Graph-Based Non-Convex Low-Rank Regularization For Image Compression Artifact Reduction
IEEE TRANSACTIONS ON IMAGE PROCESSING(2020)
摘要
Block transform coded images usually suffer from annoying artifacts at low bit-rates, because of the independent quantization of DCT coefficients. Image prior models play an important role in compressed image reconstruction. Natural image patches in a small neighborhood of the high-dimensional image space usually exhibit an underlying sub-manifold structure. To model the distribution of signal, we extract sub-manifold structure as prior knowledge. We utilize graph Laplacian regularization to characterize the sub-manifold structure at patch level. And similar patches are exploited as samples to estimate distribution of a particular patch. Instead of using Euclidean distance as similarity metric, we propose to use graph-domain distance to measure the patch similarity. Then we perform low-rank regularization on the similar-patch group, and incorporate a non-convex l(p) penalty to surrogate matrix rank. Finally, an alternatively minimizing strategy is employed to solve the non-convex problem. Experimental results show that our proposed method is capable of achieving more accurate reconstruction than the state-of-the-art methods in both objective and perceptual qualities.
更多查看译文
关键词
Low-rank model, graph Laplacian regularization, manifold structure, non-convex, compression artifact reduction
AI 理解论文
溯源树
样例
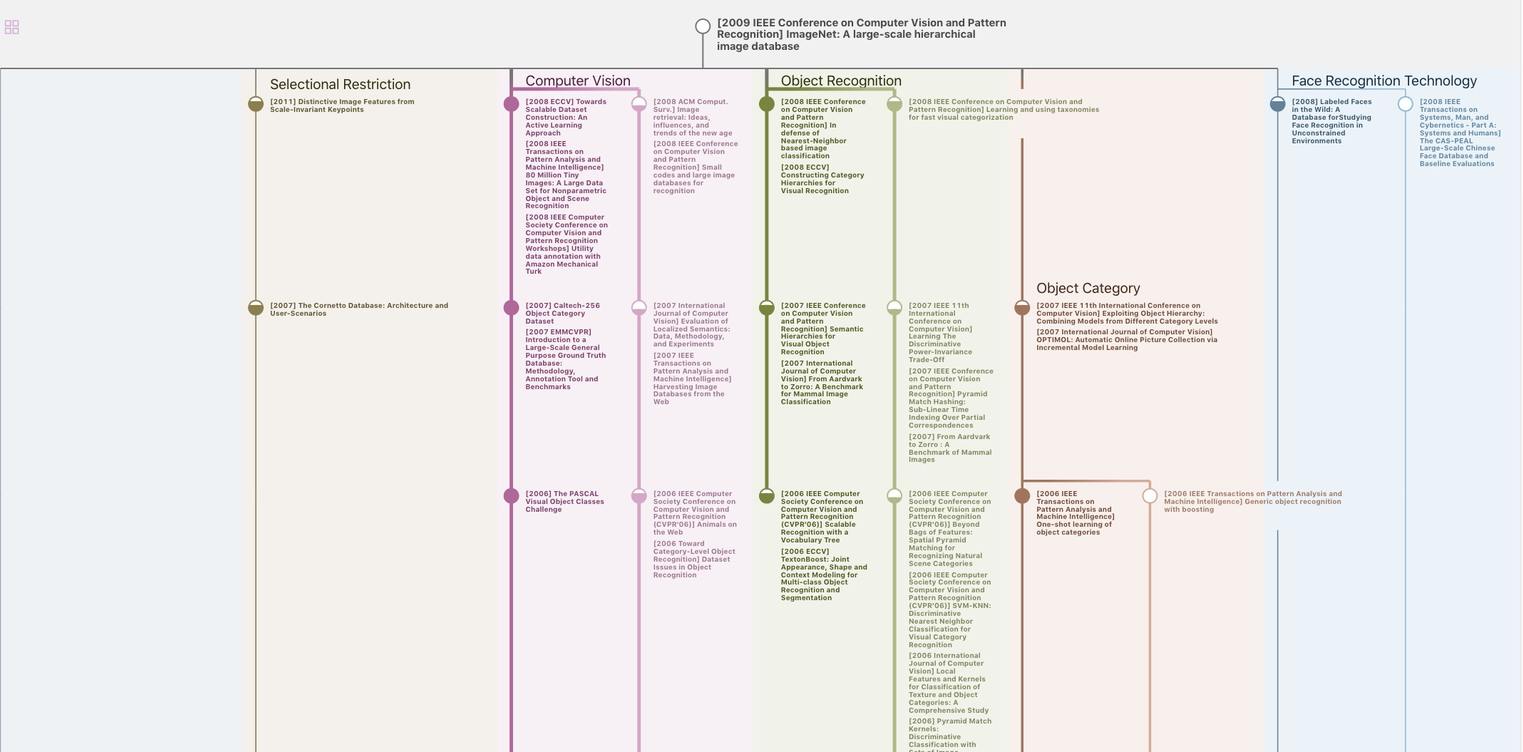
生成溯源树,研究论文发展脉络
Chat Paper
正在生成论文摘要