A Super-Resolution Algorithm for Extended Target Localization
2019 IEEE 8th International Workshop on Computational Advances in Multi-Sensor Adaptive Processing (CAMSAP)(2019)
摘要
We aim to localize extended targets (i.e. non-point targets) using stepped-frequency (SF) radar measurements from multiple transceivers. In particular, we consider a general signal model with directionally varying target reflectivity that can account for many types of extended target geometries. We localize targets under this signal model using a novel convex relaxation of a tensor atomic norm minimization problem that leverages discrete prolate spheroidal sequences (DPSS's). We test this algorithm on simulated data and show that our proposed method outperforms alternatives for our considered signal model.
更多查看译文
关键词
Stepped Frequency (SF) radar,extended target localization,atomic norm minimization,blind spectral super-resolution,convex optimization
AI 理解论文
溯源树
样例
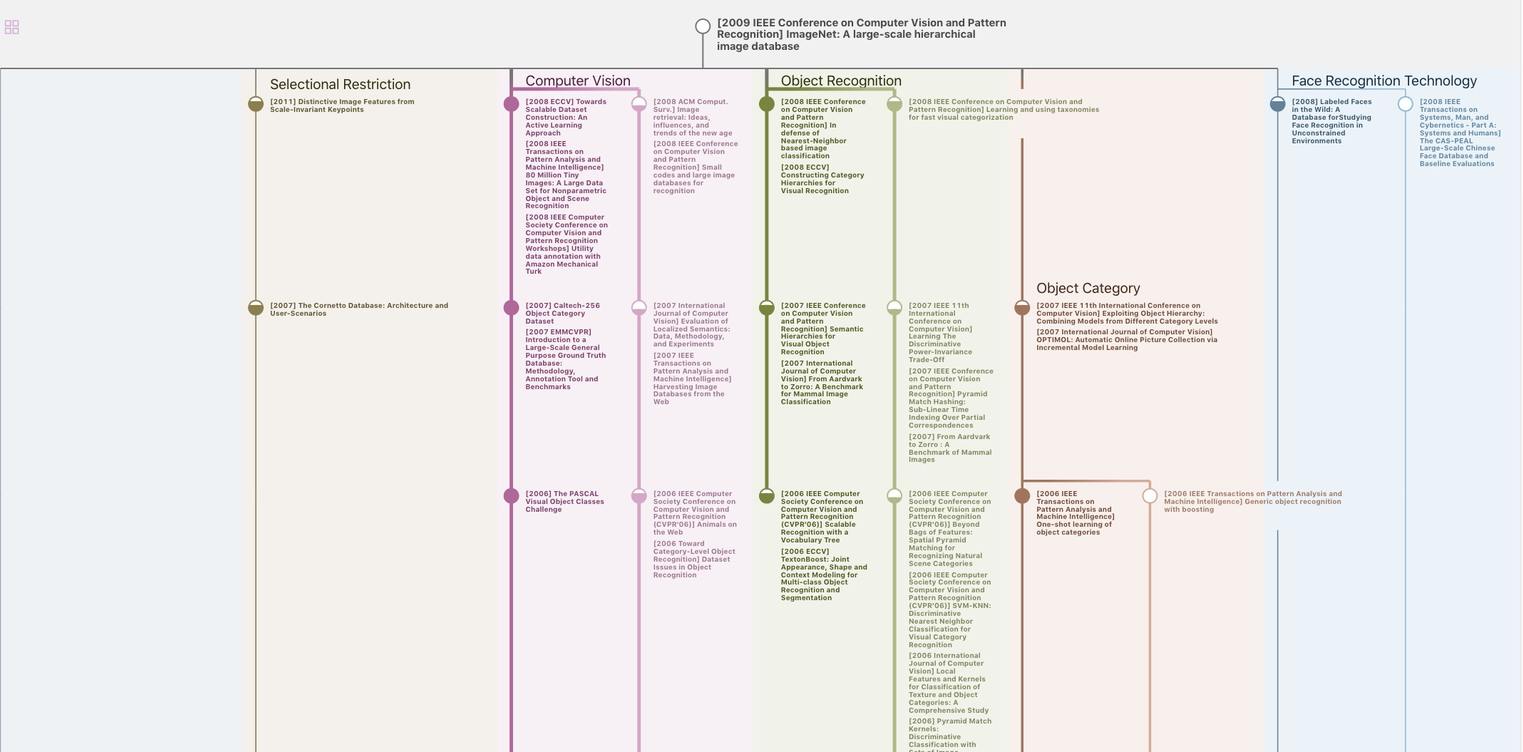
生成溯源树,研究论文发展脉络
Chat Paper
正在生成论文摘要