A Latent-Label Denoising Method For Relation Extraction With Self-Directed Confidence Learning
INTELLIGENT DATA ANALYSIS(2020)
摘要
Distant supervision for relation extraction aims to automatically obtain a large number of relational facts as training data, but it often leads to noisy label problem. In this paper, we propose a self-directed confidence learning based latent-label denoising method for distantly supervised relation extraction. Concretely, a self-directed algorithm that combines the semantic information of model prediction and distant supervision is designed to predict the confidence score of latent labels. Since this mechanism utilizes the obtained latent labels of easy examples to produce the latent labels of hard examples step by step, it is a robust and reliable learning process. Besides, it facilitates dynamic exploration of the confidence space to achieve better denoising performance. Moreover, to cope with the common imbalance problem in large corpus where the negative instances account for a much larger percentage, we introduce a discriminative loss function to solve the misclassification between non-relational and relational instances. Empirically, in order to verify the generality of the proposed denoising method, we use different neural models - CNN, PCNN and BiLSTM for representation learning. Experimental results show that our method can correct the noisy labels with high accuracy and outperform the state-of-the-art relation extraction systems.
更多查看译文
关键词
Distant supervision, relation extraction, latent label, confidence learning, discriminative loss
AI 理解论文
溯源树
样例
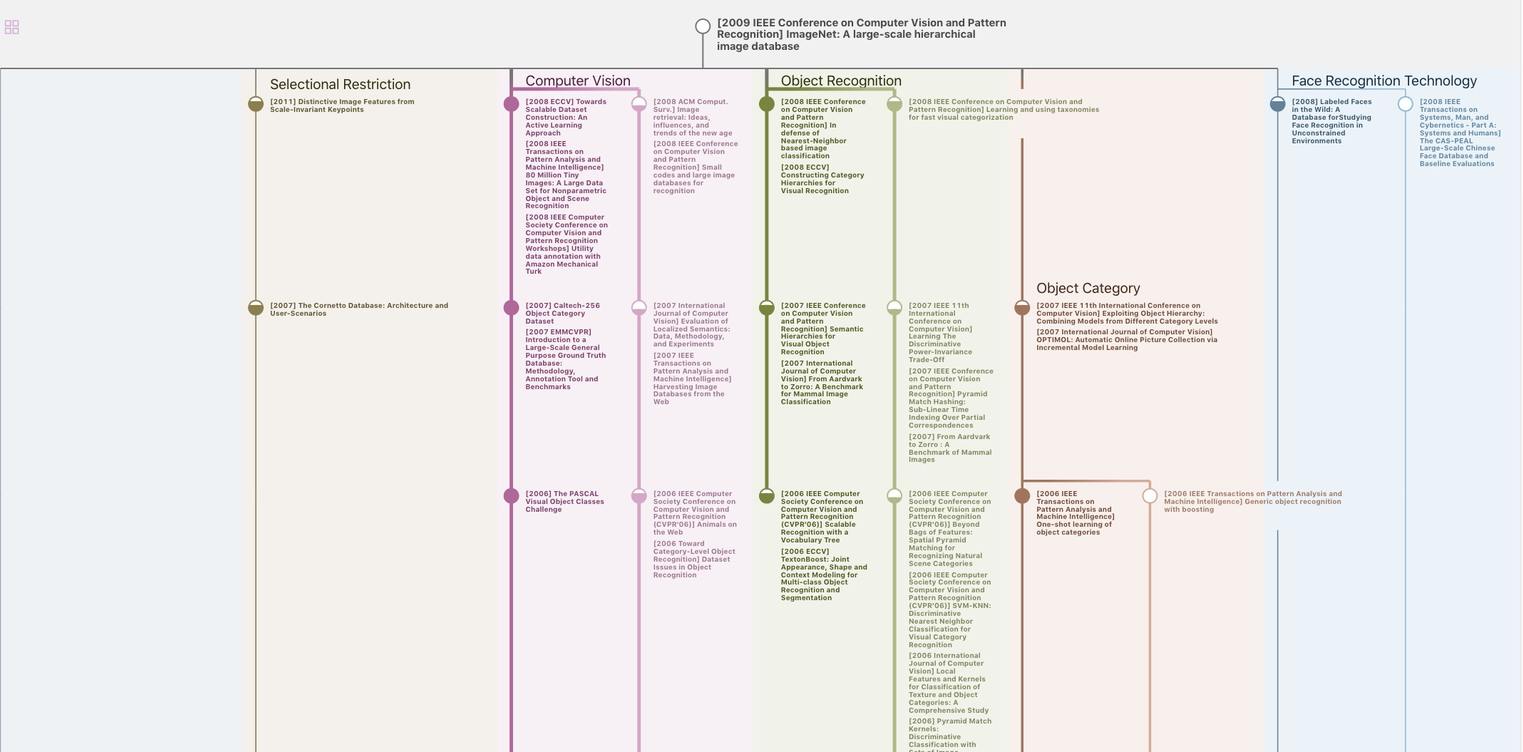
生成溯源树,研究论文发展脉络
Chat Paper
正在生成论文摘要