Deconfounded Image Captioning: A Causal Retrospect
IEEE transactions on pattern analysis and machine intelligence(2023)
摘要
Dataset bias in vision-language tasks is becoming one of the main problems which hinders the progress of our community. Existing solutions lack a principled analysis about why modern image captioners easily collapse into dataset bias. In this paper, we present a novel perspective: Deconfounded Image Captioning (DIC), to find out the answer of this question, then retrospect modern neural image captioners, and finally propose a DIC framework: DICv1.0 to alleviate the negative effects brought by dataset bias. DIC is based on causal inference, whose two principles: the backdoor and front-door adjustments, help us review previous studies and design new effective models. In particular, we showcase that DICv1.0 can strengthen two prevailing captioning models and can achieve a single-model 131.1 CIDEr-D and 128.4 c40 CIDEr-D on Karpathy split and online split of the challenging MS COCO dataset, respectively. Interestingly, DICv1.0 is a natural derivation from our causal retrospect, which opens promising directions for image captioning.
更多查看译文
关键词
Training, Correlation, Toy manufacturing industry, Visualization, Task analysis, Shape, Magnetic heads, Image captioning, causality, deconfounding, the backdoor adjustment, the front-door adjustment
AI 理解论文
溯源树
样例
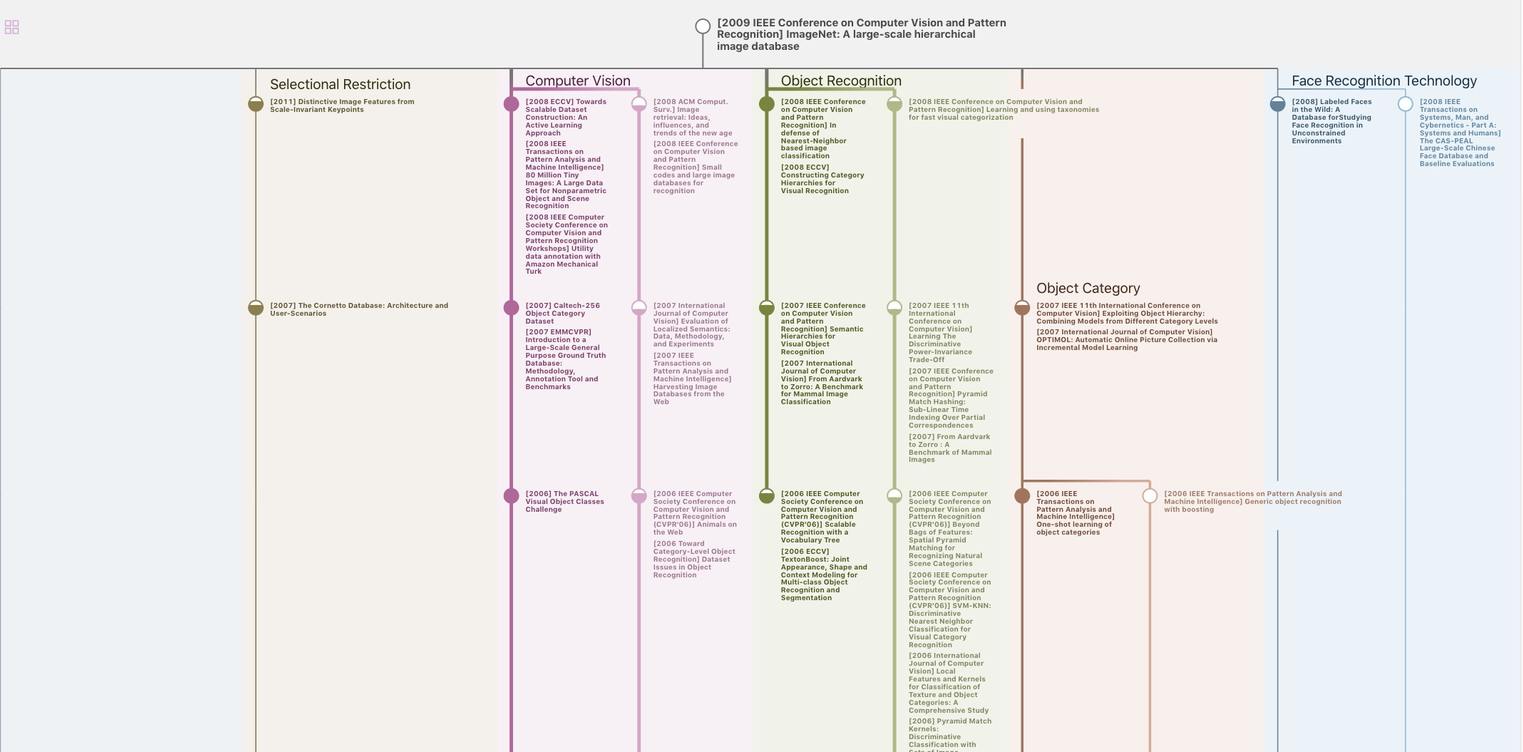
生成溯源树,研究论文发展脉络
Chat Paper
正在生成论文摘要