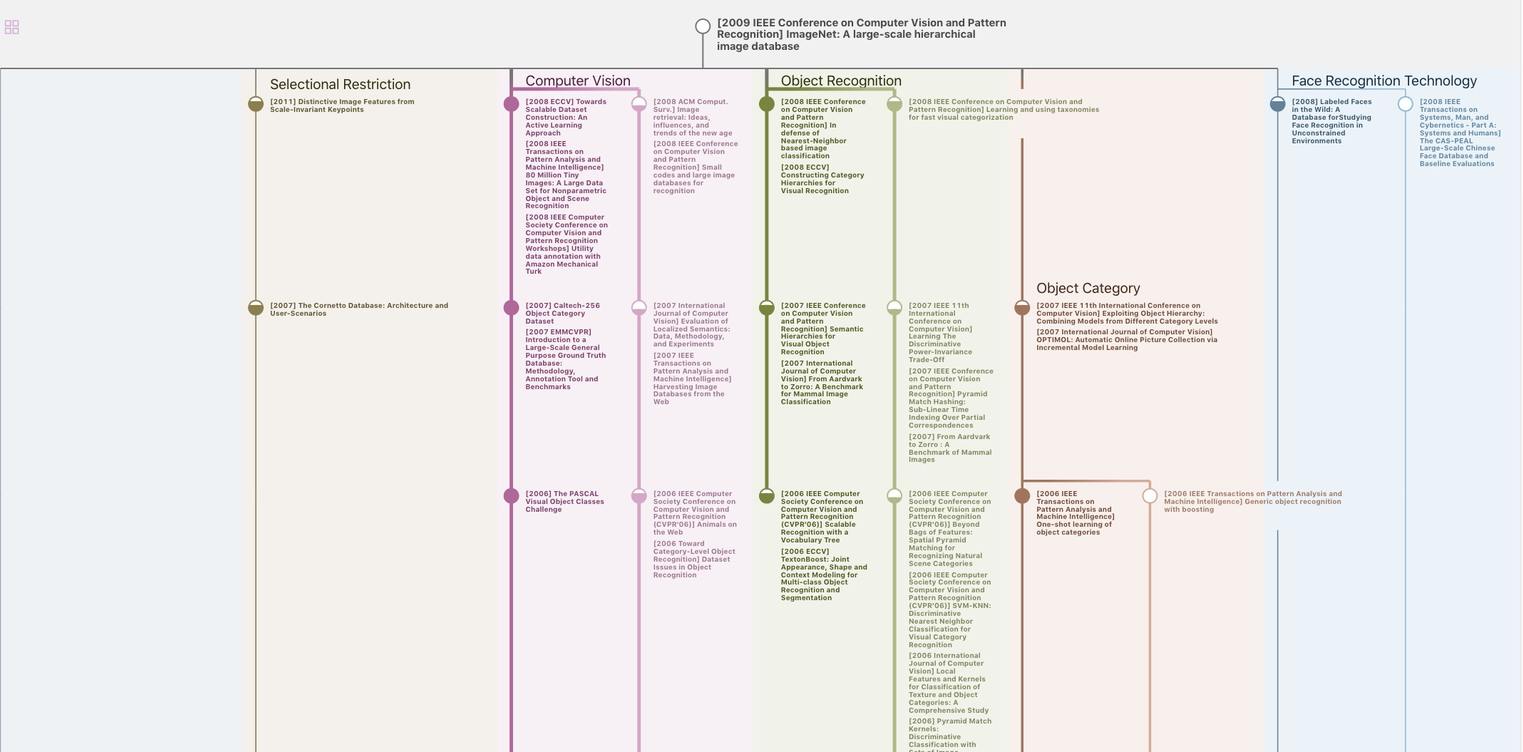
生成溯源树,研究论文发展脉络
Chat Paper
正在生成论文摘要