Redundant Sniffer Deployment for Multi-Channel Wireless Network Forensics With Unreliable Conditions
IEEE Transactions on Cognitive Communications and Networking(2020)
摘要
Network forensics refers to monitoring and analysis of network traffic for the purpose of information gathering, legal evidence, or intrusion detection. Wireless sniffers are usually deployed to collect PHY/MAC-layer information to trace abnormal wireless traffic. For multi-channel wireless networks, it becomes problematic to allocate each sniffer an appropriate monitoring channel due to the limited number of sniffers. This leads to the sniffer-channel assignment (SCA) problem that has been mostly studied assuming error-free channel conditions or known behavior of wireless users. In this paper, we study the SCA problem with more general settings. In particular, we introduce redundant sniffer deployment to combat against the
unreliable channel conditions
. This can be formulated as a non-linear integer program with the aim of maximizing the number of captured data packets. We propose both centralized and distributed algorithms to determine an optimal strategy. For
unknown user behaviors
, we formulate the redundant SCA problem as a multi-armed bandit problem and develop an online learning policy to find a balance between the exploitation, i.e., accuracy, and exploration, i.e., coverage, in channel monitoring. Simulation results reveal that the redundant sniffer deployment, though sacrificing the exploration opportunities in the learning process, is robust against the uncertainty of user activities and provides the optimal performance in terms of sensing accuracy and monitoring coverage.
更多查看译文
关键词
Passive monitoring,redundant sniffer deployment,monotonic optimization,multi-armed bandit
AI 理解论文
溯源树
样例
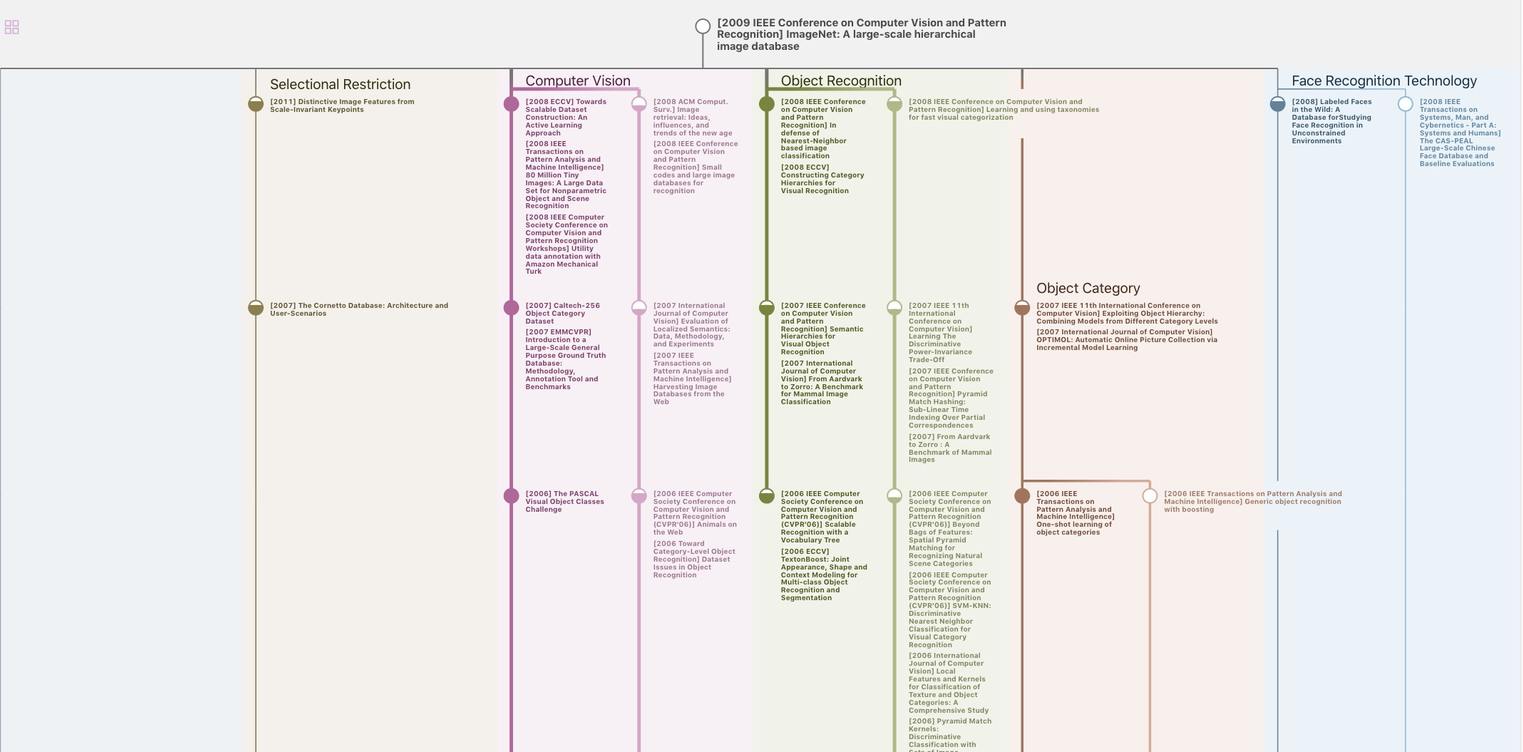
生成溯源树,研究论文发展脉络
Chat Paper
正在生成论文摘要