Color Image Demosaicing Using Progressive Collaborative Representation
IEEE TRANSACTIONS ON IMAGE PROCESSING(2020)
摘要
In this paper, a progressive collaborative representation (PCR) framework is proposed that is able to incorporate any existing color image demosaicing method for further boosting its demosaicing performance. Our PCR consists of two phases: (i) offline training and (ii) online refinement. In phase (i), multiple training-and-refining stages will be performed. In each stage, a new dictionary will be established through the learning of a large number of feature-patch pairs, extracted from the demosaicked images of the current stage and their corresponding original full-color images. After training, a projection matrix will be generated and exploited to refine the current demosaicked image. The updated image with improved image quality will be used as the input for the next training-and-refining stage and performed the same processing likewise. At the end of phase (i), all the projection matrices generated as above-mentioned will be exploited in phase (ii) to conduct online demosaicked image refinement of the test image. Extensive simulations conducted on two commonly-used test datasets (i.e., IMAX and Kodak) for evaluating the demosaicing algorithms have clearly demonstrated that our proposed PCR framework is able to constantly boost the performance of any image demosaicing method we experimented, in terms of objective and subjective performance evaluations.
更多查看译文
关键词
Training, Image color analysis, Collaboration, Rough surfaces, Color, Feature extraction, Image quality, Image demosaicing, color filter array (CFA), residual interpolation, progressive collaborative representation
AI 理解论文
溯源树
样例
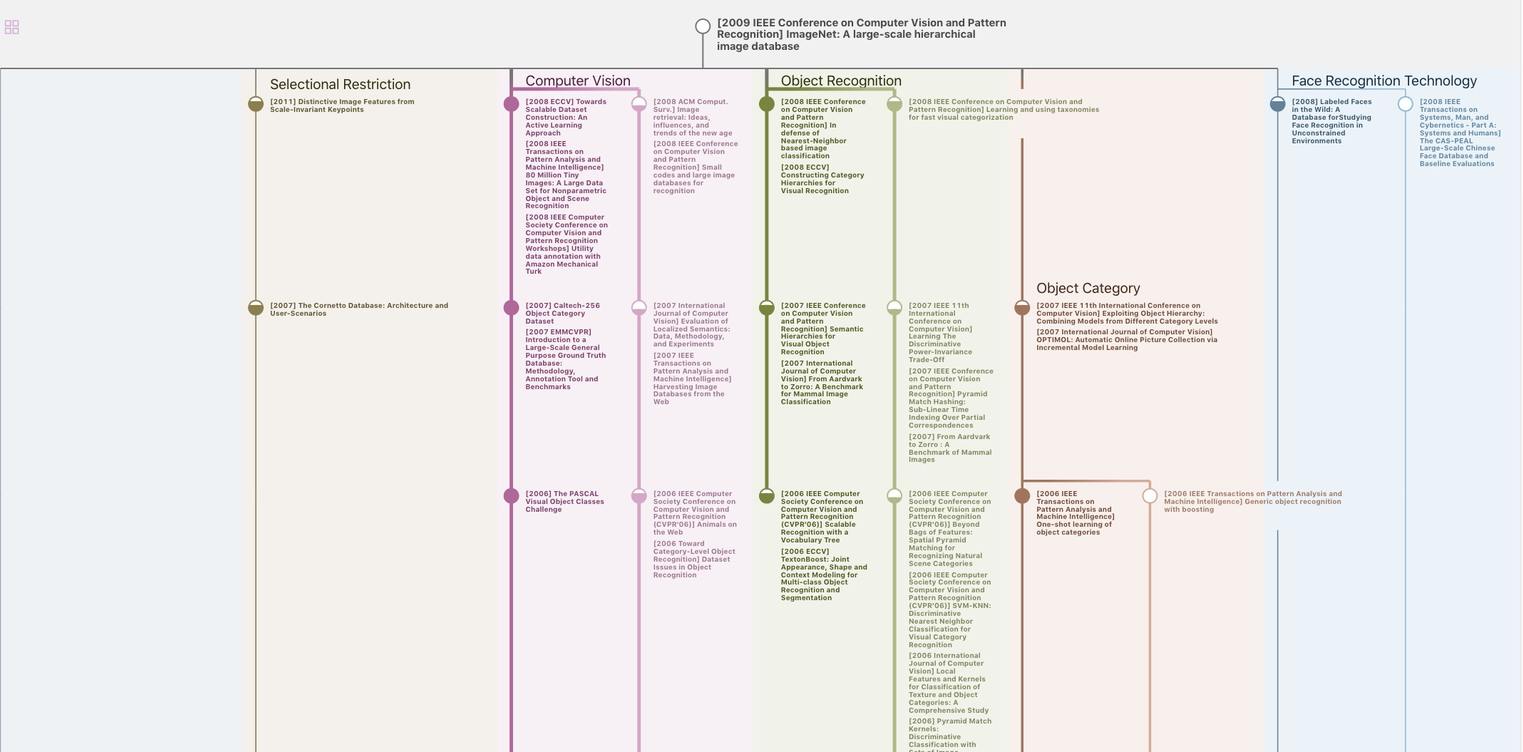
生成溯源树,研究论文发展脉络
Chat Paper
正在生成论文摘要