Salient Object Detection With Residual Refinement Network.
ICSAI(2019)
摘要
The salient object detection task has recently been further developed based on deep convolution neural networks. However, it's still challenging to extract effective features and obtain clear boundaries of the salient objects. An often-used way to enhance performance is to directly aggregate multilevel convolutional features. In this paper, We put forward a prediction-refinement architecture to detect salient regions. The saliency estimation network(E) integrates low-level structural feature and high-level context information to make estimations. While the high-level feature is capable of locating salient regions, the low-level feature can capture more detailed information to estimate salient pixels correctly. Furthermore, We bring forward a residual refinement network(R) with novel downsample and upsample layers to optimize the saliency prediction generated from E. R refines the coarse saliency detection results by learning the residual between the saliency prediction and ground-truth. The prediction-refinement architecture progressively generates saliency maps with high-quality boundaries. Experiments indicate that this method notably outperforms most previous saliency approaches.
更多查看译文
关键词
multilevel convolutional features,prediction-refinement architecture,saliency estimation network,low-level structural feature,high-level context information,residual refinement network,salient object detection task,deep convolution neural networks
AI 理解论文
溯源树
样例
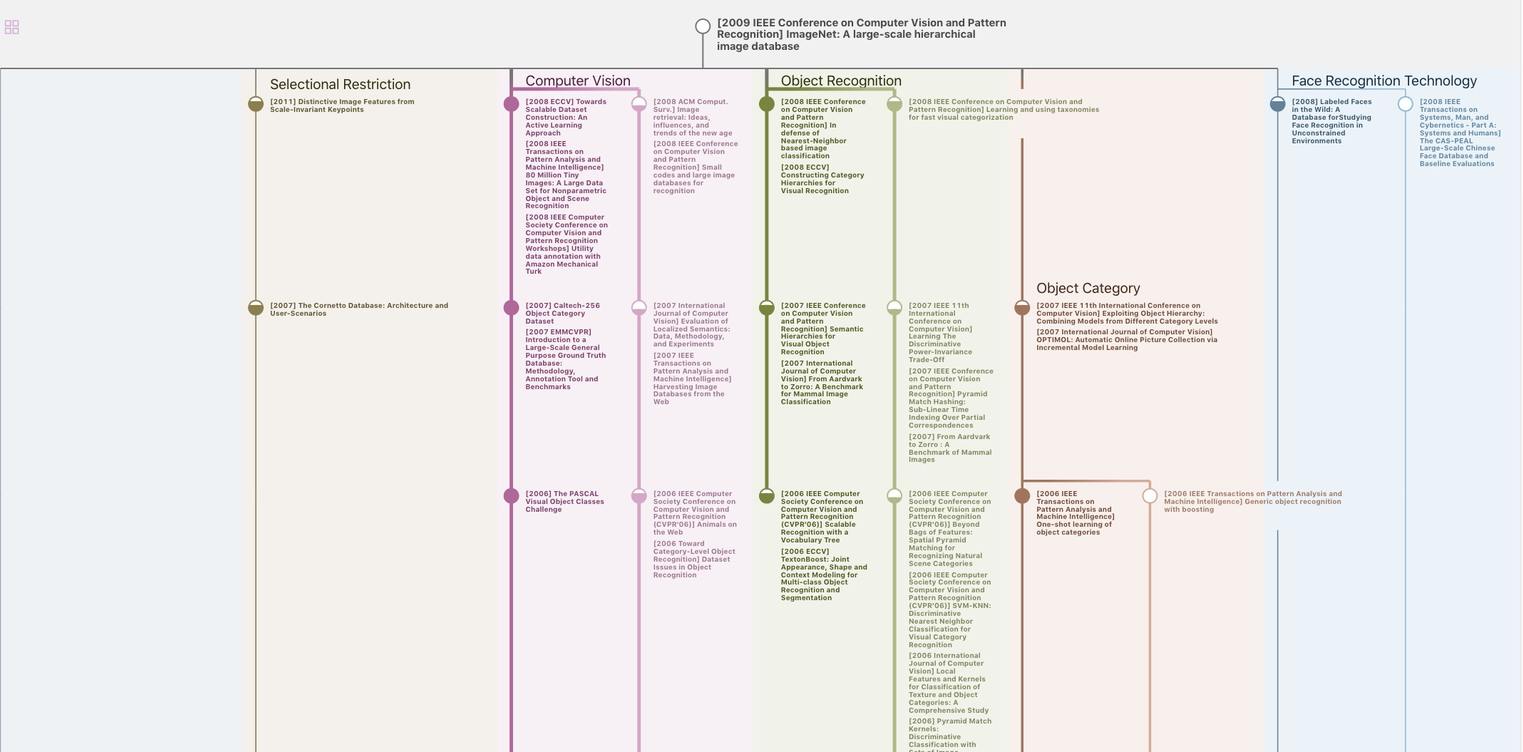
生成溯源树,研究论文发展脉络
Chat Paper
正在生成论文摘要