DeepSemanticHPPC: Hypothesis-based Planning over Uncertain Semantic Point Clouds
ICRA(2020)
摘要
Planning in unstructured environments is challenging -- it relies on sensing, perception, scene reconstruction, and reasoning about various uncertainties. We propose DeepSemanticHPPC, a novel uncertainty-aware hypothesis-based planner for unstructured environments. Our algorithmic pipeline consists of: a deep Bayesian neural network which segments surfaces with uncertainty estimates; a flexible point cloud scene representation; a next-best-view planner which minimizes the uncertainty of scene semantics using sparse visual measurements; and a hypothesis-based path planner that proposes multiple kinematically feasible paths with evolving safety confidences given next-best-view measurements. Our pipeline iteratively decreases semantic uncertainty along planned paths, filtering out unsafe paths with high confidence. We show that our framework plans safe paths in real-world environments where existing path planners typically fail.
更多查看译文
关键词
DeepSemanticHPPC,hypothesis-based planning,uncertain semantic point clouds,deep Bayesian neural network,flexible point cloud scene representation,sparse visual measurements,hypothesis-based path planner,uncertainty-aware hypothesis-based planner
AI 理解论文
溯源树
样例
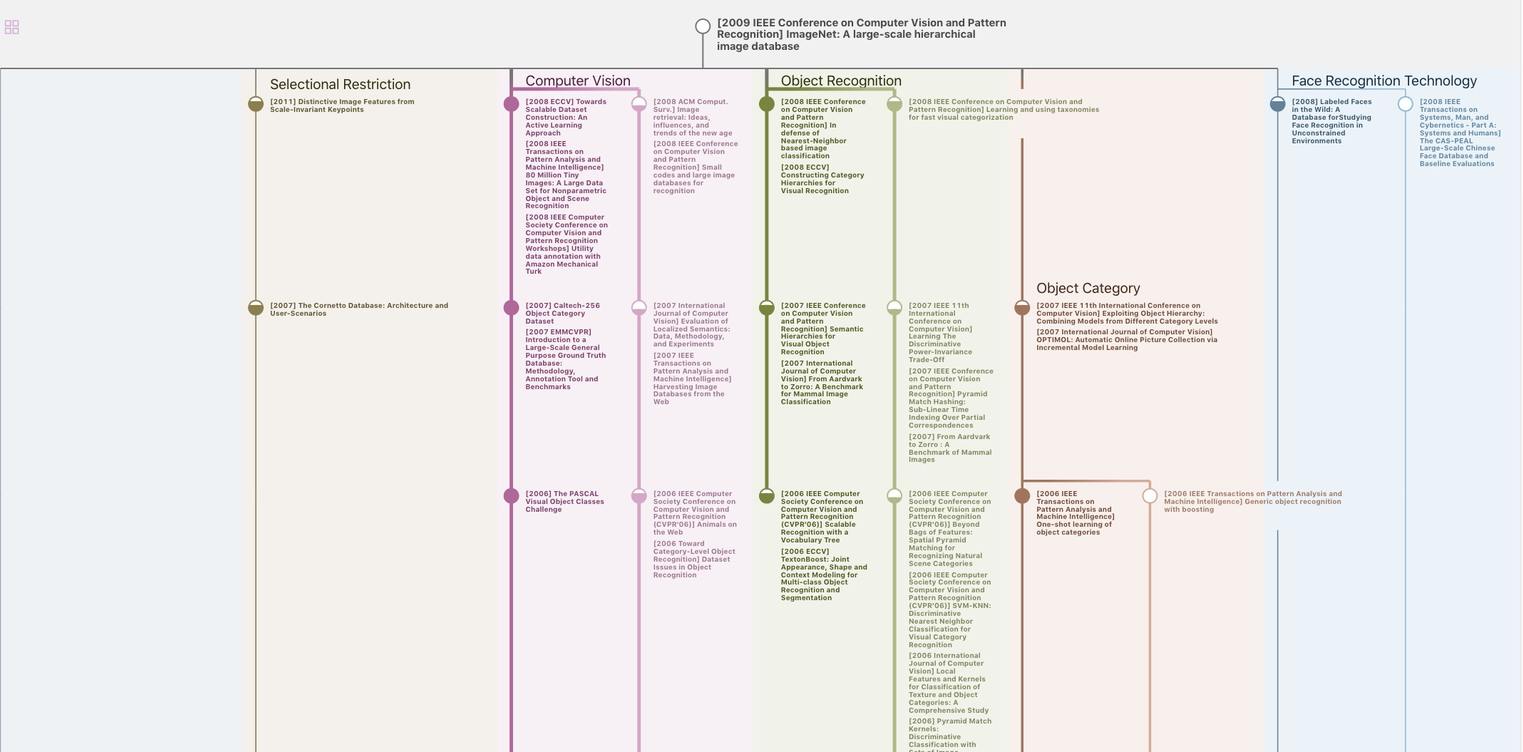
生成溯源树,研究论文发展脉络
Chat Paper
正在生成论文摘要