Zooming for Efficient Model-Free Reinforcement Learning in Metric Spaces
arxiv(2020)
摘要
Despite the wealth of research into provably efficient reinforcement learning algorithms, most works focus on tabular representation and thus struggle to handle exponentially or infinitely large state-action spaces. In this paper, we consider episodic reinforcement learning with a continuous state-action space which is assumed to be equipped with a natural metric that characterizes the proximity between different states and actions. We propose ZoomRL, an online algorithm that leverages ideas from continuous bandits to learn an adaptive discretization of the joint space by zooming in more promising and frequently visited regions while carefully balancing the exploitation-exploration trade-off. We show that ZoomRL achieves a worst-case regret $\tilde{O}(H^{\frac{5}{2}} K^{\frac{d+1}{d+2}})$ where $H$ is the planning horizon, $K$ is the number of episodes and $d$ is the covering dimension of the space with respect to the metric. Moreover, our algorithm enjoys improved metric-dependent guarantees that reflect the geometry of the underlying space. Finally, we show that our algorithm is robust to small misspecification errors.
更多查看译文
关键词
learning,metric,model-free
AI 理解论文
溯源树
样例
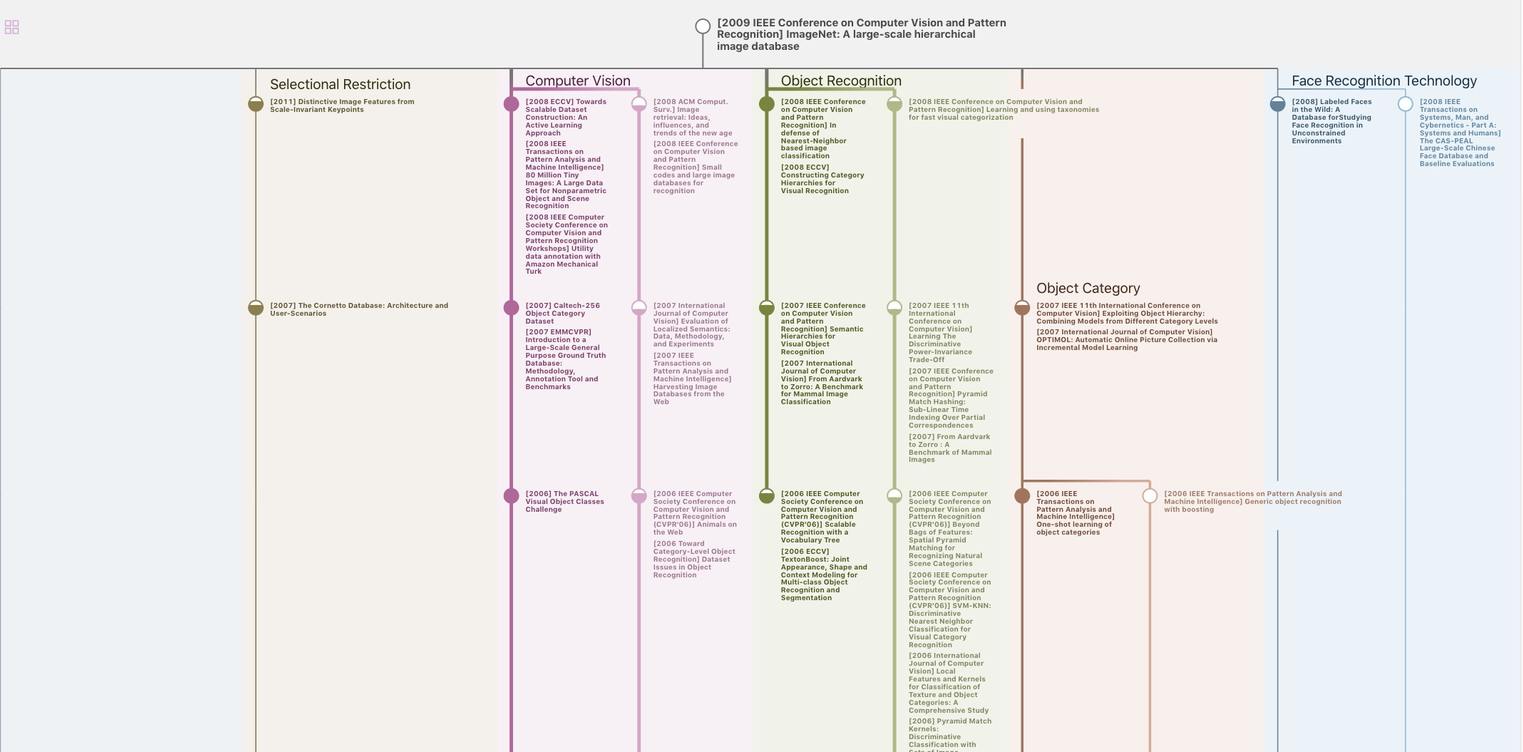
生成溯源树,研究论文发展脉络
Chat Paper
正在生成论文摘要