Label Distribution Learning With Climate Probability For Ensemble Forecasting
INTELLIGENT DATA ANALYSIS(2020)
摘要
In meteorology, ensemble forecasting aims to post-process an ensemble of multiple members' forecasts and make better weather predictions. While multiple individual forecasts are generated to represent the uncertain weather system, the performance of ensemble forecasting is unsatisfactory. In this paper we conduct data analysis based on the expertise of human forecasters and introduce a machine learning method for ensemble forecasting. The proposed method, Label Distribution Learning with Climate Probability (LDLCP), can improve the accuracy of both deterministic forecasting and probabilistic forecasting. The LDLCP method utilizes the relevant variables of previous forecasts to construct the feature matrix and applies label distribution learning (LDL) to adjust the probability distribution of ensemble forecast. Our proposal is novel in its specialized target function and appropriate conditional probability function for the ensemble forecasting task, which can optimize the forecasts to be consistent with local climate. Experimental testing is performed on both artificial data and the data set for ensemble forecasting of precipitation in East China from August to November, 2017. Experimental results show that, compared with a baseline method and two state-of-the-art machine learning methods, LDLCP shows significantly better performance on measures of RMSE and average continuous ranked probability score.
更多查看译文
关键词
Ensemble forecasting, label distribution learning, post-processing, domain knowledge
AI 理解论文
溯源树
样例
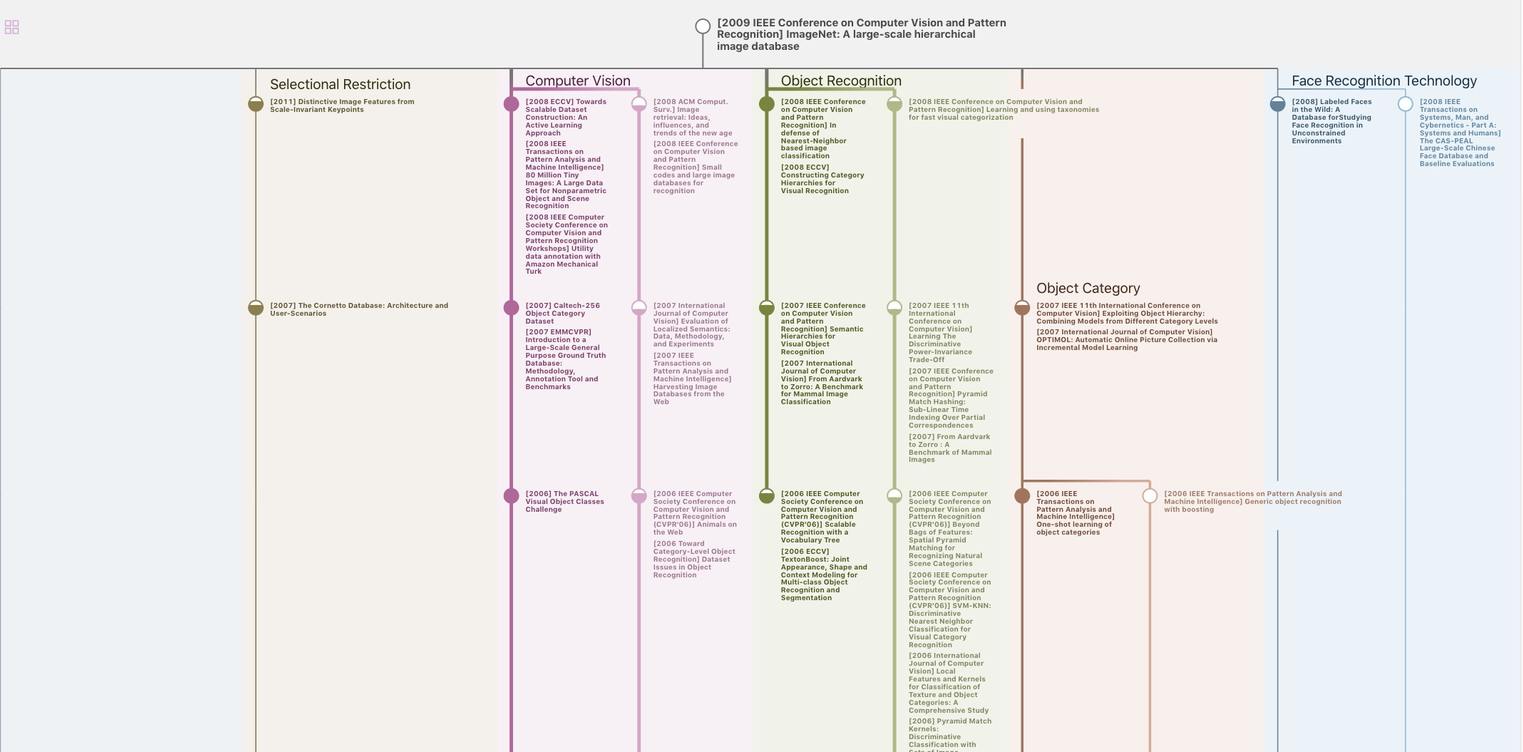
生成溯源树,研究论文发展脉络
Chat Paper
正在生成论文摘要