Risk Analysis of Divide-and-Conquer ERM
arxiv(2020)
摘要
Theoretical analysis of the divide-and-conquer based distributed learning with least square loss in the reproducing kernel Hilbert space (RKHS) have recently been explored within the framework of learning theory. However, the studies on learning theory for general loss functions and hypothesis spaces remain limited. To fill the gap, we study the risk performance of distributed empirical risk minimization (ERM) for general loss functions and hypothesis spaces. The main contributions are two-fold. First, we derive two risk bounds of optimal rates under certain basic assumptions on the hypothesis space, as well as the smoothness, Lipschitz continuity, strong convexity of the loss function. Second, we further develop two more general risk bounds for distributed ERM without the restriction of strong convexity
更多查看译文
关键词
risk,divide-and-conquer
AI 理解论文
溯源树
样例
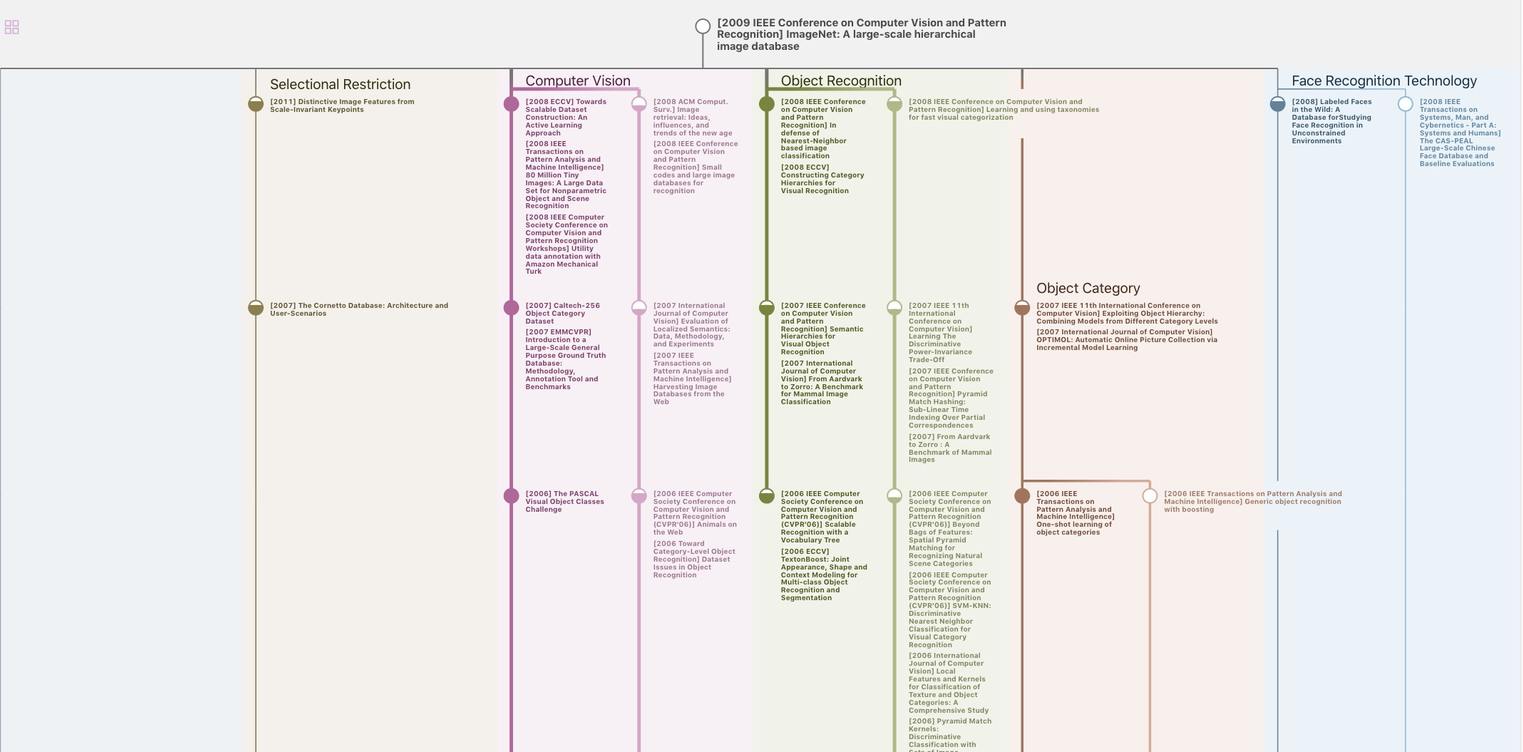
生成溯源树,研究论文发展脉络
Chat Paper
正在生成论文摘要