Learning A Mixture Of Granularity-Specific Experts For Fine-Grained Categorization
2019 IEEE/CVF INTERNATIONAL CONFERENCE ON COMPUTER VISION (ICCV 2019)(2019)
摘要
We aim to divide the problem space of fine-grained recognition into some specific regions. To achieve this, we develop a unified framework based on a mixture of experts. Due to limited data available for the fine-grained recognition problem, it is not feasible to learn diverse experts by using a data division strategy. To tackle the problem, we promote diversity among experts by combing an expert gradually-enhanced learning strategy and a Kullback-Leibler divergence based constraint. The strategy learns new experts on the dataset with the prior knowledge from former experts and adds them to the model sequentially, while the introduced constraint forces the experts to produce diverse prediction distribution. These drive the experts to learn the task from different aspects, making them specialized in different subspace problems. Experiments show that the resulting model improves the classification performance and achieves the state-of-the-art performance on several fine-grained benchmark datasets.
更多查看译文
关键词
granularity-specific experts,fine-grained categorization,fine-grained recognition problem,data division strategy,Kullback-Leibler divergence based constraint,fine-grained benchmark datasets,gradually-enhanced learning strategy
AI 理解论文
溯源树
样例
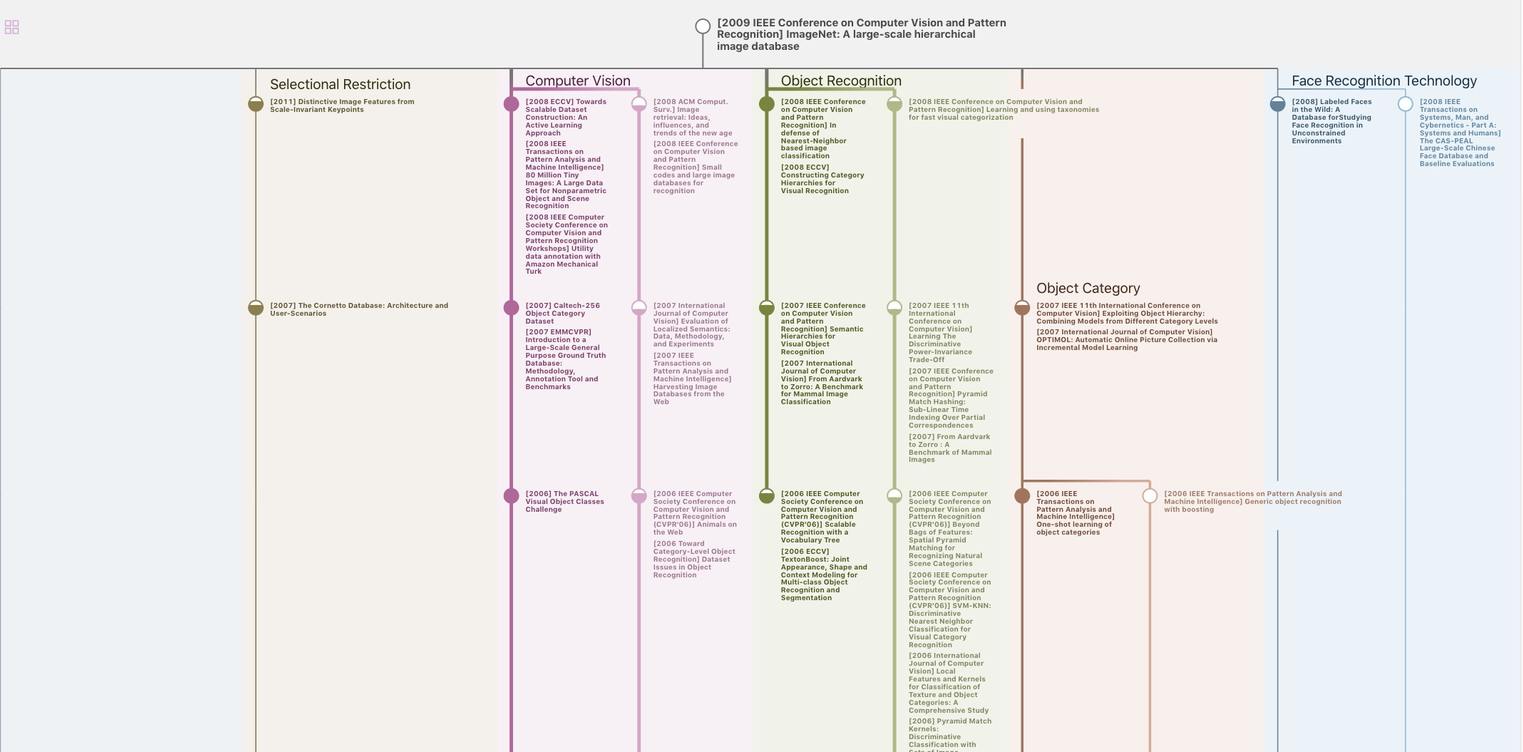
生成溯源树,研究论文发展脉络
Chat Paper
正在生成论文摘要